A Surrogate-Assisted Bi-Swarm Evolutionary Algorithm for Expensive Optimization
Applied intelligence(2022)
Abstract
This article introduces an efficient surrogate-assisted bi-swarm evolutionary algorithm (SABEA) with hybrid and ensemble strategies for computationally expensive optimization problems. In SABEA, the evolutionary swarm is randomly partitioned into two sub-swarms to maintain diversity of the population. One sub-swarm evolves using the differential evolution (DE) and the other one evolves using teaching-learning-based optimization (TLBO). The proposed SABEA has strong exploration and exploitation capabilities by taking advantages of these two powerful algorithms. Besides, both the global and the local surrogate models cooperate effectively in the proposed SABEA for estimating the fitness value. The global model is established with all samples in the database for global search, and the local model is created with training samples around the current swarm for local search. In addition, a restart mechanism and two model management schemes, namely the individual-based and generation-based, are effectively integrated in the proposed algorithm to make SABEA more strong. Twenty benchmark functions and the tension/compression spring design problem are employed to assess the proposed SABEA. Comprehensive experimental results demonstrate that our proposed SABEA has superior performance comparing with several state-of-the-art competing algorithms on most of the test problems with low, medium and high dimensions.
MoreTranslated text
Key words
Surrogate-assisted,Expensive problems,Bi-swarm evolutionary algorithm,Differential evolution,Teaching-learning-based optimization
AI Read Science
Must-Reading Tree
Example
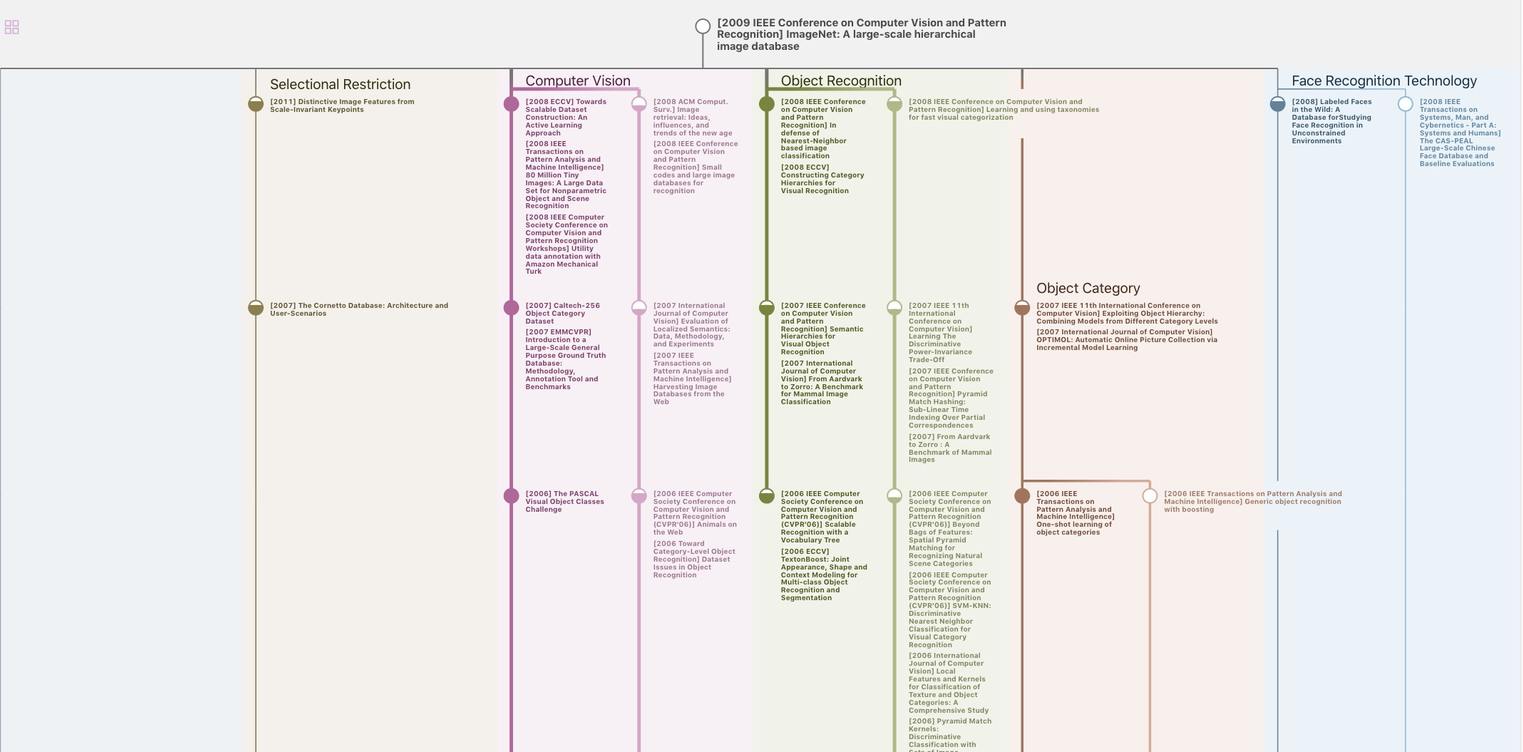
Generate MRT to find the research sequence of this paper
Chat Paper
Summary is being generated by the instructions you defined