Adaptive Industrial Control System Attack Sample Expansion Algorithm Based on Generative Adversarial Network
APPLIED SCIENCES-BASEL(2022)
Abstract
The scarcity of attack samples is the bottleneck problem of anomaly detection of underlying business data in the industrial control system. Predecessors have done a lot of research on temporal data generation, but most of them are not suitable for industrial control attack sample generation. The change patterns of the characteristics of the underlying business data attack samples can be divided into three types: oscillation type, step type, and pulse type. This paper proposes an adaptive industrial control attack sample expansion algorithm based on GAN, which expands the three types of features in different ways. The basic network structure of data expansion adopts GAN. According to the characteristics of oscillation type changes, momentum is selected as the optimizer. Aiming at the characteristics of step type changes, the Adam optimization method is improved. For pulse type features, attack samples are generated according to the location and length of the pulse. Compared with previous time-series data generation methods, this method is more targeted for each feature and has higher similarities.
MoreTranslated text
Key words
generative adversarial networks, improved Adam optimization method, industrial control safety, industrial control attack sample generation
AI Read Science
Must-Reading Tree
Example
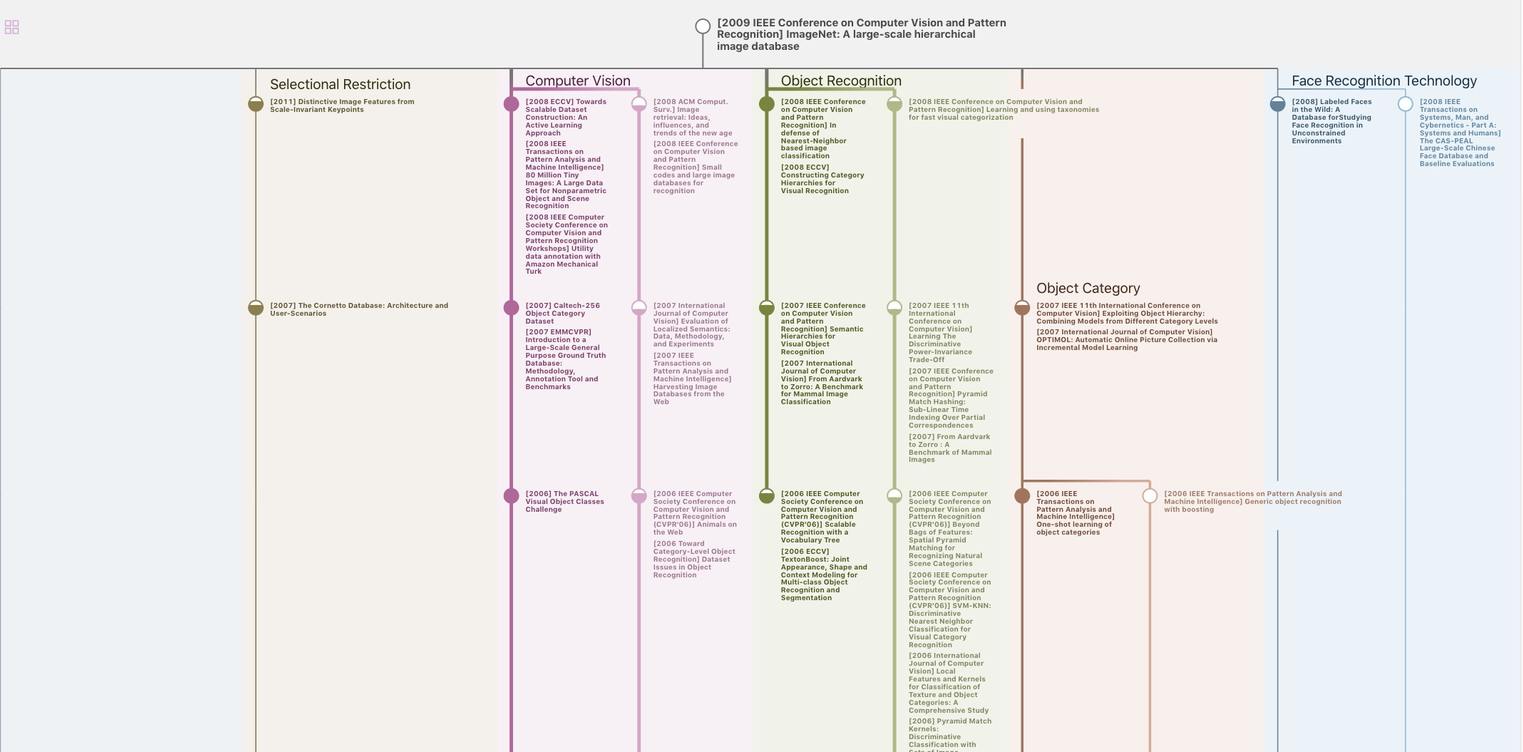
Generate MRT to find the research sequence of this paper
Chat Paper
Summary is being generated by the instructions you defined