Experimental Demonstration of Multilevel Resistive Random Access Memory Programming for up to Two Months Stable Neural Networks Inference Accuracy
ADVANCED INTELLIGENT SYSTEMS(2022)
摘要
Crossbars of resistive memories, or memristors, provide a road to reduce the energy consumption of artificial neural networks, by naturally implementing multiply accumulate operations, their most basic calculations. However, a major challenge of implementing robust hardware neural networks is the conductance instability over time of resistive memories, due to the local recombination of oxygen vacancies. This effect causes resistive memory-based neural networks to rapidly lose accuracy, an issue that is sometimes overlooked. Herein, this conductance instability issue is shown, which can be avoided without changing the material stack of the resistive memory by exploiting an original programming strategy. This technique relies on program-and-verify loops with appropriately chosen wait times and ensures that the resistive memories are programmed into states with stable filaments. To test the strategy, a 32 x 32 in-memory computing system, fabricated in a hybrid complementary metal-oxide-semiconductor (CMOS)/hafnium oxide technology, is programmed to classify heart arrhythmia from electrocardiogram. When the resistive memories are programmed conventionally, the system loses accuracy within hours. In contrast, when using this technique, the system maintains an accuracy of 95% over more than 2 months. These results highlight the potential of resistive memory for the implementation of low-power neural networks with long-term stability.
更多查看译文
关键词
in-memory computing, memristors, multilevel cells, neural networks, neuromorphic, resistive random access memories
AI 理解论文
溯源树
样例
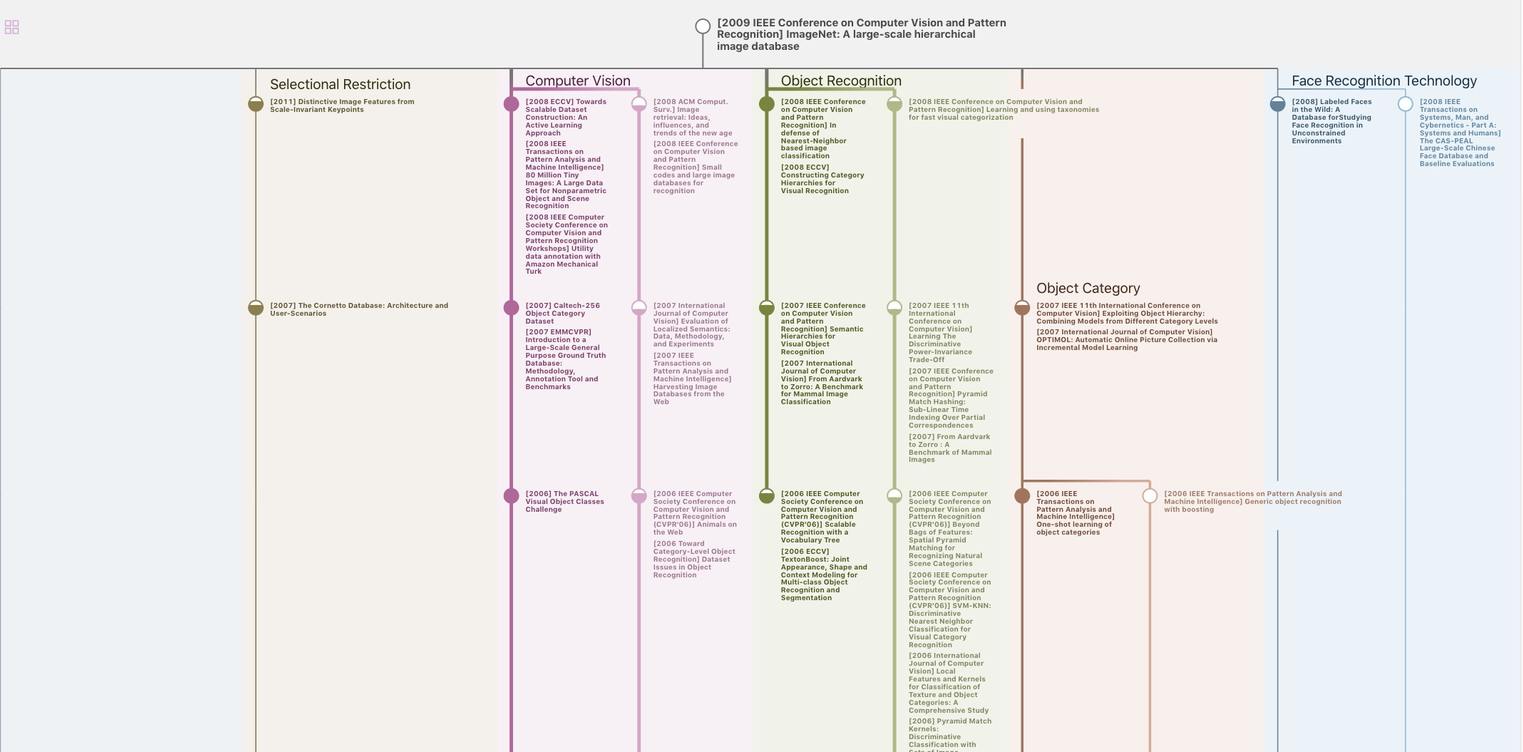
生成溯源树,研究论文发展脉络
Chat Paper
正在生成论文摘要