Blood Pressure Morphology as a Fingerprint of Cardiovascular Health: A Machine Learning Based Approach
APPLIED INFORMATICS (ICAI 2021)(2021)
摘要
Daily exercise and a healthful diet allows the cardiovascular (CV) system to age slower. However, unhealthy life habits and diseases impact negatively on this condition. In consequence, an individual's "chronological age" (CHA) may differ from the "arterial age" (AA). There is proven evidence of correlation between arterial stiffness (AS), highly associated with AA, and central blood pressure (BP) waveform, which combined with other CV properties, define a group of characteristic parameters to assess CV Health (CVH). Such assessment, obtained by means of noninvasive and simple arterial measurements is extremely useful for the prevention of CV diseases. Machine Learning (ML) techniques allow the development of predicting models, but usually a large amount of data is required, often unavailable and/or not fully complete. One-dimensional (1D) CV models become an interesting alternative to overcome this issue, since CV system simulations can be performed. OBJECTIVE: A ML model was trained/validated with data from healthy and heterogeneous population (n = 4374), using parameters derived from arterial pressure waveforms (APW) in order to assess CVH. METHODS: Sixteen features were extracted from carotid and radial APW. Such were used for prediction of cardiac output (CO), systemic vascular resistance (SVR), systolic BP (SBPc), diastolic BP (DBPc), carotid-femoral pulse wave velocity (PWVcf), aortic augmentation index (Ala) and CHA as a surrogate of AA. RESULTS: The normalized-RMSEs for CO, SVR, SBPc, DBPc, PWVcf, Ala and CHA using the best-performing model were 5.4%, 2.2%, 0.1%, 0.06%, 0.6%, 1.2% and 5.5%, respectively. CONCLUSION: The acquired results showed that the ML models obtained from measurements in the assessed locations provide an acceptable performance on estimating CVH parameters. Further in-vivo studies are required to validate the application of the outcome.
更多查看译文
关键词
One-dimensional modelling, Pulse wave analysis, Cardiovascular health, Machine learning, Risk prediction
AI 理解论文
溯源树
样例
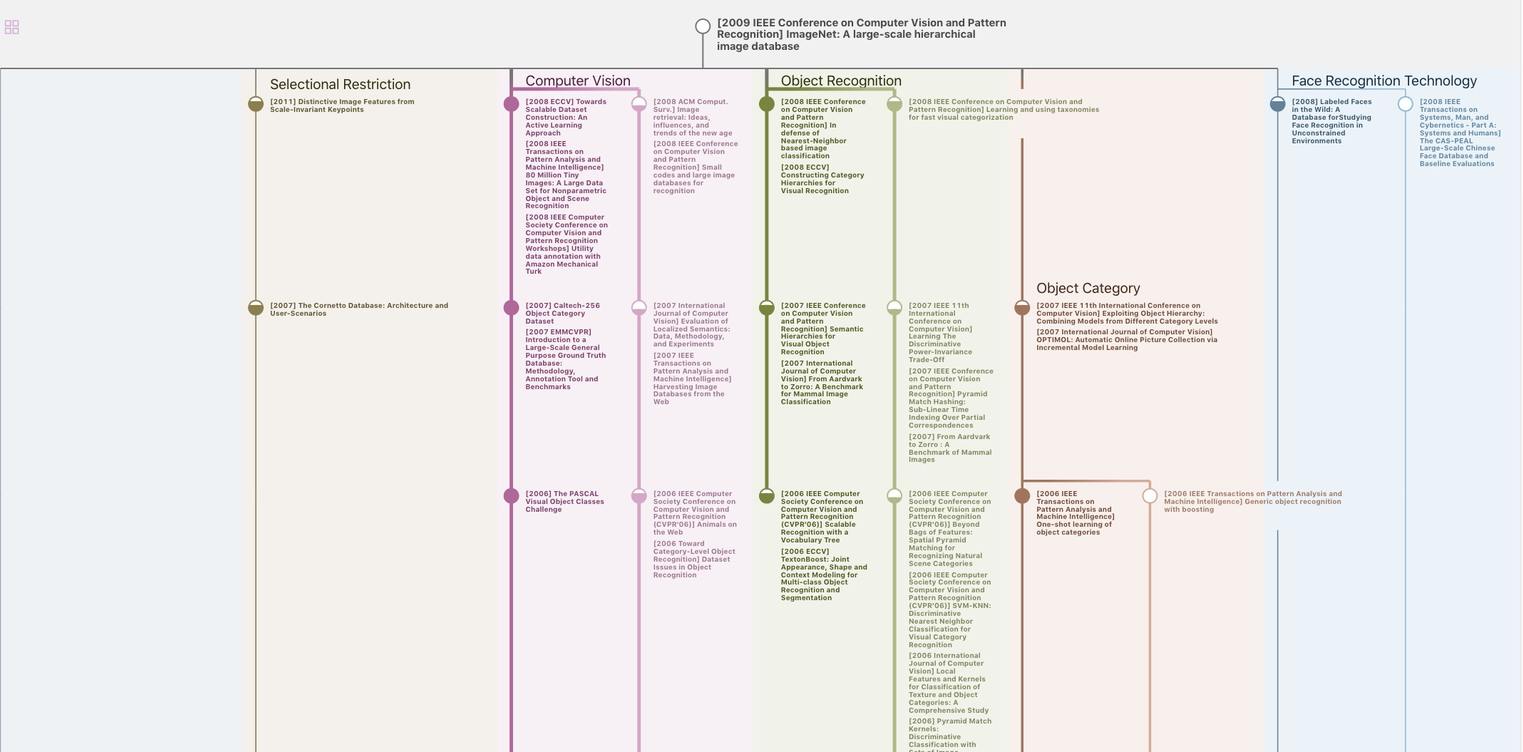
生成溯源树,研究论文发展脉络
Chat Paper
正在生成论文摘要