Medical Image Segmentation Using U-Net and Progressive Neuron Expansion
PATTERN RECOGNITION AND TRACKING XXXIII(2022)
摘要
With fast-growing computing power and large amounts of data availability, deep learning (DL) algorithms are achieving unprecedented success across different fields. One of its great achievements in health care is medical imaging. Medical image segmentation, such as lung cancer segmentation, is an important tool that facilitates clinical decision systems. U-Net, a recent innovative DL architecture, has shown great promise in segmenting regions of interest in medical images. One of the key advantages of U-Net is that it effectively constructs contracting and expanding paths with symmetric network connection which allows for capturing context information and enabling precise localization in a single network. Although U-Net and its variants have been widely adopted in the medical image segmentation task, there are some limitations that need to be addressed to meet specific requirements, including hardware memory consumption and segmentation accuracy. In this work, we propose a new U-Net based DL architecture, U-PEN (U-net with Progressively Expanded Neuron), that requires less memory on hardware while achieving highly accurate segmentation. The underlying hypothesis behind the proposed architecture is that this model, when compared to existing models, can efficiently capture image context via incorporating nonlinear functions into hidden neurons expansion in the encoder path. The proposed network can eliminate additional convolutional layers thus producing less trainable parameters compared to U-Net. The proposed DL model was tested on two benchmark datasets, namely DRIVE and CHASH_DB1, for different medical image segmentation problems. The experimental results show that the suggested architecture is effective, yielding better performance over U-Net and Residual U-Net in most of the experiments.
更多查看译文
关键词
Deep learning, U-Net, image segmentation, progressive expansion, medical image analysis
AI 理解论文
溯源树
样例
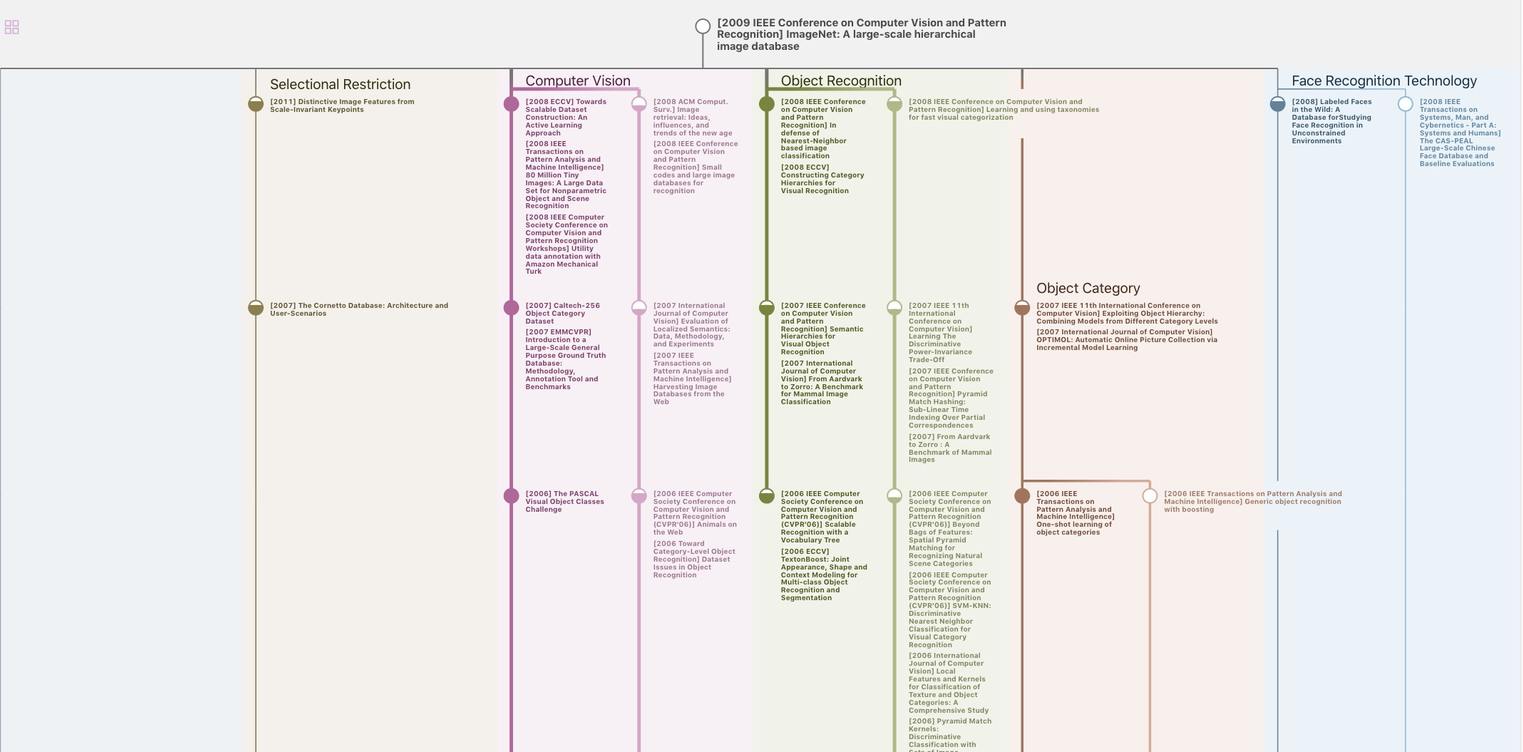
生成溯源树,研究论文发展脉络
Chat Paper
正在生成论文摘要