Query-specific Subtopic Clustering
2022 ACM/IEEE Joint Conference on Digital Libraries (JCDL)(2022)
摘要
We propose a Query-Specific Siamese Similarity Metric (QS3M) for query-specific clustering of text documents. Our approach uses fine-tuned BERT embeddings to train a non-linear projection into a query-specific similarity space. We build on the idea of Siamese networks but include a third component, a representation of the query. QS3M is able to model the fine-grained similarity between text passages about the same broad topic and also generalizes to new unseen queries during evaluation. The empirical evaluation for clustering employs two TREC datasets and a set of academic abstracts from arXiv. When used to obtain query-relevant clusters, QS3M achieves a 12% performance improvement on the TREC datasets over a strong BERT-based reference method and many baselines such as TF-IDF and topic models. A similar improvement is observed for the arXiv dataset suggesting the general applicability of QS3M to different domains. Qualitative evaluation is carried out to gain insight into the strengths and limitations of the model. CCS CONCEPTS • Information systems → Specialized information retrieval; Digital libraries and archives.
更多查看译文
关键词
clustering,topic detection,topic model,neural networks,query-specific clustering,Siamese neural networks,similarity metric
AI 理解论文
溯源树
样例
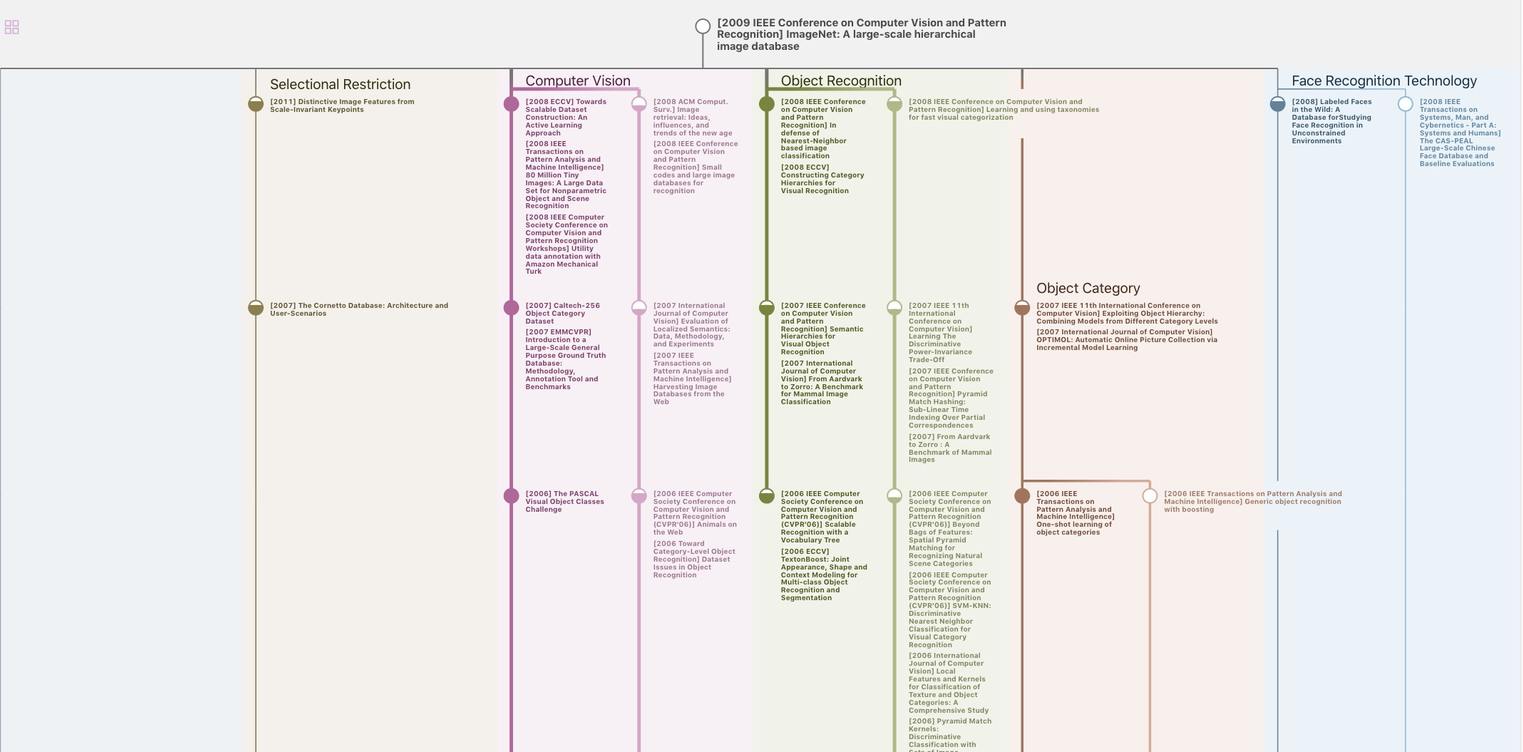
生成溯源树,研究论文发展脉络
Chat Paper
正在生成论文摘要