A Comprehensive Overview on Modified Versions of Stockwell Transform For Power Quality Monitoring
IEEE ACCESS(2022)
摘要
The increasing trends toward the accurate identification of power quality disturbances (PQD) via power quality (PQ) monitoring require an appropriate digital signal processing (DSP) technique and a robust classifier. To this end, Stockwell transform (ST), one of the most efficient feature extraction DSP tools, and its several variants play an utmost role in PQ assessment framework. Its time-varying spectral characteristics generally extract the local instantaneous frequency spectrum from the global temporary behavior of PQD signal. However, the Standard ST suffers from the poor time-frequency resolution because of its frequency-dependent Gaussian window (GW). While the analysis of the statistically time-varying signals requires a suitable balance between time and frequency resolution. To this end, this paper provides a comprehensive literature review on several modified versions of Standard ST for the first time to reduce the computational complexity of the algorithm as well as maximize the energy concentration of the time-frequency plane. A comparative analysis of all the modified STs has been presented in tabular form to provide the key characteristics of each technique. Additionally, a case study has been presented to substantiate the highest accuracy of the proposed algorithm over the other ST variants. Apart from the PQD classification, miscellaneous applications of Standard ST and its modified variants have been indicated. This review paper may provide a valuable resource to the researchers for further improvement of the time-frequency resolution of ST not only in classifying PQD but also for its other wide applications.
更多查看译文
关键词
Time-frequency analysis, Standards, Transforms, Feature extraction, Continuous wavelet transforms, Signal resolution, Power quality, Energy consumption, Energy concentration, power quality monitoring, power quality disturbance, Stockwell transform, time-frequency analysis
AI 理解论文
溯源树
样例
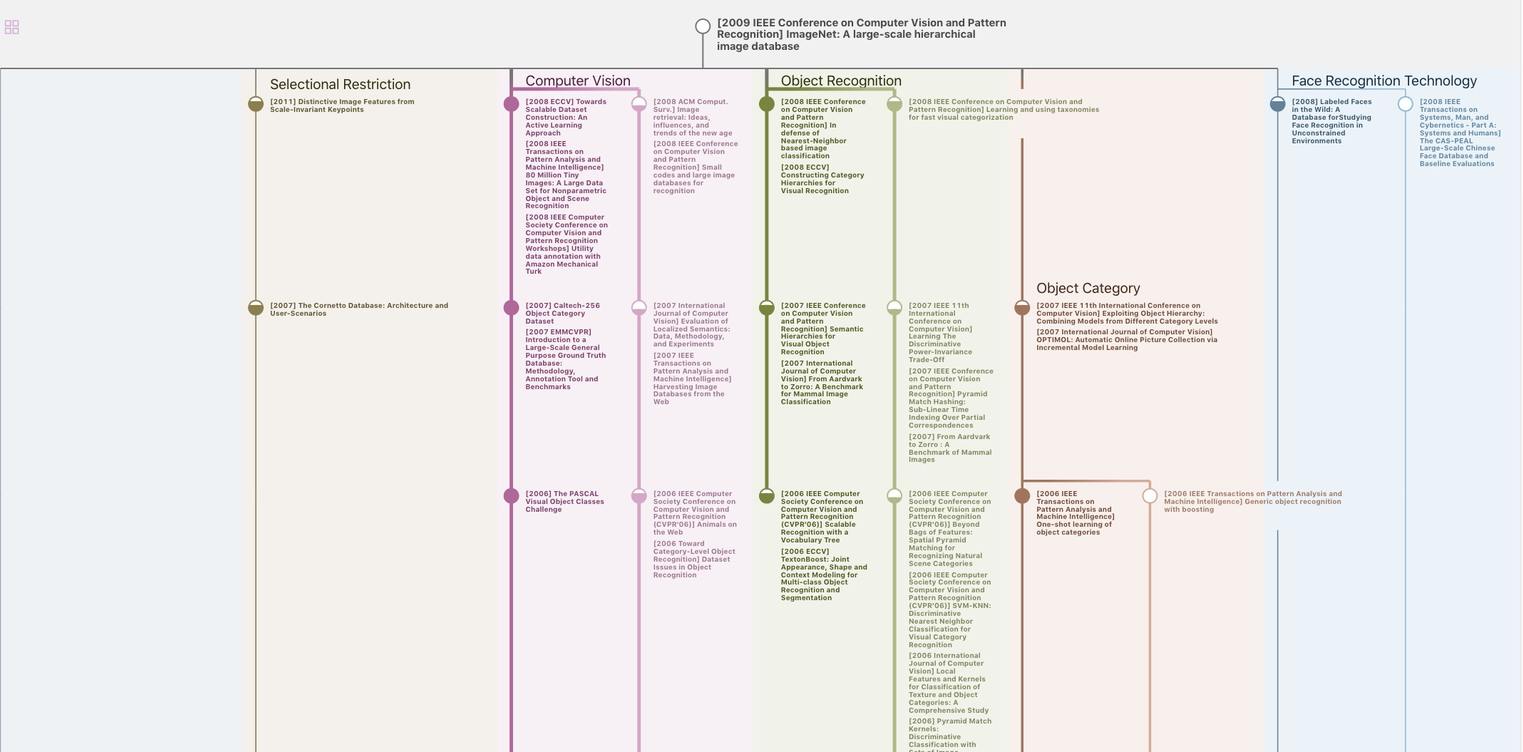
生成溯源树,研究论文发展脉络
Chat Paper
正在生成论文摘要