An Optimized Technique for RNA Prediction Based on Neural Network
INTELLIGENT AUTOMATION AND SOFT COMPUTING(2023)
摘要
Pathway reconstruction, which remains a primary goal for many inves-tigations, requires accurate inference of gene interactions and causality. Non -cod-ing RNA (ncRNA) is studied because it has a significant regulatory role in many plant and animal life activities, but interacting micro-RNA (miRNA) and long non-coding RNA (lncRNA) are more important. Their interactions not only aid in the in-depth research of genes' biological roles, but also bring new ideas for illness detection and therapy, as well as plant genetic breeding. Biological inves-tigations and classical machine learning methods are now used to predict miRNA-lncRNA interactions. Because biological identification is expensive and time-con-suming, machine learning requires too much manual intervention, and the feature extraction process is difficult. This research presents a deep learning model that combines the advantages of convolutional neural networks (CNN) and bidirec-tional long short-term memory networks (Bi-LSTM). It not only takes into account the connection of information between sequences and incorporates con-textual data, but it also thoroughly extracts the sequence data's features. On the corn data set, cross-checking is used to evaluate the model's performance, and it is compared to classical machine learning. To acquire a superior classification effect, the proposed strategy was compared to a single model. Additionally, the potato and wheat data sets were utilized to evaluate the model, with accuracy rates of 95% and 93%, respectively, indicating that the model had strong generalization capacity.
更多查看译文
关键词
Neural networks, biomedical engineering, genetic analysis, machine learning
AI 理解论文
溯源树
样例
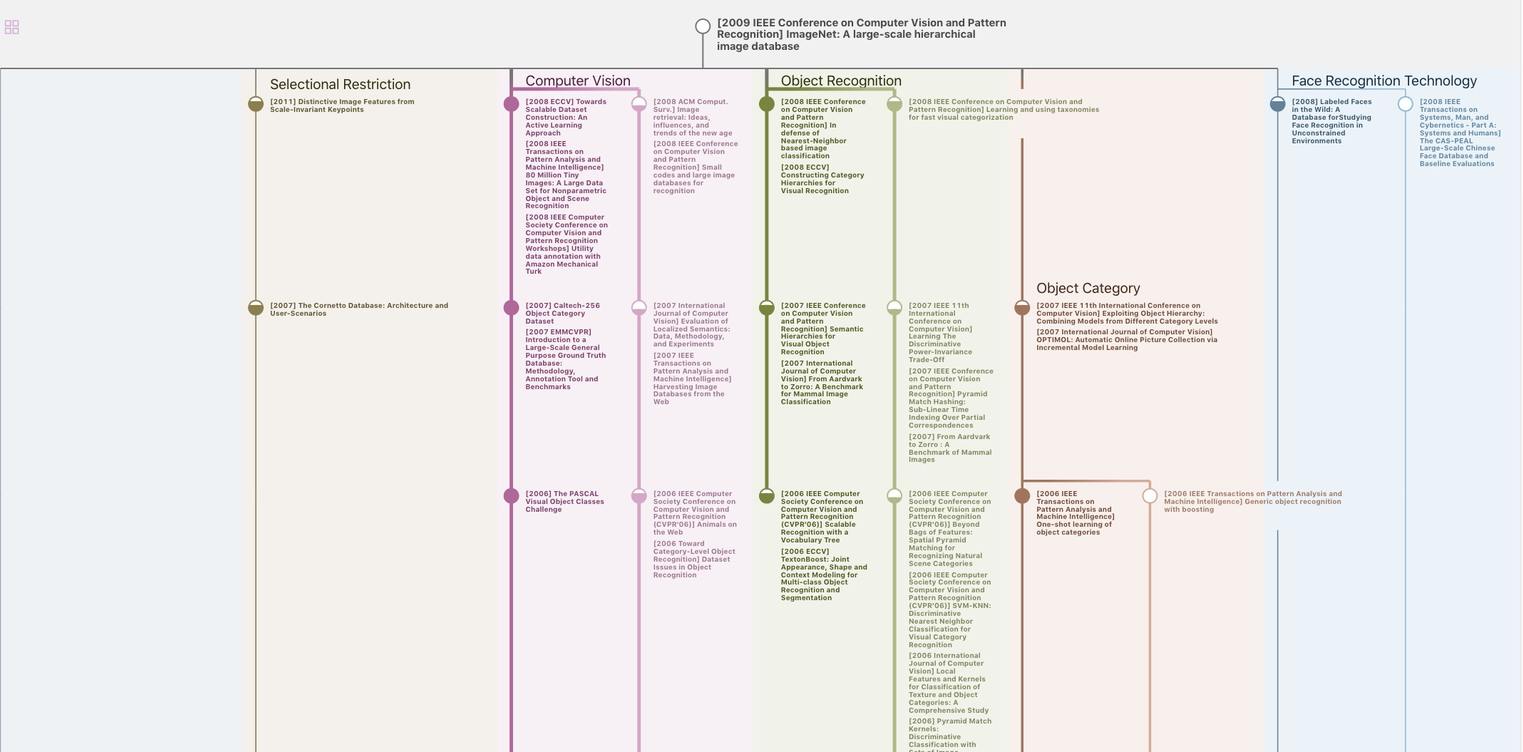
生成溯源树,研究论文发展脉络
Chat Paper
正在生成论文摘要