Leveraging Contractive Autoencoder with Fuzzy Lattice Reasoning and Resilient KNN for Detection of multi-level Bitcoin Ransomware
JOURNAL OF INFORMATION ASSURANCE AND SECURITY(2022)
摘要
In recent years, ransomware attacks have become increasingly rampant by the offenders for which ransomware has maintained a major cyber security threat as time progresses. With paradigm shift from social to technical factors, ransomware has also maintained the equal adaptiveness by shifting its focus from initial days' scareware and locker attacks to most recent crypto-ransomware threats. There is no silver bullet available to wipe out completely crypto-ransomware attacks for its obvious relationships between social engineering which investigates more infections with encrypted malware. Bitcoin, a means of digital payment demanded by Ransomware family needs characterization and analysis to predict the crypto-ransomware attack types. In this paper, at first, contractive autoencoder (CAE) is used on bitcoin transaction dataset for dimensionality reduction as a filter approach in order to obtain a reduced yet a powerful representation of the raw data and then the output of CAE is applied to the classifier for its improved performance and to make it a robust model. We use two classifiers for our experiments namely: Resilient KNN and Fuzzy Lattice Reasoning (FLR). The original KNN classifier was successful in dealing with homogenous data where the values of the numerical attribute exist completely but poses limitations while dealing with heterogeneous incomplete data containing mixed data (numeric and categorical) yet having missing values. Further, KNN used same K values for all the query objects that sometimes leads to misclassification. Resilient KNN is proposed in this paper to deal with these pitfalls effectively by assigning different k-values for different query objects, so as to obtain a most accurate predictive model. Next, the FLR is used for its ability to handle different types of data types and moreover, it is incremental and fast learning which tempted us to explore its possibility in detecting the crypto-ransomware attacks efficiently. The experimental results with several conventional and new evaluation metrics justifies the suitability of our proposed approach in building a robust and efficient classifier model to detect crypto ransomware families in comparison to existing research.
更多查看译文
关键词
bitcoin, Crypto-ransomware, cyber security, autoencoder, FLR, Resilient KNN, Likelihood ratio, Youden's Index, Net benefit, Efficiency index, Gain, detection accuracy
AI 理解论文
溯源树
样例
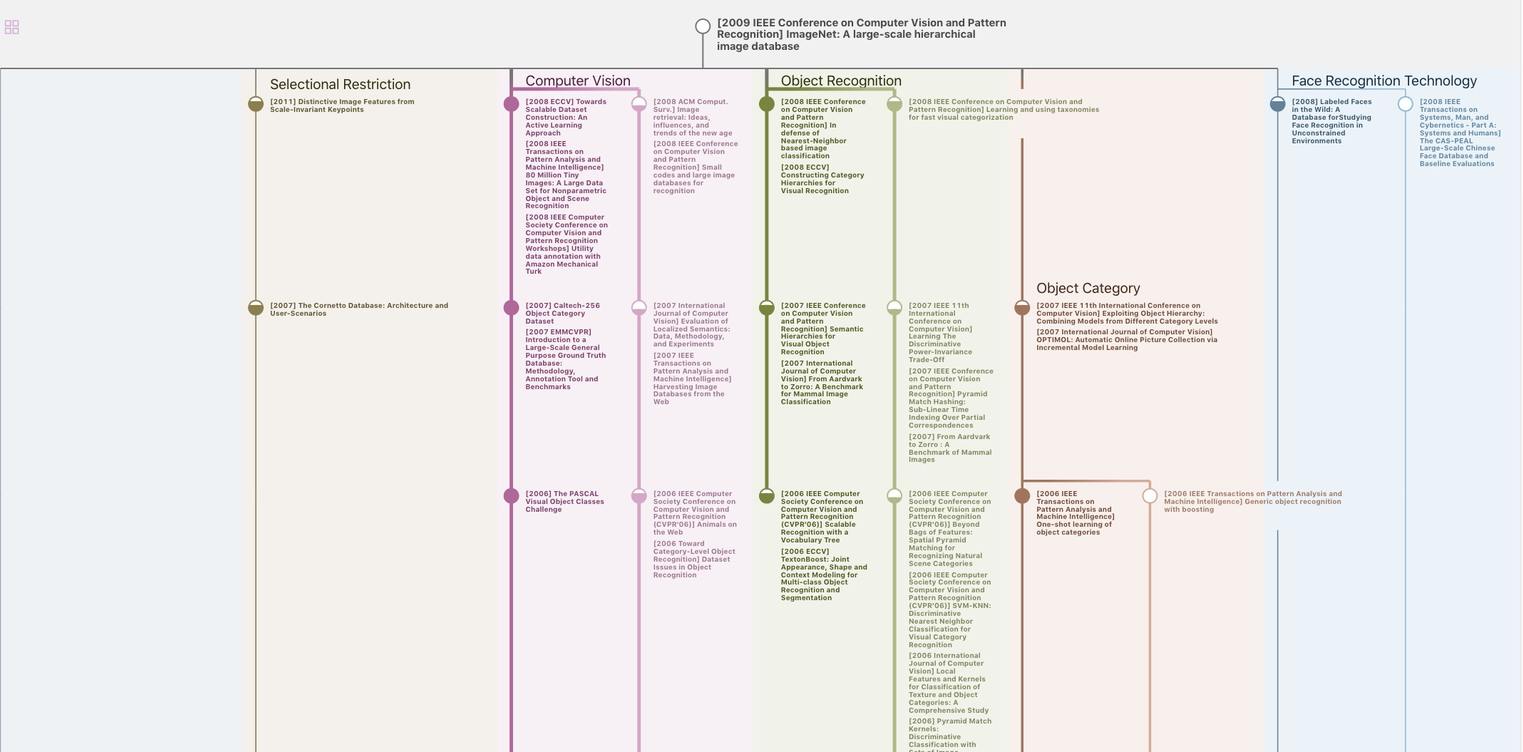
生成溯源树,研究论文发展脉络
Chat Paper
正在生成论文摘要