Real-Time Hysteresis Foreground Detection in Video Captured by Moving Cameras
2022 IEEE International Conference on Imaging Systems and Techniques (IST)(2022)
摘要
Foreground detection is an important first step in video analytics. While the stationary cameras facilitate the foreground detection due to the apparent motion between the moving foreground and the still background, the moving cameras make such a task more challenging because both the foreground and the background appear in motion in the video. To tackle this challenging problem, an innovative real-time foreground detection method is presented, that models the foreground and the background simultaneously and works for both moving and stationary cameras. In particular, first, each input video frame is partitioned into a number of blocks. Then, assuming the background takes the majority of each video frame, the iterative pyramidal implementation of the Lucas-Kanade optical flow approach is applied on the centers of the background blocks in order to estimate the global motion and compensate for the camera movements. Subsequently, each block in the background is modeled by a mixture of Gaussian distributions and a separate Gaussian mixture model is constructed for the foreground in order to enhance the classification. However, the errors in motion compensation can contaminate the foreground model with background values. The novel idea of the proposed method matches a set of background samples to their corresponding block for the most recent frames in order to avoid contaminating the foreground model with background samples. The input values that do not fit into either the statistical or the sample-based background models are used to update the foreground model. Finally, the foreground is detected by applying the Bayes classification technique to the major components in the background and foreground models, which removes the false positives caused by the hysteresis effect. Experimental evaluations demonstrate the feasibility of the proposed method in the foreground segmentation when applied to videos in public datasets.
更多查看译文
关键词
stationary cameras,video frame,background blocks,camera movements,Gaussian mixture model,background values,background samples,foreground segmentation,moving cameras,video analytics,moving foreground,real-time hysteresis foreground detection,sample-based background,still background,iterative pyramidal implementation,Lucas-Kanade optical flow,global motion estimation,Gaussian distributions,foreground classification,motion compensation,Bayes classification,hysteresis effect
AI 理解论文
溯源树
样例
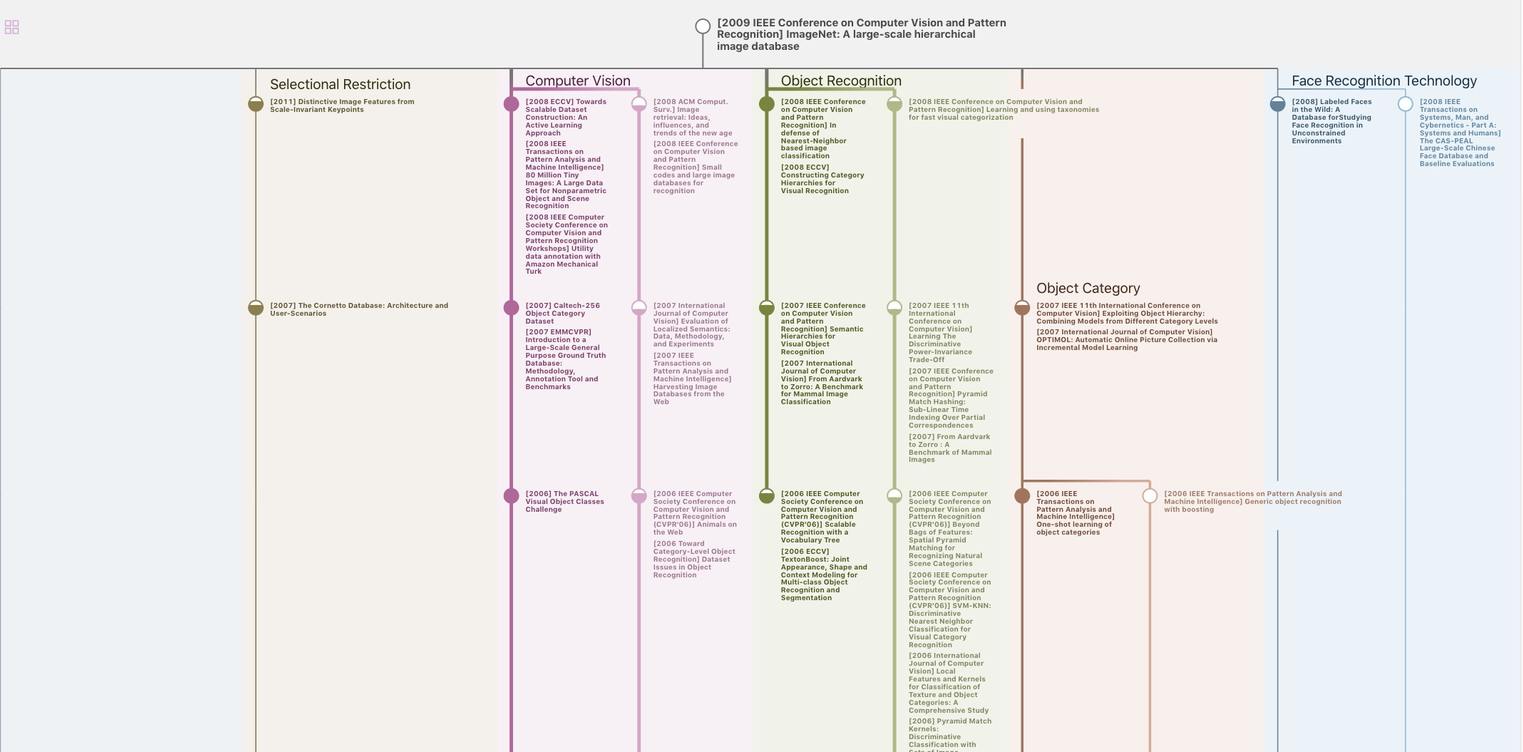
生成溯源树,研究论文发展脉络
Chat Paper
正在生成论文摘要