On the estimation of partially observed continuous-time Markov chains
COMPUTATIONAL STATISTICS(2022)
Abstract
Motivated by the increasing use of discrete-state Markov processes across applied disciplines, a Metropolis–Hastings sampling algorithm is proposed for a partially observed process. Current approaches, both classical and Bayesian, have relied on imputing the missing parts of the process and working with a complete likelihood. However, from a Bayesian perspective, the use of latent variables is not necessary and exploiting the observed likelihood function, combined with a suitable Markov chain Monte Carlo method, results in an accurate and efficient approach. A comprehensive comparison with simulated and real data sets demonstrate our approach when compared with alternatives available in the literature.
MoreTranslated text
Key words
Bayesian estimation,Transition matrix,Credit risk scoring
AI Read Science
Must-Reading Tree
Example
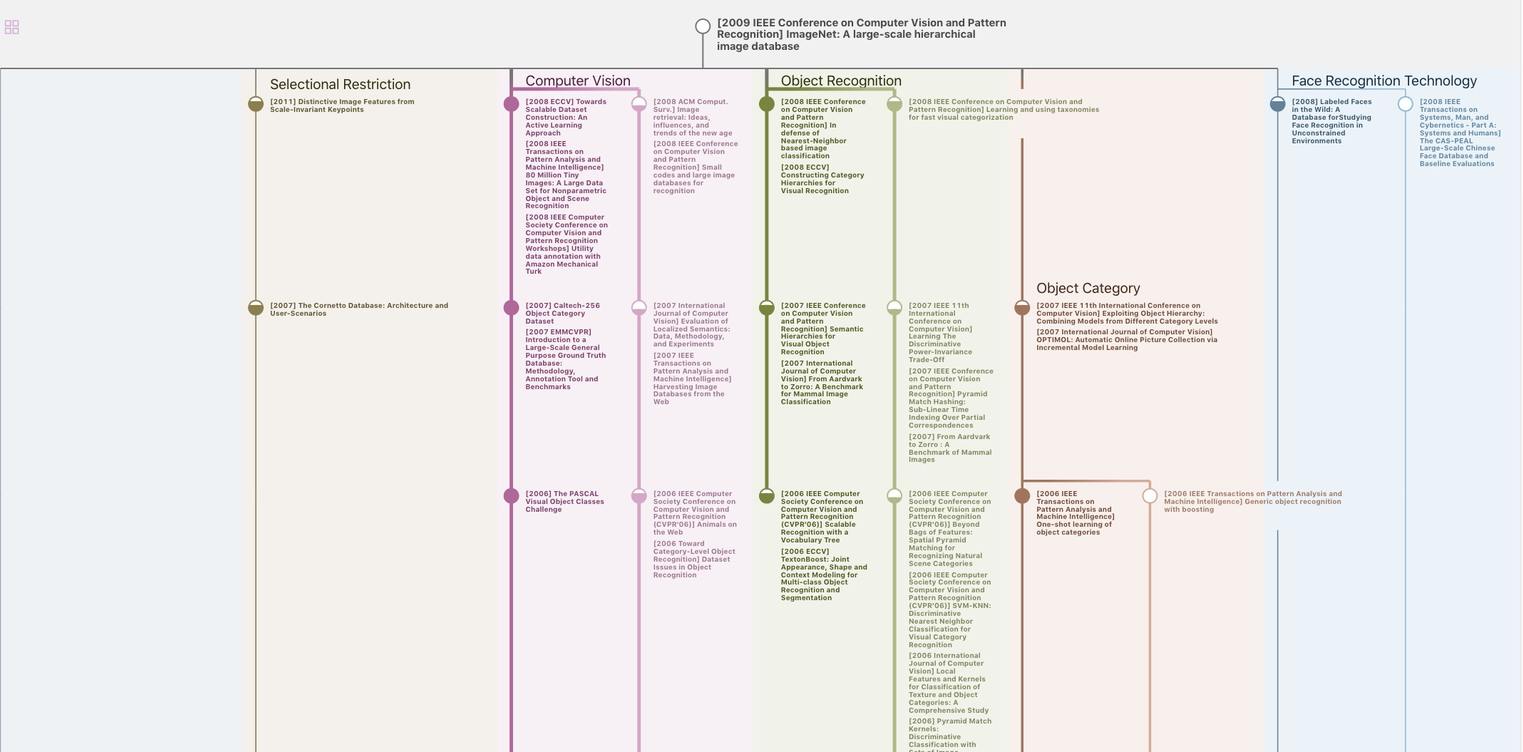
Generate MRT to find the research sequence of this paper
Chat Paper
Summary is being generated by the instructions you defined