Intelligent pixel-level detection of multiple distresses and surface design features on asphalt pavements
COMPUTER-AIDED CIVIL AND INFRASTRUCTURE ENGINEERING(2022)
摘要
Simultaneous pixel-level detection of multiple distresses and surface design features on complex asphalt pavements is a critical challenge in intelligent pavement survey. This paper proposes a deep-learning model named ShuttleNet to provide an efficient solution for this challenge by implementing robust semantic segmentation on asphalt pavements. The proposed ShuttleNet aims at repeating the encoding-decoding round freely or even endlessly such that the contexts at different resolution levels can be learned and integrated many times for enhanced latent representations. Additionally, a new and efficient connection method called memory connection is also proposed in the paper and deployed in the ShuttleNet model to provide shortcut connections between successive encoding-decoding rounds. The proposed memory connection can partially or entirely carry the decoded information at different resolution levels into the next encoding-decoding round. Pairing 3D pavement images with 2D pavement images, the proposed ShuttleNet model is applied to detect multiple distresses and surface design features on asphalt pavements simultaneously, including pavement cracks, potholes, sealed cracks, patches, markings, expansion joints, and the pavement background. Experimental results demonstrate that the mean F-measure and mean intersection-over-union attained by the recommended architectural variation of the proposed ShuttleNet model on 1500 testing image pairs are 92.54% and 0.8657 respectively. According to the performance comparisons using both private and public datasets, the proposed ShuttleNet model can yield a noticeably higher detection accuracy, compared with four state-of-the-art models for semantic segmentation.
更多查看译文
AI 理解论文
溯源树
样例
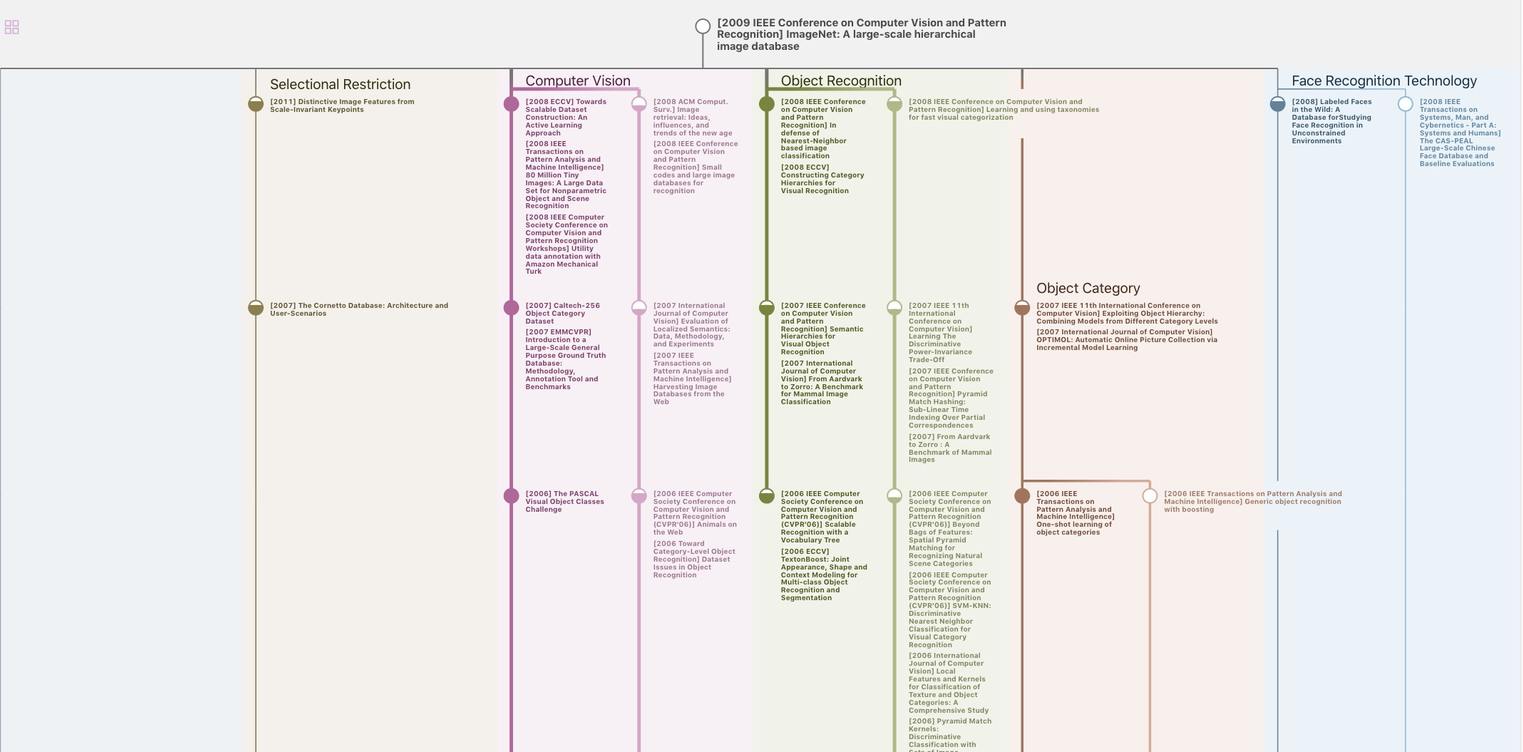
生成溯源树,研究论文发展脉络
Chat Paper
正在生成论文摘要