Perioperative Outcomes and Learning Curve of Robot-Assisted McKeown Esophagectomy
Journal of gastrointestinal surgery : official journal of the Society for Surgery of the Alimentary Tract(2022)
Abstract
Background This study aimed to evaluate the perioperative outcomes of patients undergoing robot-assisted McKeown esophagectomy (RAME) and the learning curves of surgeons performing RAME at a single center. Methods Perioperative outcomes of RAME and video-assisted McKeown esophagectomy (VAME) were compared after eliminating confounding factors by propensity score matching (PSM). The cumulative sum (CUSUM) method was used to evaluate the learning curves of RAME for a single surgical team. Results In general, a total of 198 patients with esophageal cancer (RAME: 45 patients, VAME: 153 patients) were included in this study, and 43 pairs of patients receiving RAME or VAME were matched using 1:1 PSM analysis. Those in the RAME group had more lymph nodes dissected in the total lymph nodes (median 29.0 vs . 26.0, P = 0.011) and the upper mediastinum (median 8.0 vs. 6.0, P < 0.001), especially the left recurrent laryngeal nerve (RLN) lymph node (median 4.0 vs. 2.0, P = 0.001). According to the trend of the CUSUM plot, the learning curve was divided into two stages at the 20th RAME procedure. After mastering the learning curve, RAME harvested a significantly higher number of upper mediastinal lymph nodes (median 9.0 vs. 6.0, P = 0.001), left RLN lymph nodes (median 5.0 vs. 3.5, P = 0.003), and right RLN lymph nodes (median 4.0 vs. 2.0, P = 0.002). Meanwhile, the incidence of postoperative pneumonia in the proficiency phase was significantly lower than that in the learning phase (4.0% vs. 25.0%, P = 0.04). Conclusions RAME improved left RLN lymph node dissection. Surgeons with extensive VAME experience need at least 20 cases to achieve early proficiency in RAME.
MoreTranslated text
Key words
Esophageal cancer,Robotic esophagectomy,Perioperative outcomes,Learning curve
AI Read Science
Must-Reading Tree
Example
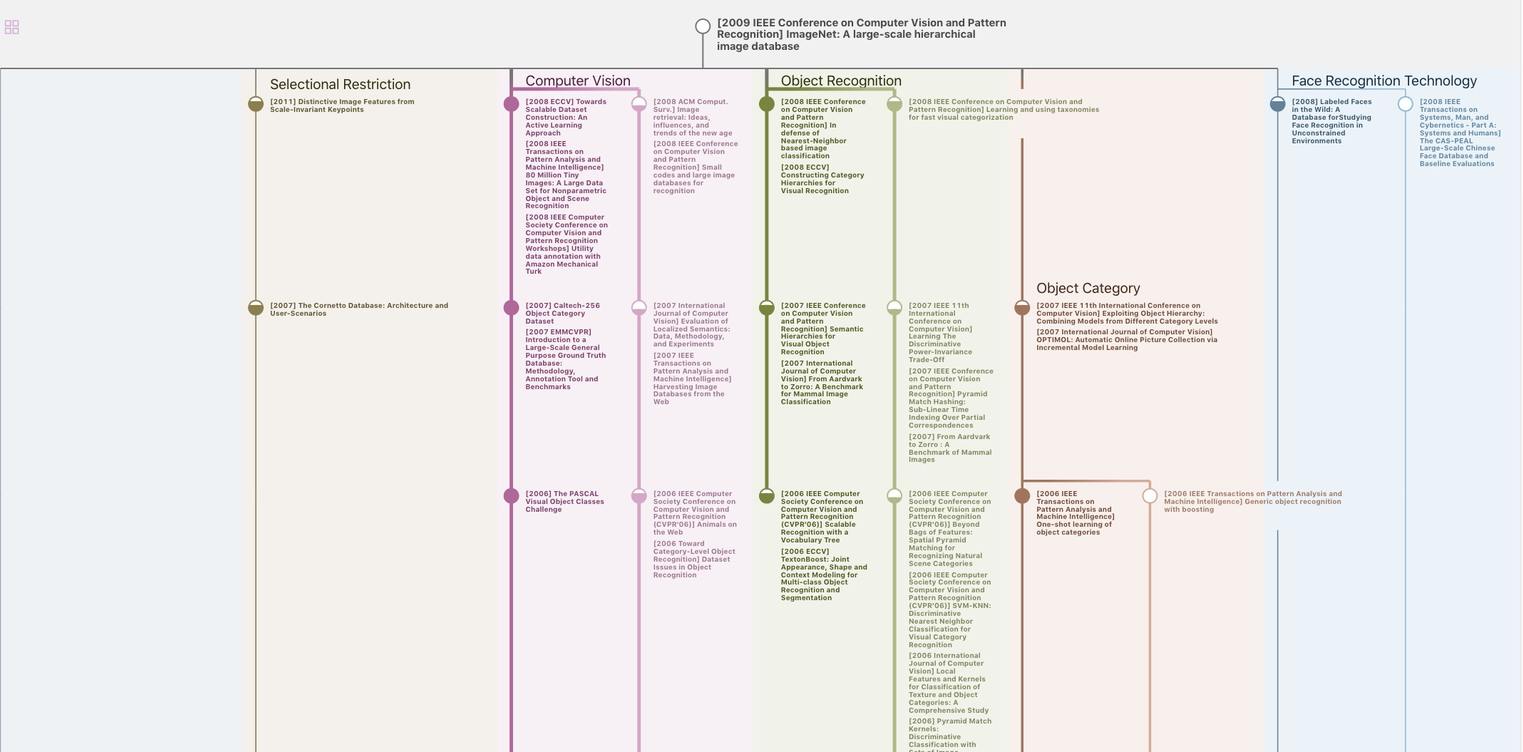
Generate MRT to find the research sequence of this paper
Chat Paper
Summary is being generated by the instructions you defined