Validation of a Hybrid Active Shape and Deep Learning Intracochlear Anatomy Segmentation Method for Image-guided Cochlear Implant Programming
MEDICAL IMAGING 2021: IMAGE-GUIDED PROCEDURES, ROBOTIC INTERVENTIONS, AND MODELING(2021)
摘要
Cochlear implants (CIs) are neuroprosthetic devices that can improve hearing in patients with severe-to-profound hearing loss. Postoperatively, a CI device needs to be programmed by an audiologist to determine parameter settings that lead to the best outcomes. Our group has developed an image-guided cochlear implant programming (IGCIP) system to simplify this laborious post- programming procedure and improve hearing outcomes. IGCIP requires image processing techniques to analyze the location of the inserted electrode arrays (EAs) with respect to the intracochlear anatomy (ICA). An active shape model (ASM)-based method is currently in routine use in our IGCIP system for ICA segmentation. Recently, we have proposed a hybrid ASM/deep learning (DL) segmentation method that improves segmentation accuracy. In this work, we first evaluate the effect of this method on so-called distance-vs.-frequency curves (DVFs), which permit to visualize electrode interaction and are used to provide programming guidance. An expert evaluation study is then performed to manually configure the electrodes based on the DVFs and grade the quality of the electrode configurations derived from ASM and hybrid ASM/DL segmentations compared to the one derived from ground truth segmentation. Results we have obtained show that the hybrid ASM/DL segmentation technique tends to generate DVFs with smaller frequency error and distance error, and electrode configurations which are comparable to the existing ASM-based method.
更多查看译文
关键词
Cochlear implant,image segmentation,image-guided cochlear implant programming
AI 理解论文
溯源树
样例
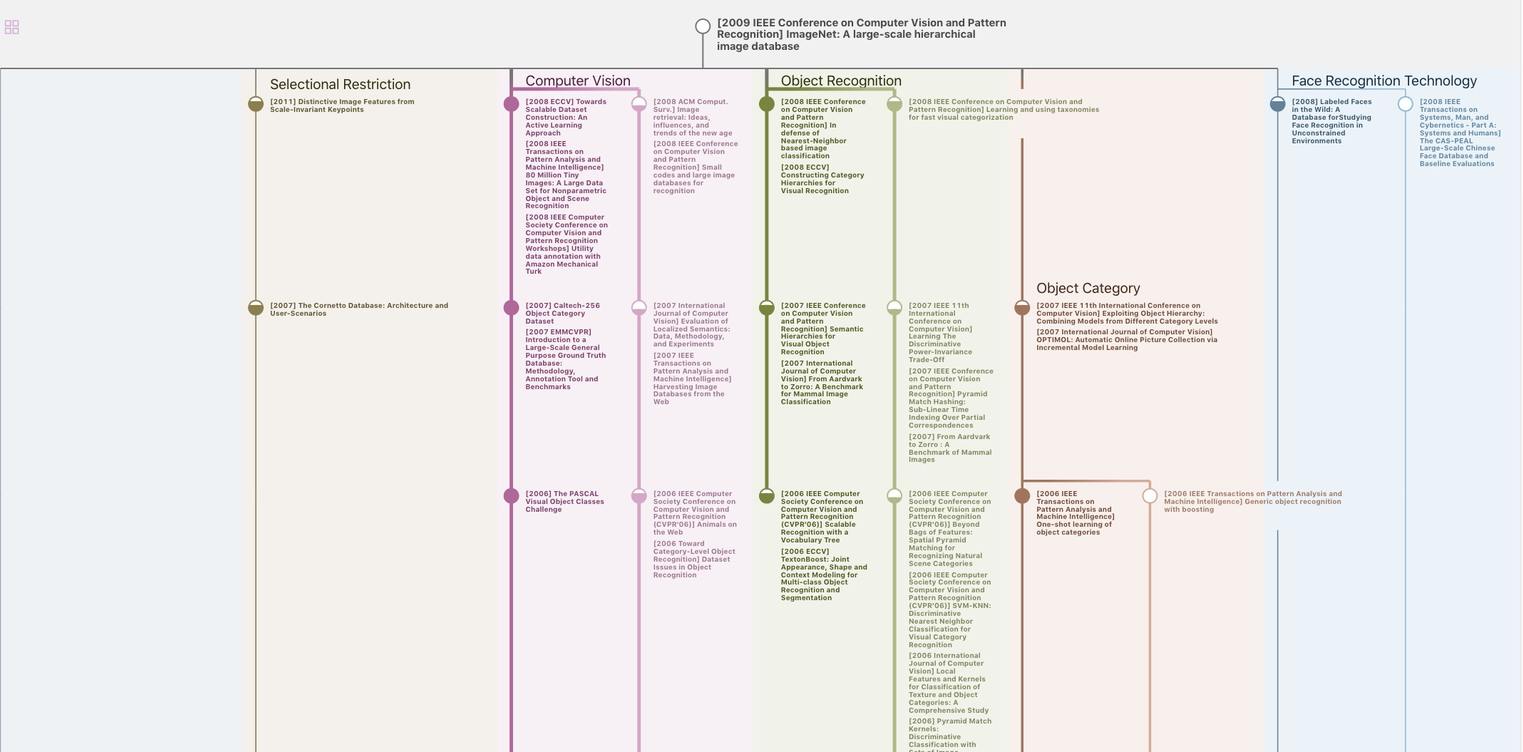
生成溯源树,研究论文发展脉络
Chat Paper
正在生成论文摘要