CMAN: Leaning Global Structure Correlation for Monocular 3D Object Detection
IEEE TRANSACTIONS ON INTELLIGENT TRANSPORTATION SYSTEMS(2022)
摘要
The key to 3D object detection is proper utilization of depth data. Compared with LiDAR based approaches, 3D object detection from a single image remains a challenging task due to the lack of structure information. Recent methods leverage monocular depth estimation as a way to produce 2D depth maps, and adopt the depth maps as additional source of input to explore structure information. However, these methods either encode local structure correlations, or encode long range structure correlations by iteratively passing local messages. In this work, we propose a cross modal attention network (CMAN) for monocular 3D object detection. It is built upon the self-attention module which learns attention map from single modal data. Our CMAN is able to encode structure correlations from depth data, and embed the structure correlations with appearance information which is learned from RGB data. Thanks to the attention learning mechanism, our CMAN learns global structure correlations without iteration. In order to reduce the computational burden, our CMAN adopts a novel node sampler to eliminate redundant nodes during the attention map calculation. Experiment results on benchmark KITTI3D dataset show that our proposed CMAN outperforms the state-of-the-art methods.
更多查看译文
关键词
Three-dimensional displays, Correlation, Object detection, Point cloud compression, Feature extraction, Laser radar, Estimation, 3D object detection, attention learning, structure learning, data fusion
AI 理解论文
溯源树
样例
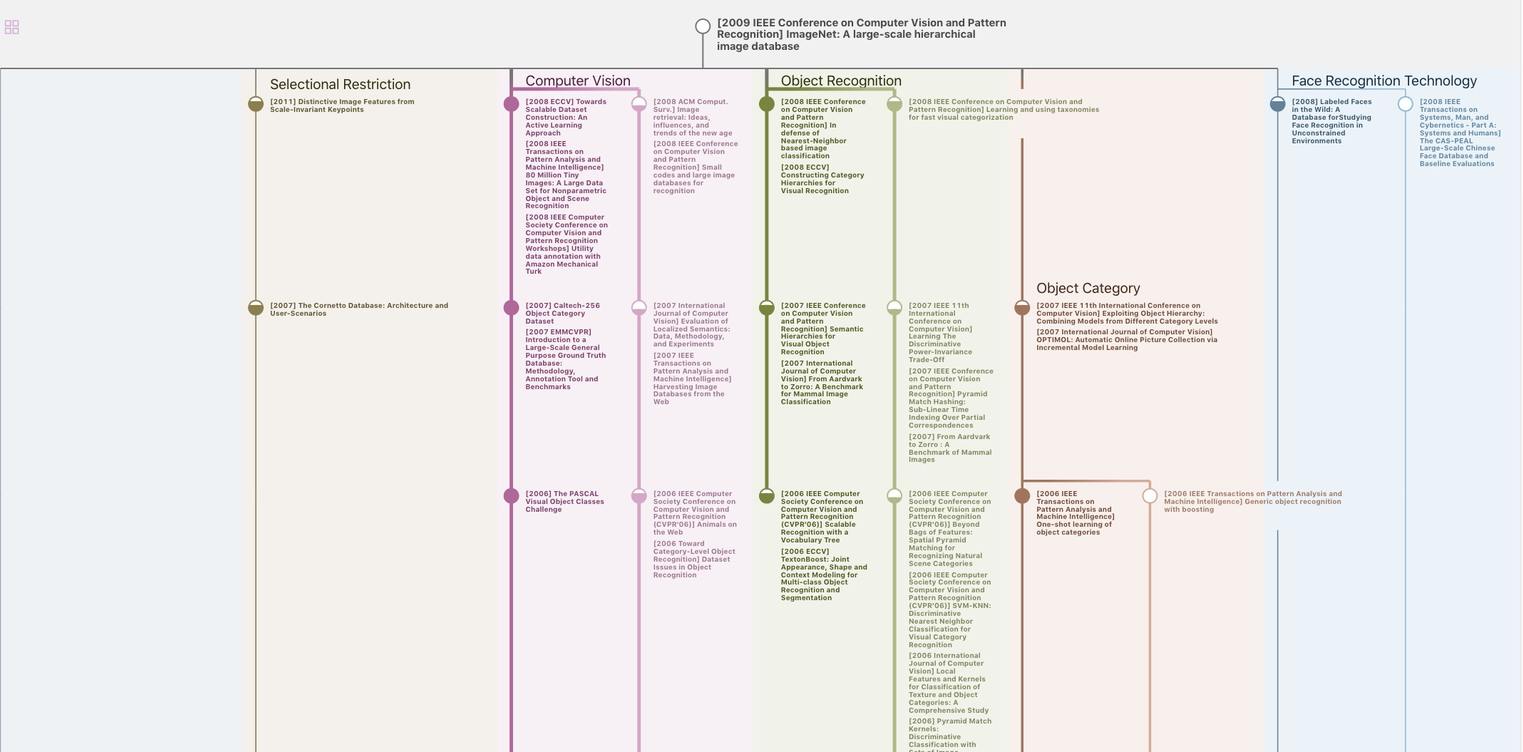
生成溯源树,研究论文发展脉络
Chat Paper
正在生成论文摘要