A Deep Learning Micro-Scale Model to Estimate the CO2 Emissions from Light-Duty Diesel Trucks Based on Real-World Driving
ATMOSPHERE(2022)
摘要
On-road carbon dioxide (CO2) emissions from light-duty diesel trucks (LDDTs) are greatly affected by driving conditions, which may be better predicted with the sequence deep learning model as compared to traditional models. In this study, two typical LDDTs were selected to investigate the on-road CO2 emission characteristics with a portable emission measurement system (PEMS) and a global position system (GPS). A deep learning-based LDDT CO2 emission model (DL-DTCEM) was developed based on the long short-term memory network (LSTM) and trained by the measured data with the PEMS. Results show that the vehicle speed, acceleration, VSP, and road slope had obvious impacts on the transient CO2 emission rates. There was a rough positive correlation between the vehicle speed, road slope, and CO2 emission rates. The CO2 emission rate increased significantly when the speed was >5 m/s, especially at high acceleration. The correlation coefficient (R-2) and the root mean square error (RMSE) between the monitored CO2 emissions with PEMS and the predicted values with the DL-DTCEM were 0.986-0.990 and 0.165-0.167, respectively. The results proved that the model proposed in this study can predict very well the on-road CO2 emissions from LDDTs.
更多查看译文
关键词
vehicle emission model, deep learning, light-duty diesel truck (LDDT), CO2 emissions, portable emission measurement system (PEMS), long short-term memory network (LSTM)
AI 理解论文
溯源树
样例
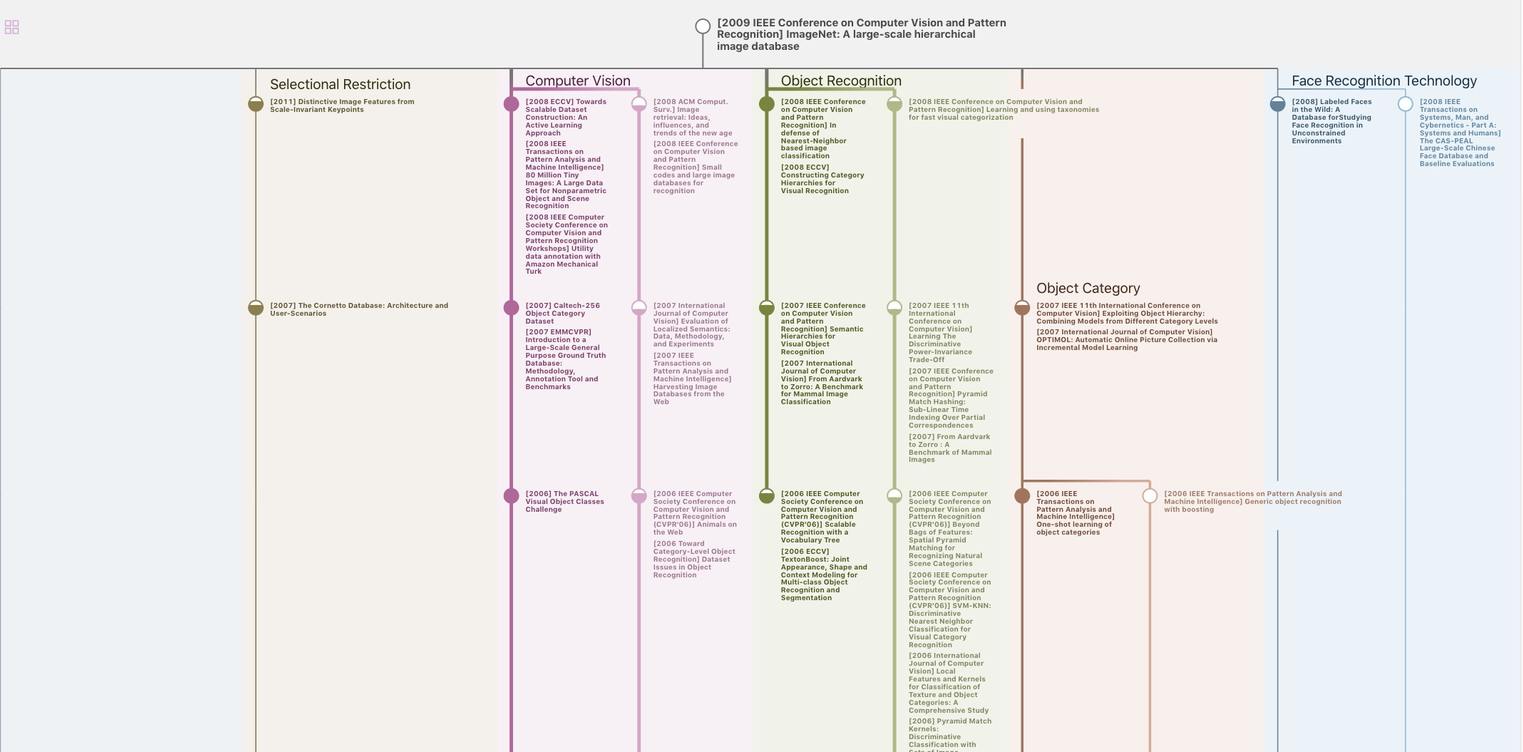
生成溯源树,研究论文发展脉络
Chat Paper
正在生成论文摘要