VOC-Net: A Deep Learning Model for the Automated Classification of Rotational THz Spectra of Volatile Organic Compounds
APPLIED SCIENCES-BASEL(2022)
摘要
Conventional black box machine learning (ML) algorithms for gas-phase species identification from THz frequency region absorption spectra have been reported in the literature. While the robust classification performance of such ML models is promising, the black box nature of these ML tools limits their interpretability and acceptance in application. Here, a one-dimensional convolutional neural network (CNN), VOC-Net, is developed and demonstrated for the classification of absorption spectra for volatile organic compounds (VOCs) in the THz frequency range, specifically from 220 to 330 GHz where prior experimental data is available. VOC-Net is trained and validated against simulated spectra, and also demonstrated and tested against experimental spectra. The performance of VOC-Net is examined by the consideration of confusion matrices and receiver-operator-characteristic (ROC) curves. The model is shown to be 99+% accurate for the classification of simulated spectra and 97% accurate for the classification of noisy experimental spectra. The model's internal logic is examined using the Gradient-weighted Class Activation Mapping (Grad-CAM) method, which provides a visual and interpretable explanation of the model's decision making process with respect to the important distinguishing spectral features.
更多查看译文
关键词
VOC, DNN, CNN, classification, THz, spectroscopy, rotational, microelectronic, spectrometer, gas sensing
AI 理解论文
溯源树
样例
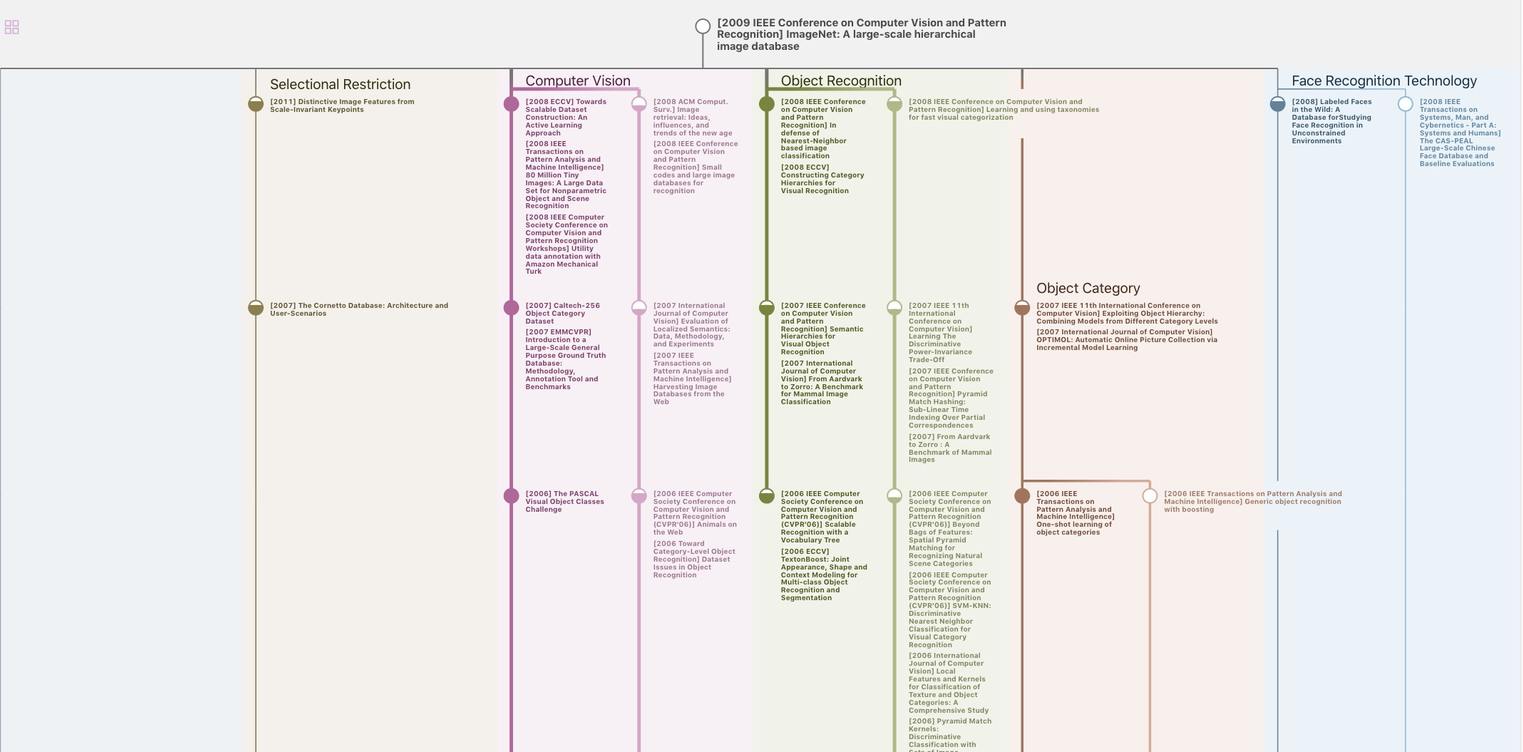
生成溯源树,研究论文发展脉络
Chat Paper
正在生成论文摘要