Unsupervised Anomaly Detection in Traffic Surveillance Based on Global Foreground Modeling
2022 IEEE International Conference on Imaging Systems and Techniques (IST)(2022)
摘要
One of the main challenges facing foreground detection methods in the applications of video analytic, is the continuous detection of the objects after they have stopped moving. In this paper, a novel global foreground modeling (GFM) guided background subtraction method, which is capable of detecting stopped foreground objects, is presented. Specifically, the GFM method, which detects both the moving and stopped foreground objects, guides the background modeling method, which detects only the moving objects, for the purpose of adaptive background updating. The main contributions of the paper are two-fold. First, a novel GFM guided background modeling method is proposed by adaptively updating the background model based on the differences between the two foreground masks produced by the GFM method and a background modeling method, such as Zivkovic's method, respectively. As a result, the proposed method both improves the foreground detection and detects the stopped foreground objects. Second, a boosting strategy is integrated into the proposed method for eliminating the false alarms caused by noise. Real traffic videos are used to evaluate the effectiveness of the proposed method. In particular, experimental results using the real traffic videos from the New Jersey Department of Transportation (NJDOT) show that the proposed GFM guided background modeling method is able to detect stopped foreground objects, such as stopped vehicles, in real time.
更多查看译文
关键词
unsupervised anomaly detection,foreground detection methods,continuous detection,novel global foreground modeling guided background subtraction method,stopped foreground objects,GFM method,background modeling method,moving objects,adaptive background updating,background model,foreground masks,Zivkovic's method,traffic videos
AI 理解论文
溯源树
样例
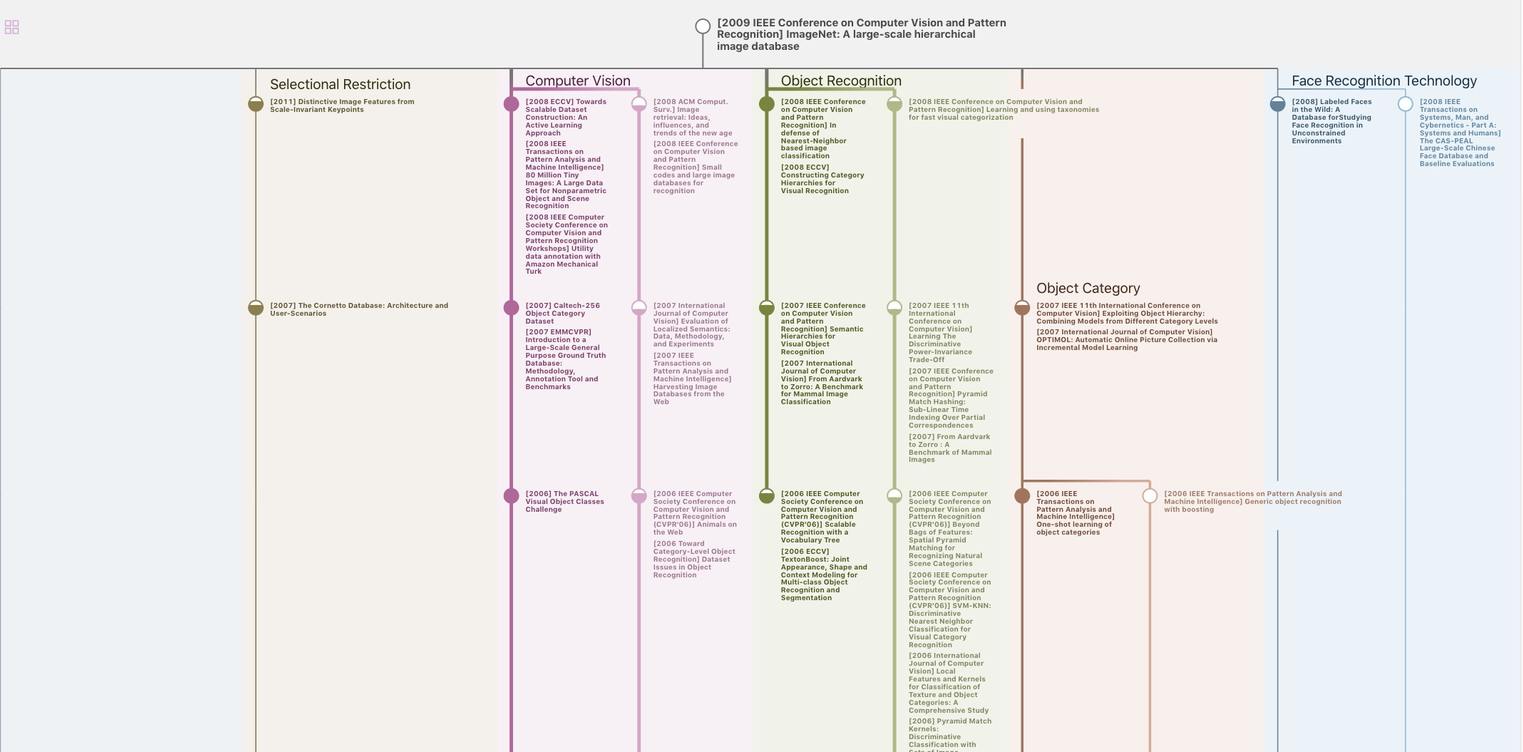
生成溯源树,研究论文发展脉络
Chat Paper
正在生成论文摘要