MACHINE LEARNING-BASED MODELING AND OPTIMIZATION IN HARD TURNING OF AISI D6 STEEL WITH ADVANCED AlTiSiN-COATED CARBIDE INSERTS TO PREDICT SURFACE ROUGHNESS AND OTHER MACHINING CHARACTERISTICS
SURFACE REVIEW AND LETTERS(2022)
摘要
In recent times, mechanical and production industries are facing increasing challenges related to the shift towards sustainable manufacturing. In this work, the machining was performed in dry cutting condition with the newly developed AlTiSiN-coated carbide inserts coated through scalable pulsed power plasma technique, and a dataset was generated for different machining parameters and output responses. The machining parameters are speed, feed and depth of cut, while the output responses are surface roughness, cutting force, crater wear length, crater wear width and flank wear. Abrasion and adhesion were found to be the two dominant wear mechanisms. With speed, the tool wear was found to increase. Cutting force was found to increase rapidly for higher speed ranges (70, 80 and 90m/min). But reduction of cutting force was observed in both low and medium ranges of cutting speed (40, 50, 55 and 60m/min). With higher values of feed and depth of cut, higher cutting force was also noticed. With the variation of depth of cut, the machined surface morphology and machined surface roughness were found to deteriorate. With higher feed and depth of cut values, more surface damages were observed compared to low values of feed and depth of cut. Both the feed rate and tool-chip contact length contributed significantly to the formation of crater on the tool rake surface. At high speed, continuous chips were observed. Both chip sliding and sticking were observed on the tool rake face. The data collected from the machining operation was used for the development of machine learning (ML)-based surrogate models to test, evaluate and optimize various input machining parameters. Different ML approaches such as polynomial regression (PR), random forests (RF) regression, gradient boosted (GB) trees and adaptive boosting (AB)-based regression were used to model different output responses in the hard machining of AISI D6 steel. Out of the four ML methodologies, RF and PR performed better in comparison to the other two algorithms in terms of the R-2 values of predictions. The surrogate models for different output responses were used to prepare a complex objective function for the germinal center algorithm-based optimization of the machining parameters of the hard turning operation.
更多查看译文
关键词
Hard turning, AlTiSiN coating, surface roughness, flank wear, cutting force, crater wear, machine learning
AI 理解论文
溯源树
样例
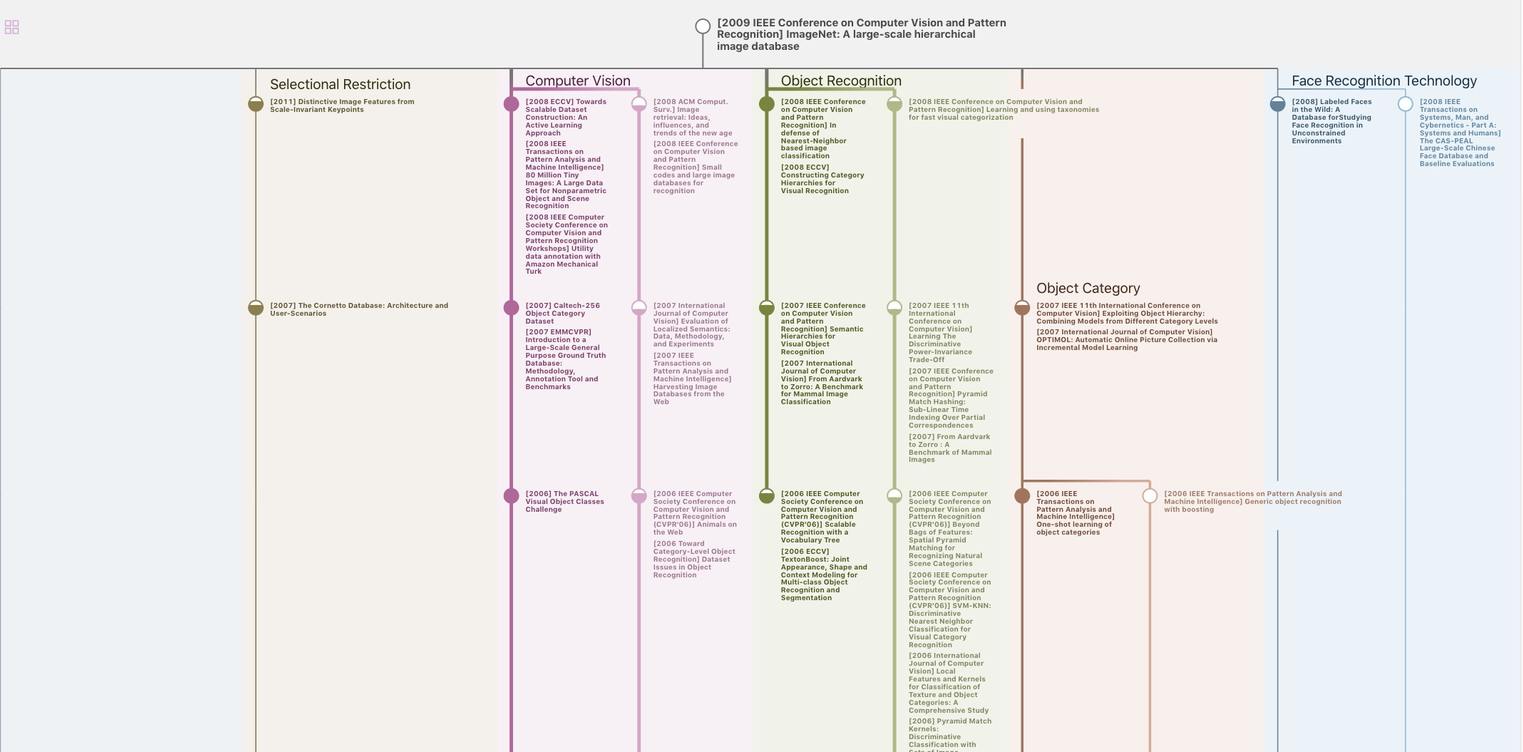
生成溯源树,研究论文发展脉络
Chat Paper
正在生成论文摘要