DFHelper: Help clients to participate in federated learning tasks
Applied Intelligence(2022)
摘要
Federated learning is widely researched due to its strength in broking data islands. In federated learning, data belonging to clients is no longer uploaded to a central server, which brings a great positive influence to privacy protection and data cooperation. However, federated learning also has higher requirements that a client should locally train a model on his own data. Some clients are unwilling to participate in federated learning due to insufficient computing resources or potential economic risks. To encourage all devices to take part in federated learning, we develop a tool DFHelper based on the combination of cloud and edge computing. First, DFHelper provides a collaborative computing service for powerless clients. Through this service, powerless clients can upload their data to nearby edge nodes according to the arrangement of cloud computing. Different edge nodes are responsible for different processes in model training, and the backup mechanism ensures the rights of powerless clients. Second, DFHelper also provides an incentive mechanism for all participating roles, including task publisher, powerful clients, powerless clients, and edge nodes. This incentive mechanism calculates the rewards based on contributions. Numerical results about collaborative computing and incentive mechanism show that DFHelper can relieve the computing pressure of powerless clients and bring reasonable rewards for all roles in federated learning.
更多查看译文
关键词
Federated learning, Edge computing, Incentive mechanism, Collaborative computing, Cloud computing
AI 理解论文
溯源树
样例
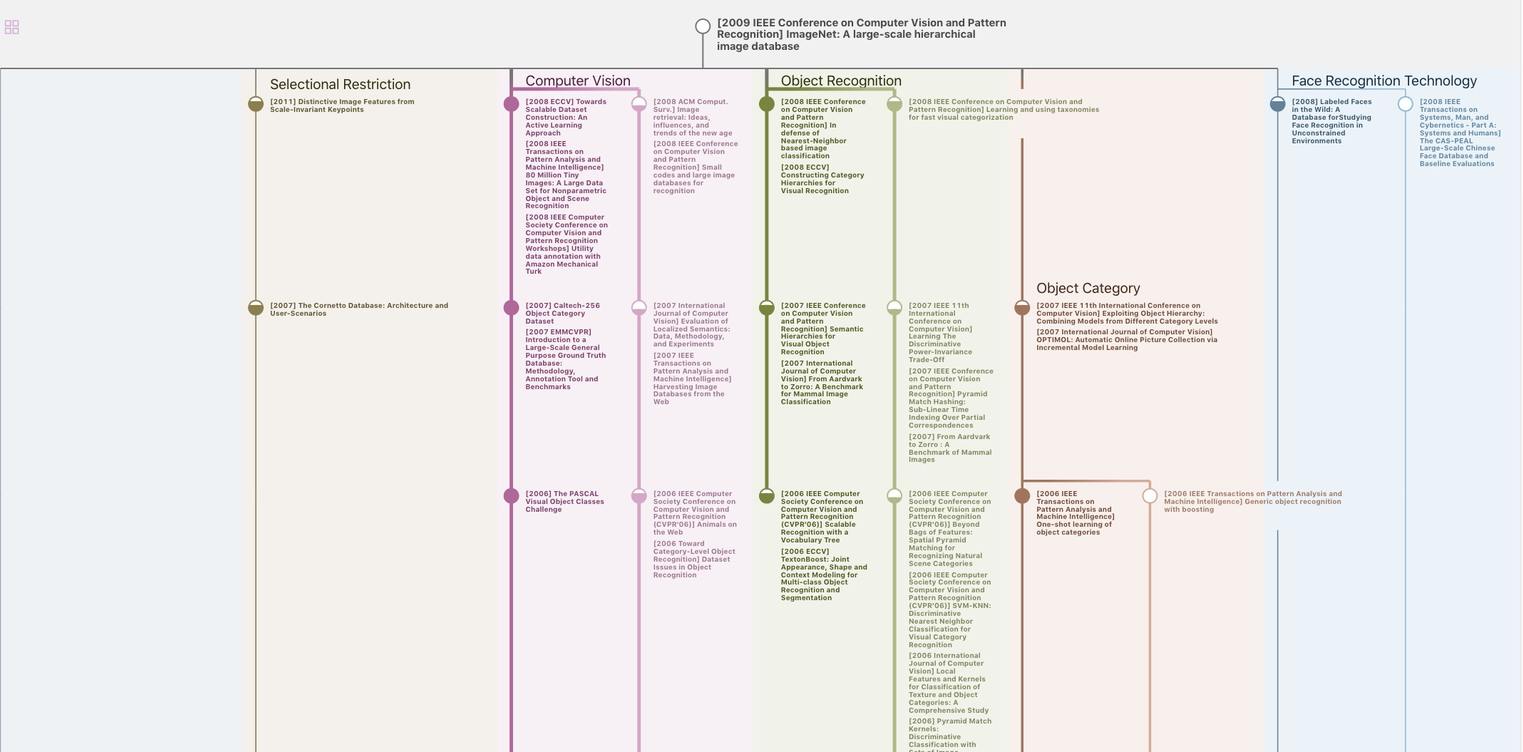
生成溯源树,研究论文发展脉络
Chat Paper
正在生成论文摘要