Rank-GCN for Robust Action Recognition
IEEE ACCESS(2022)
摘要
We present a robust skeleton-based action recognition method with graph convolutional network (GCN) that uses the new adjacency matrix, called Rank-GCN. In Rank-GCN, the biggest change from previous approaches is how the adjacency matrix is generated to accumulate features from neighboring nodes by re-defining "adjacency." The new adjacency matrix, which we call the rank adjacency matrix, is generated by ranking all the nodes according to metrics including the Euclidean distance from the nodes of interest, whereas the previous GCNs methods used only 1-hop neighboring nodes to construct adjacency. By adopting the rank adjacency matrix, we find not only performance improvements but also robustness against swapping, location shifting and dropping of certain nodes. The fact that the human-made rank adjacency matrix wins against the deep-learning-based matrix, implies that there are still some parts that need touch of humans. We expect our Rank-GCN can make performance improvements especially when the predicted human joints are less accurate and unstable.
更多查看译文
关键词
Three-dimensional displays, Spatiotemporal phenomena, Robustness, Feature extraction, Convolutional neural networks, Action recognition, graph convolutional network, dynamic convolutional network
AI 理解论文
溯源树
样例
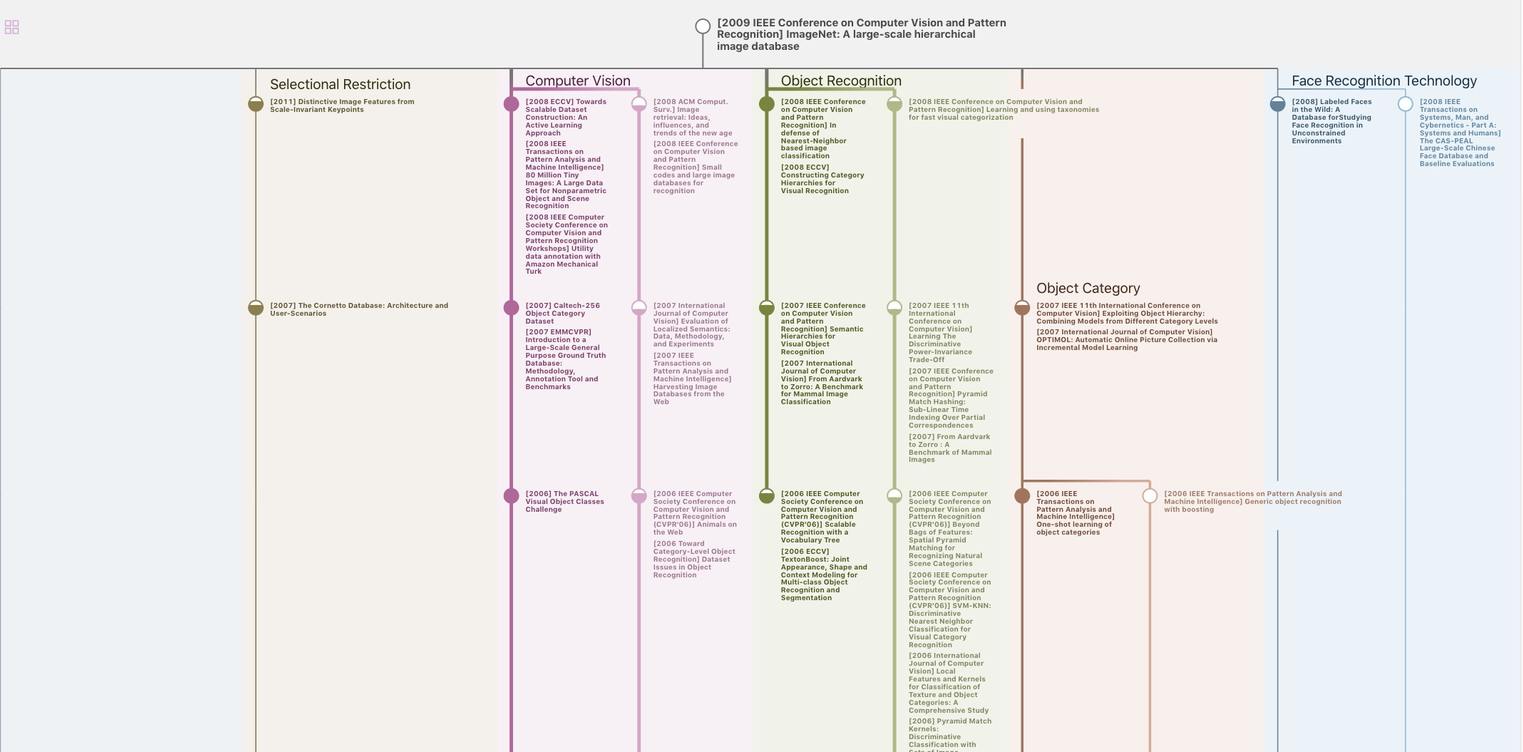
生成溯源树,研究论文发展脉络
Chat Paper
正在生成论文摘要