SR-ISTA-Net: Sparse Representation-Based Deep Learning Approach for SAR Imaging
IEEE GEOSCIENCE AND REMOTE SENSING LETTERS(2022)
摘要
Compressed sensing (CS) reconstruction of nonsparse scenes is one of the difficulties in synthetic aperture radar (SAR) imaging technology. Although the conventional CS method with sparse representation has proven applicable for nonsparse SAR reconstruction, its disadvantages are unsatisfactory imaging quality and high computational complexity under downsampling. In this letter, a novel deep learning approach for nonsparse SAR scene reconstruction is proposed based on sparse representation and the iterative shrinkage threshold algorithm (ISTA). Specifically, we first develop a sparse-representation-based imaging model associated with the l(1) sparse regularizer in nonlinear transform domains. Then, the advantages of the recurrent neural network (RNN) and convolutional neural network (CNN) are incorporated into an ISTA-inspired deep unfolded network (DUN) called SR-ISTA-Net, in which all the parameters are layer-varied rather than handcrafted. The experiments verify that the proposed SR-ISTA-Net can provide high-quality reconstruction results under nonsparse scenes while substantially reducing the imaging time.
更多查看译文
关键词
Compressed sensing (CS), deep learning, sparse reconstruction, sparse representation, synthetic aperture radar (SAR)
AI 理解论文
溯源树
样例
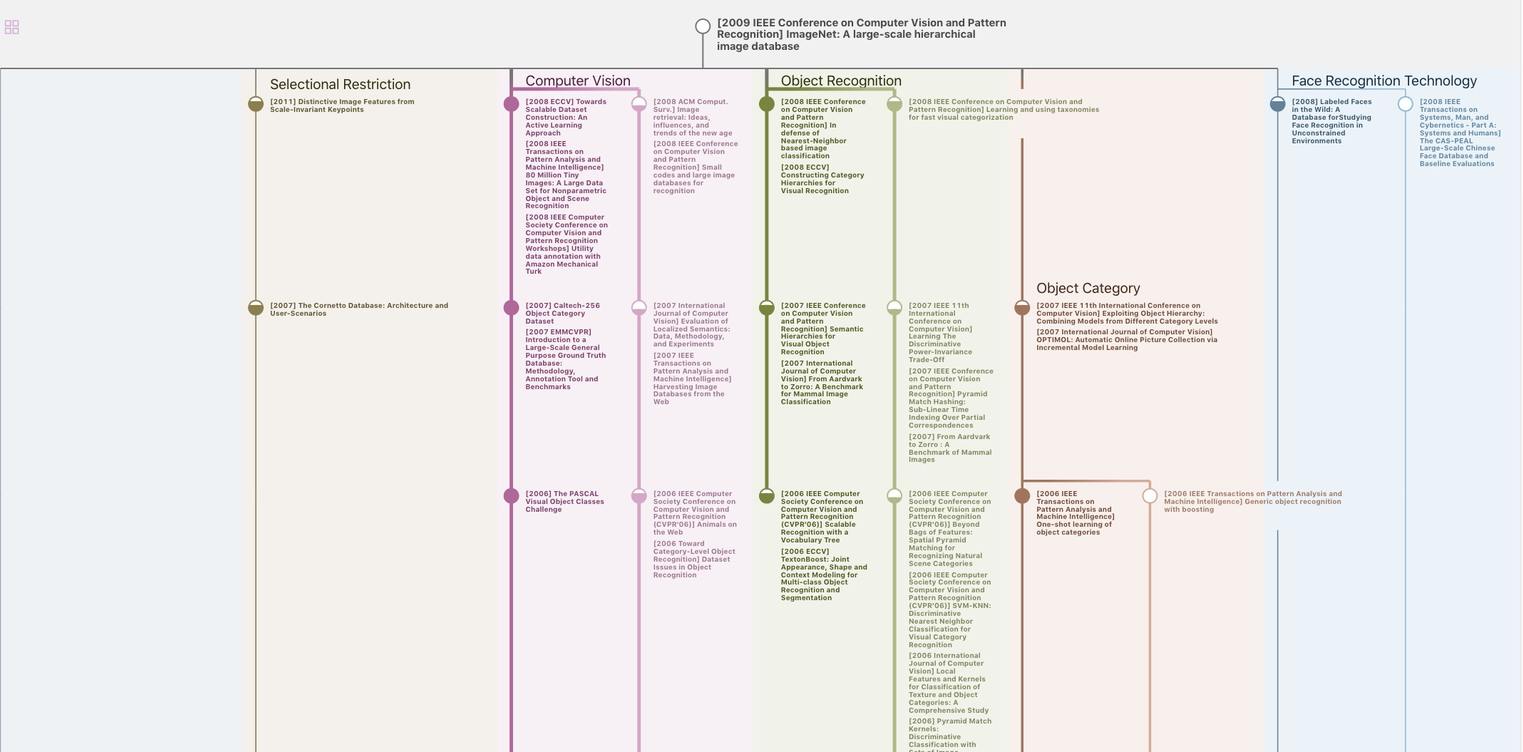
生成溯源树,研究论文发展脉络
Chat Paper
正在生成论文摘要