Maximum interpolable gap length in missing smartphone-based GPS mobility data
TRANSPORTATION(2024)
摘要
Passively-generated location data have the potential to augment mobility and transportation research, as demonstrated by a decade of research. A common trait of these data is a high proportion of missingness. Naive handling, including list-wise deletion of subjects or days, or linear interpolation across time gaps, has the potential to bias summary results. On the other hand, it is unfeasible to collect mobility data at frequencies high enough to reflect all possible movements. In this paper, we describe the relationship between the temporal and spatial aspects of these data gaps, and illustrate the impact on measures of interest in the field of mobility. We propose a method to deal with missing location data that combines a so-called top-down ratio segmentation method with simple linear interpolation. The linear interpolation imputes missing data. The segmentation method transforms the set of location points to a series of lines, called segments. The method is designed for relatively short gaps, but is evaluated also for longer gaps. We study the effect of our imputation method for the duration of missing data using a completely observed subset of observations from the 2018 Statistics Netherlands travel study. We find that long gaps demonstrate greater downward bias on travel distance, movement events and radius of gyration as compared to shorter but more frequent gaps. When the missingness is unrelated to travel behavior, total sparsity can reach levels of up to 20% with gap lengths of up to 10 min while maintaining a maximum 5% downward bias in the metrics of interest. Temporal aspects can increase these limits; sparsity occurring in the evening or night hours is less biasing due to fewer travel behaviors.
更多查看译文
关键词
Mobility,Sensors,Smartphone data,Missing data,Travel diary
AI 理解论文
溯源树
样例
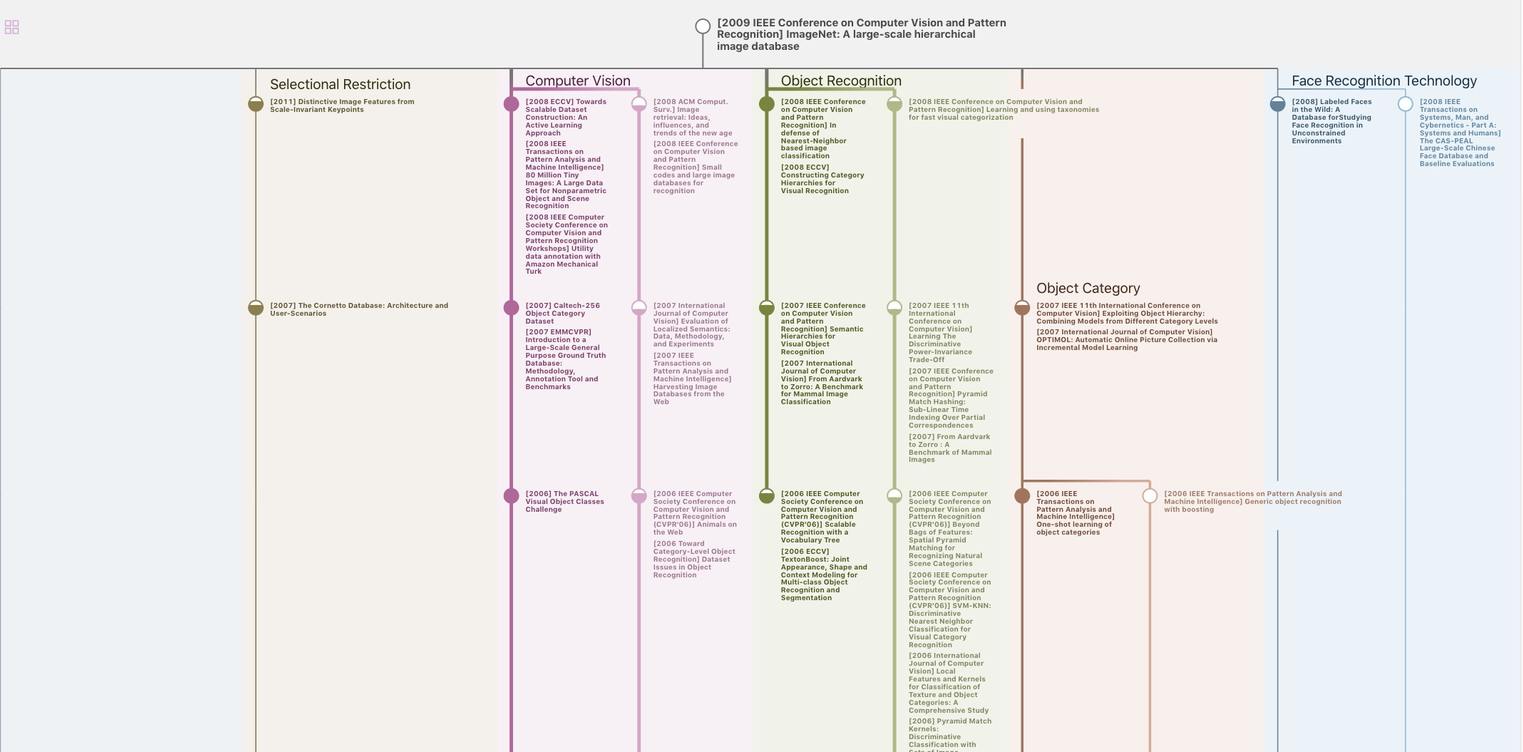
生成溯源树,研究论文发展脉络
Chat Paper
正在生成论文摘要