CDFF: a fast and highly accurate method for recognizing traffic signs
NEURAL COMPUTING & APPLICATIONS(2022)
摘要
Convolutional neural networks method is a commonly used traffic sign recognition method based on deep learning over recent years. However, traffic signs contain objects of different sizes. Since small objects occupy a small input image area, the features that can be extracted are less, and the detection difficulty is greater than that of medium and large objects, and it is still challenging to achieve high-speed and high-accuracy detection of all objects of different sizes at the same time. In this paper, a model for detecting traffic signs is proposed, namely CDFF and CDFF-s. The model contains the following four modules: (1) in the backbone part of the model, we apply an improved activation function FMish to increase training stability, (2) after the backbone of the model, we apply the DFb-SPP module to perform context and semantic fusion, (3) in the neck part of the model, we use the DFb module for feature fusion, which also reduces the number of parameters, and (4) in the head part of the model, we propose a loss function SCIoU, which is optimized for small objects and the model is converged faster. The experimental results on the general traffic sign datasets TT100K and LISA show that the proposed two models can achieve accurate small object detection without losing the detection accuracy of medium and large objects. In addition, excellent results are also obtained on the remote sensing dataset RSOD with similar object size distribution. Meanwhile, the detection speed is faster than YOLOv4, which can meet the accuracy and real-time requirements of automatic driving systems and assisted driving systems.
更多查看译文
关键词
Convolutional neural networks,Object detection,Traffic signs recognition,Neural network pruning
AI 理解论文
溯源树
样例
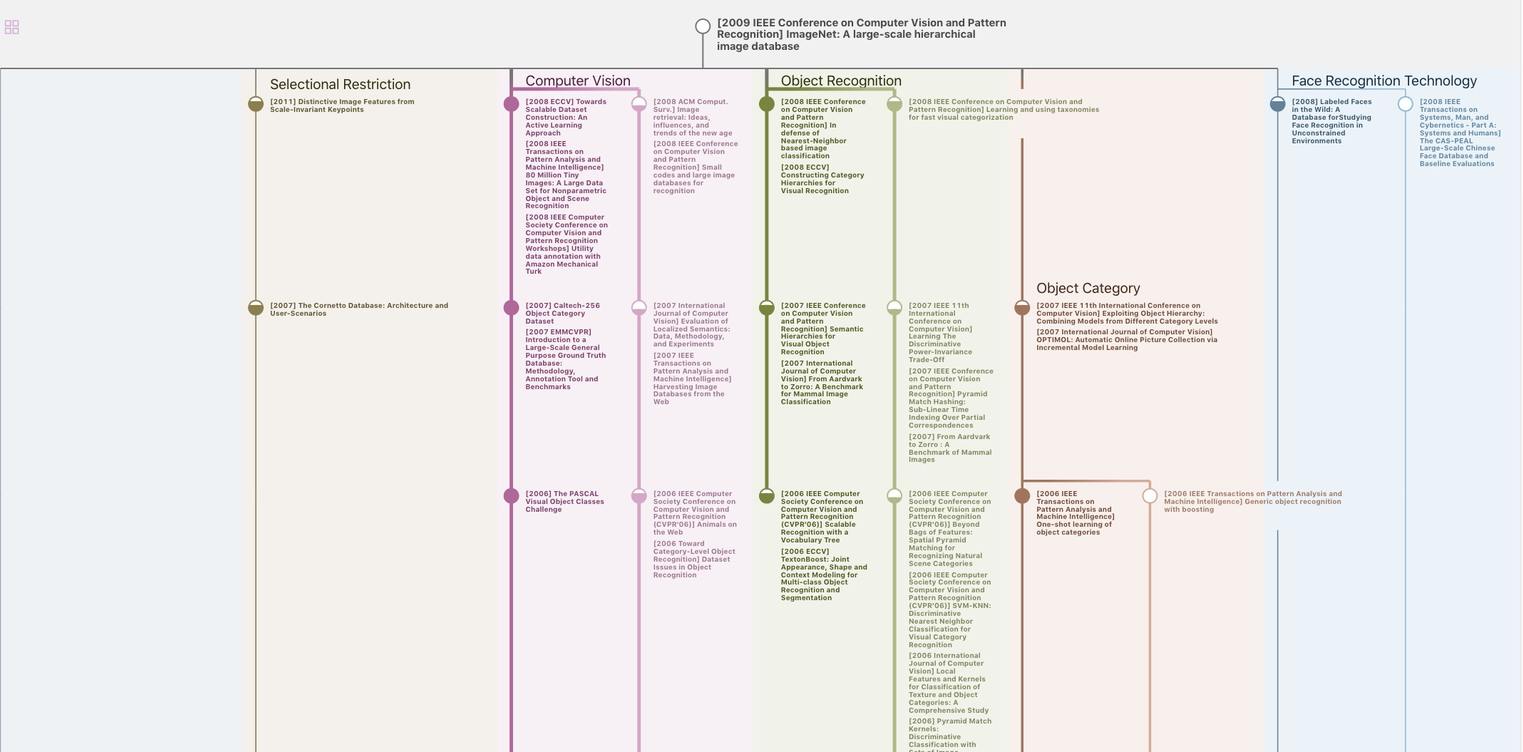
生成溯源树,研究论文发展脉络
Chat Paper
正在生成论文摘要