CGRM: A Context-Based Graph Reasoning Model for Fitting Detection
IEEE TRANSACTIONS ON INSTRUMENTATION AND MEASUREMENT(2022)
摘要
Transmission lines' status detection and fault diagnosis are based on the high-accuracy detection of typical fittings in aerial inspection photographs. Detecting tiny-size fittings in complicated sceneries is still an unresolved issue, nevertheless, because of the constraints of the small number of feature pixels. Considering the characteristics of fitting connection structures, we proposed a novel method named context-based graph reasoning model (CGRM), which is based on multiscale context, self-attention map, and graph learning. This proposed model includes one graph-based global context (GGC) subnetwork and one multiscale local context (MLC) subnetwork. The GGC effectively extracted the pixel-level global map based on multihead attention mechanism to capture global context and the graph convolutional neural network (GCN) was further used for reasoning. The MLC exploited the internal and external contextual information of multiscale for each unique proposal to capture its local context. The final detection result is then reached by fusing the global and local circumstances. Experimental results well demonstrate the superiority of the proposed model for fitting detection. In addition, compared with the original Faster R-CNN, the detection accuracy of CGRM is increased by 16.3%. It also provides a new idea for general object detection of tiny-size targets.
更多查看译文
关键词
Fitting, Feature extraction, Context modeling, Clamps, Power transmission lines, Insulators, Proposals, Attention mechanism, context, deep learning, fitting detection, graph convolutional neural network (GCN)
AI 理解论文
溯源树
样例
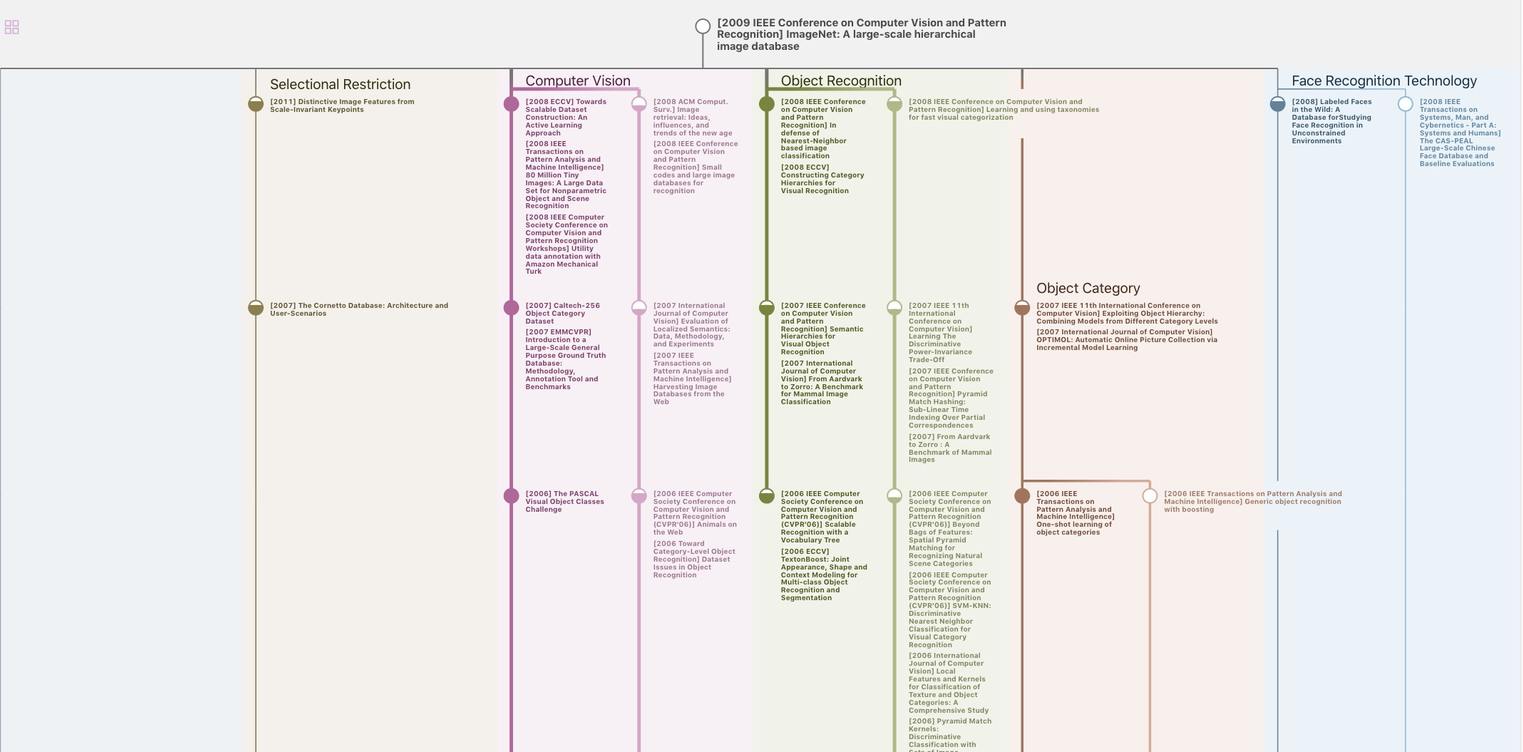
生成溯源树,研究论文发展脉络
Chat Paper
正在生成论文摘要