Uncertainties of gross primary productivity of Chinese grasslands based on multi-source estimation
FRONTIERS IN ENVIRONMENTAL SCIENCE(2022)
摘要
Gross primary productivity (GPP) is an important parameter in the carbon cycle and climate change studies. The results of GPP fluxes estimated based on multiple models or remote sensing vary widely, but current studies of GPP in Chinese grasslands tend to ignore data uncertainty. In this study, uncertainty analysis of GPP datasets estimated based on terrestrial ecosystem models and remote sensing was conducted using cross-validation, standard error statistics, and ensemble empirical modal decomposition. We found that 1) the fit coefficients R-2 of two-by-two cross-validation of GPP datasets mostly exceeded 0.8 at the global scale. 2) GPP from different sources were consistent in portraying the spatial and temporal patterns of GPP in Chinese grasslands. However, due to many differences in model structure, parameterization and driving data, some uncertainties still exist, especially in the parts of dry-cold areas where the standard deviations are relatively large. 3) Uncertainties were higher for future scenarios than for historical periods, and GPP uncertainties were much higher for future high-emissions scenarios than for low- and medium-emissions scenarios. This study highlighted the need for uncertainty analysis when GPP is applied to spatio-temporal analysis, and suggested that when comparing and assessing carbon balance conditions, multiple source data sets should be combined to avoid misleading conclusion due to uncertainty.
更多查看译文
关键词
gross primary productivity, uncertainty, Chinese grasslands, MsTIMP, ISIMIP
AI 理解论文
溯源树
样例
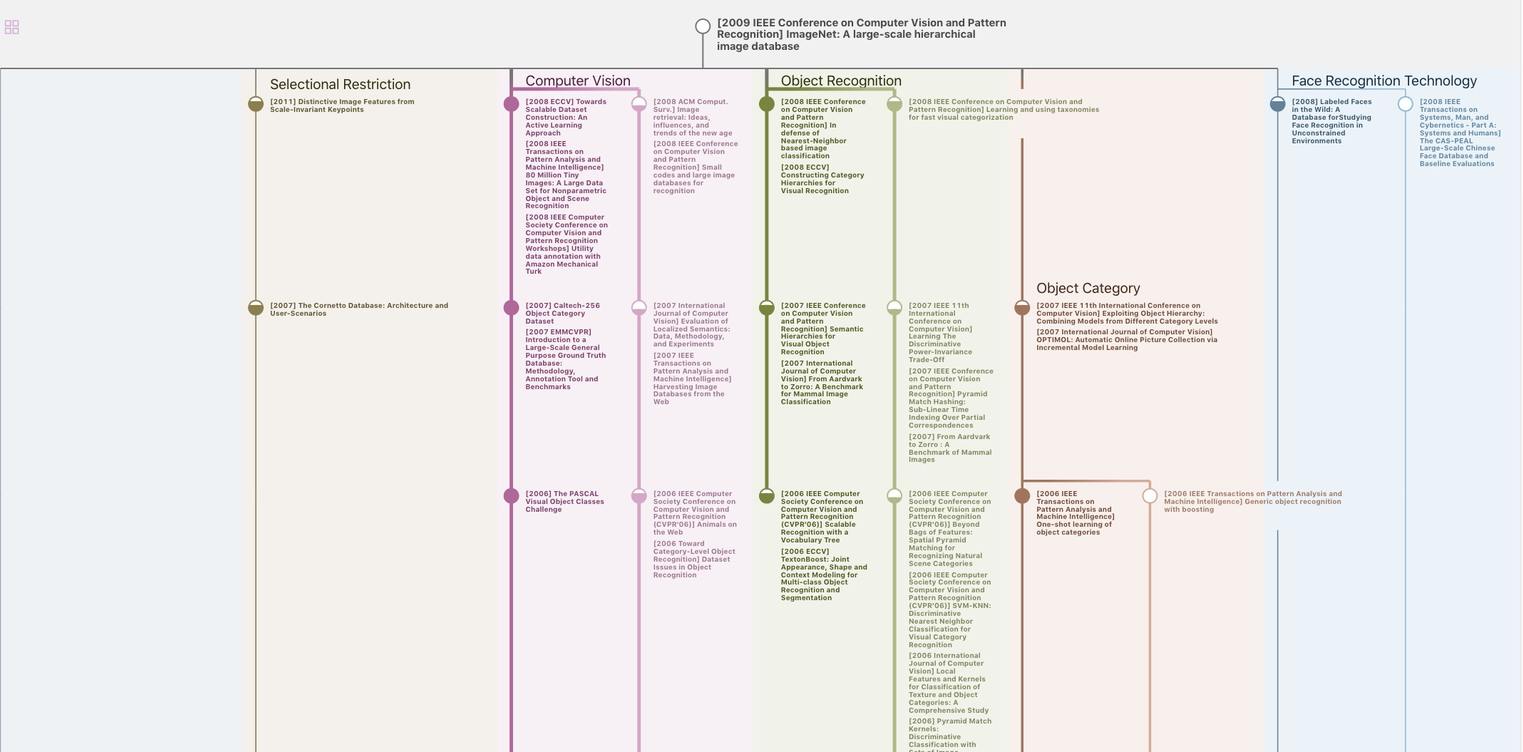
生成溯源树,研究论文发展脉络
Chat Paper
正在生成论文摘要