Accelerated Inference of Face Detection under Edge-Cloud Collaboration
APPLIED SCIENCES-BASEL(2022)
Abstract
Model compression makes it possible to deploy face detection models on devices with limited computing resources. Edge-cloud collaborative inference, as a new paradigm of neural network inference, can significantly reduce neural network inference latency. Inspired by these two techniques, this paper adopts a two-step acceleration strategy for the CenterNet model. Firstly, the model pruning method is used to prune the convolutional layer and the deconvolutional layer to obtain a preliminary acceleration effect. Secondly, the neural network is segmented by the optimizer to make full use of the computing resources on the edge and the cloud to further accelerate the inference of the neural network. In the first strategy, we achieve a 62.12% reduction in inference latency compared to the state-of-the-art object detection model Blazeface. Additionally, with a two-step speedup strategy, our method is only 26.5% of the baseline when the bandwidth is 500 kbps.
MoreTranslated text
Key words
collaborative intelligence,deep learning,deconvolution pruning,face detection
AI Read Science
Must-Reading Tree
Example
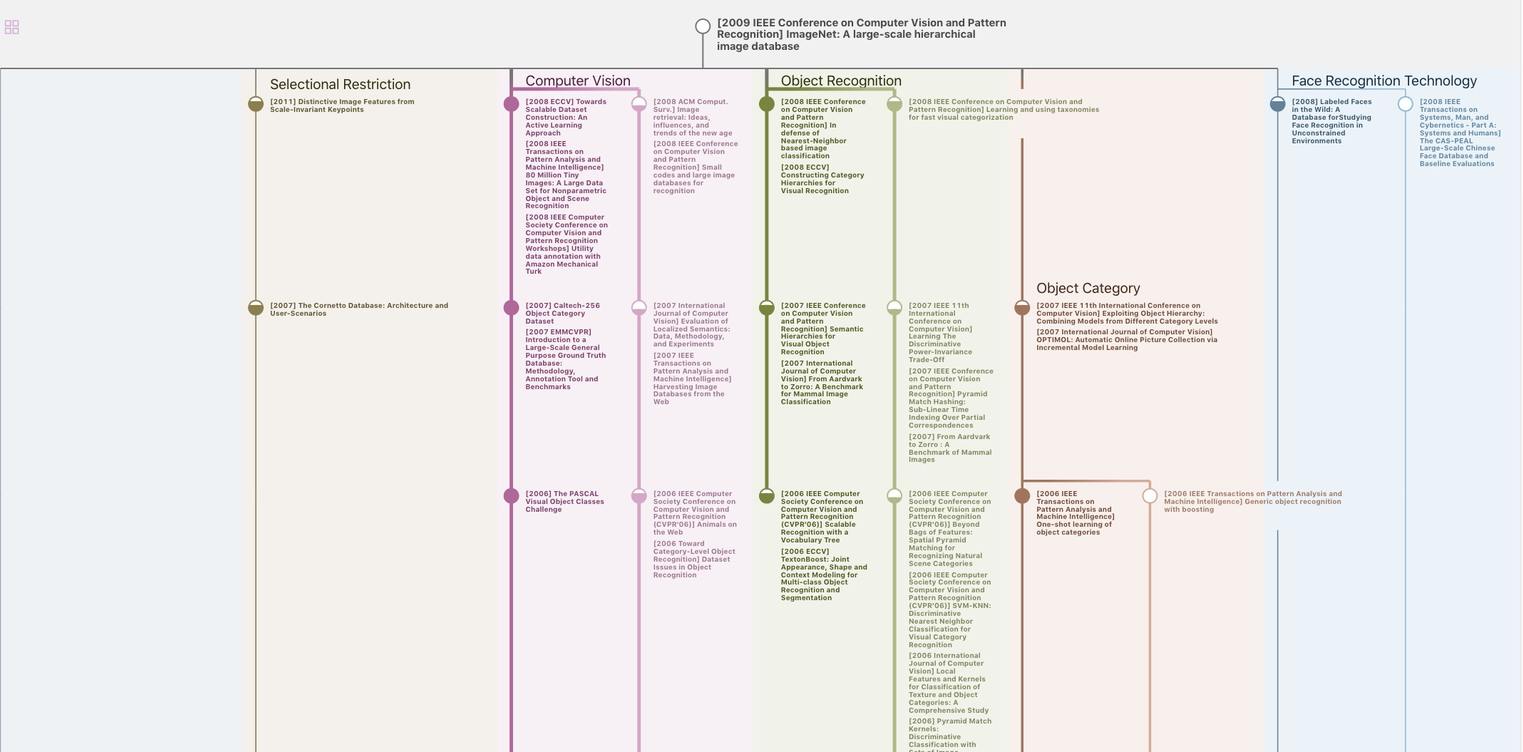
Generate MRT to find the research sequence of this paper
Chat Paper
Summary is being generated by the instructions you defined