An Optimized and Hybrid Framework for Image Processing Based Network Intrusion Detection System
CMC-COMPUTERS MATERIALS & CONTINUA(2022)
摘要
The network infrastructure has evolved rapidly due to the ever-increasing volume of users and data. The massive number of online devices and users has forced the network to transform and facilitate the operational necessities of consumers. Among these necessities, network security is of prime significance. Network intrusion detection systems (NIDS) are among the most suitable approaches to detect anomalies and assaults on a network. However, keeping up with the network security requirements is quite challenging due to the constant mutation in attack patterns by the intruders. This paper presents an effective and prevalent framework for NIDS by merging image processing with convolution neural networks (CNN). The proposed framework first converts non-image data from network traffic into images and then further enhances those images by using the Gabor filter. The images are then classified using a CNN classifier. To assess the efficacy of the recommended method, four benchmark datasets i.e., CSE-CIC-IDS2018, CIC-IDS-2017, ISCX-IDS 2012, and NSL-KDD were used. The proposed approach showed higher precision in contrast with the recent work on the mentioned datasets. Further, the proposed method is compared with the recent well-known image processing methods for NIDS.
更多查看译文
关键词
Anomaly detection, convolution neural networks, deep learning, image processing, intrusion detection, network intrusion detection
AI 理解论文
溯源树
样例
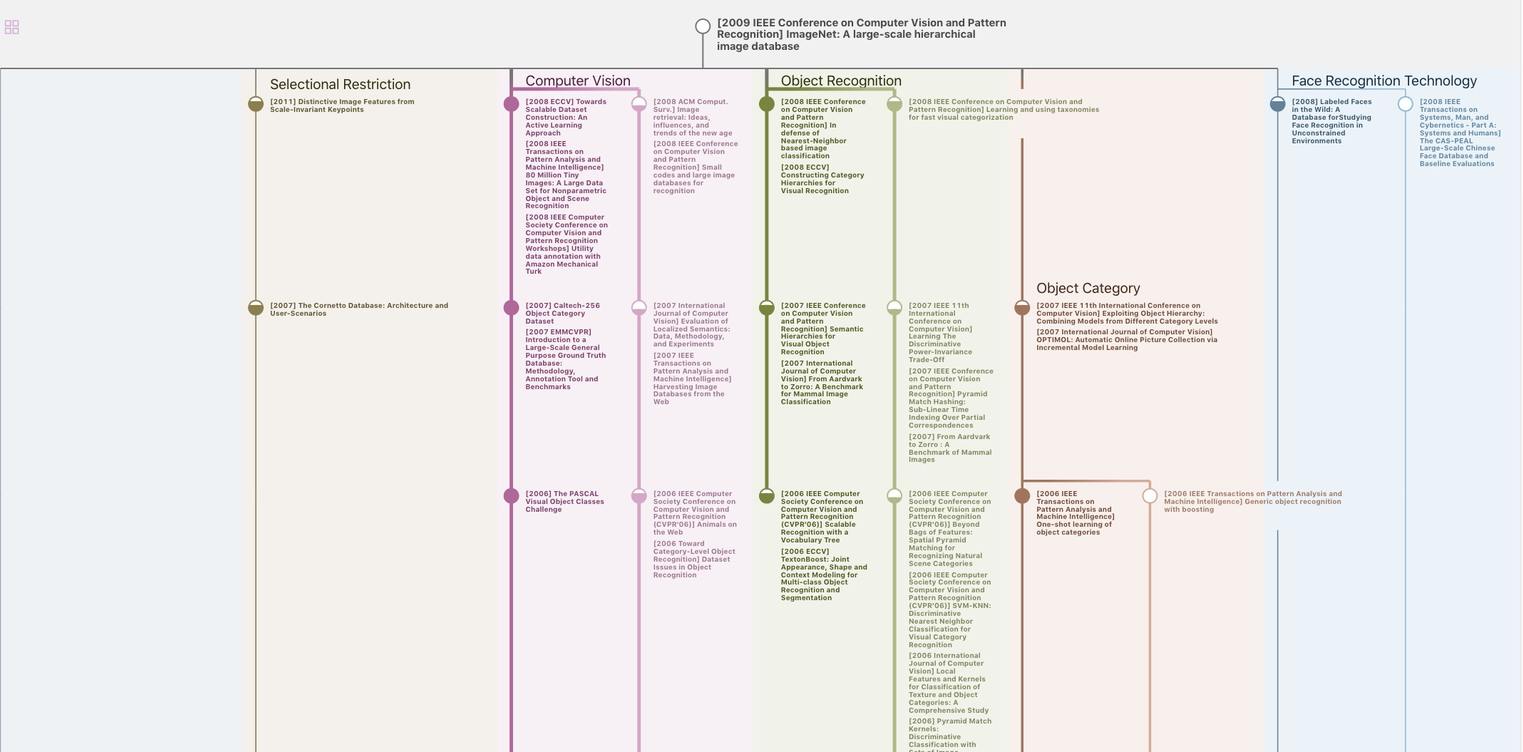
生成溯源树,研究论文发展脉络
Chat Paper
正在生成论文摘要