Cover-based multiple book genre recognition using an improved multimodal network
INTERNATIONAL JOURNAL ON DOCUMENT ANALYSIS AND RECOGNITION(2022)
摘要
Despite the idiom not to prejudge something by its outward appearance, we consider deep learning to learn whether we can judge a book by its cover or, more precisely, by its text and design. The classification was accomplished using three strategies, i.e., text only, image only, and both text and image. State-of-the-art CNNs (convolutional neural networks) models were used to classify books through cover images. The Gram and SE layers (squeeze and excitation) were used as an attention unit in them to learn the optimal features and identify characteristics from the cover image. The Gram layer enabled more accurate multi-genre classification than the SE layer. The text-based classification was done using word-based, character-based, and feature engineering-based models. We designed EXplicit interActive Network (EXAN) composed of context-relevant layers and multi-level attention layers to learn features from books title. We designed an improved multimodal fusion architecture for multimodal classification that uses an attention mechanism between modalities. The disparity in modalities convergence speed is addressed by pre-training each sub-network independently prior to end-to-end training of the model. Two book cover datasets were used in this study. Results demonstrated that text-based classifiers are superior to image-based classifiers. The proposed multimodal network outperformed all models for this task with the highest accuracy of 69.09 % and 38.12% for Latin and Arabic book cover datasets. Similarly, the proposed EXAN surpassed the extant text classification models by scoring the highest prediction rates of 65.20% and 33.8% for Latin and Arabic book cover datasets.
更多查看译文
关键词
Book covers classification, CNN, Image classifiers, Multimodal learning, Text classifiers
AI 理解论文
溯源树
样例
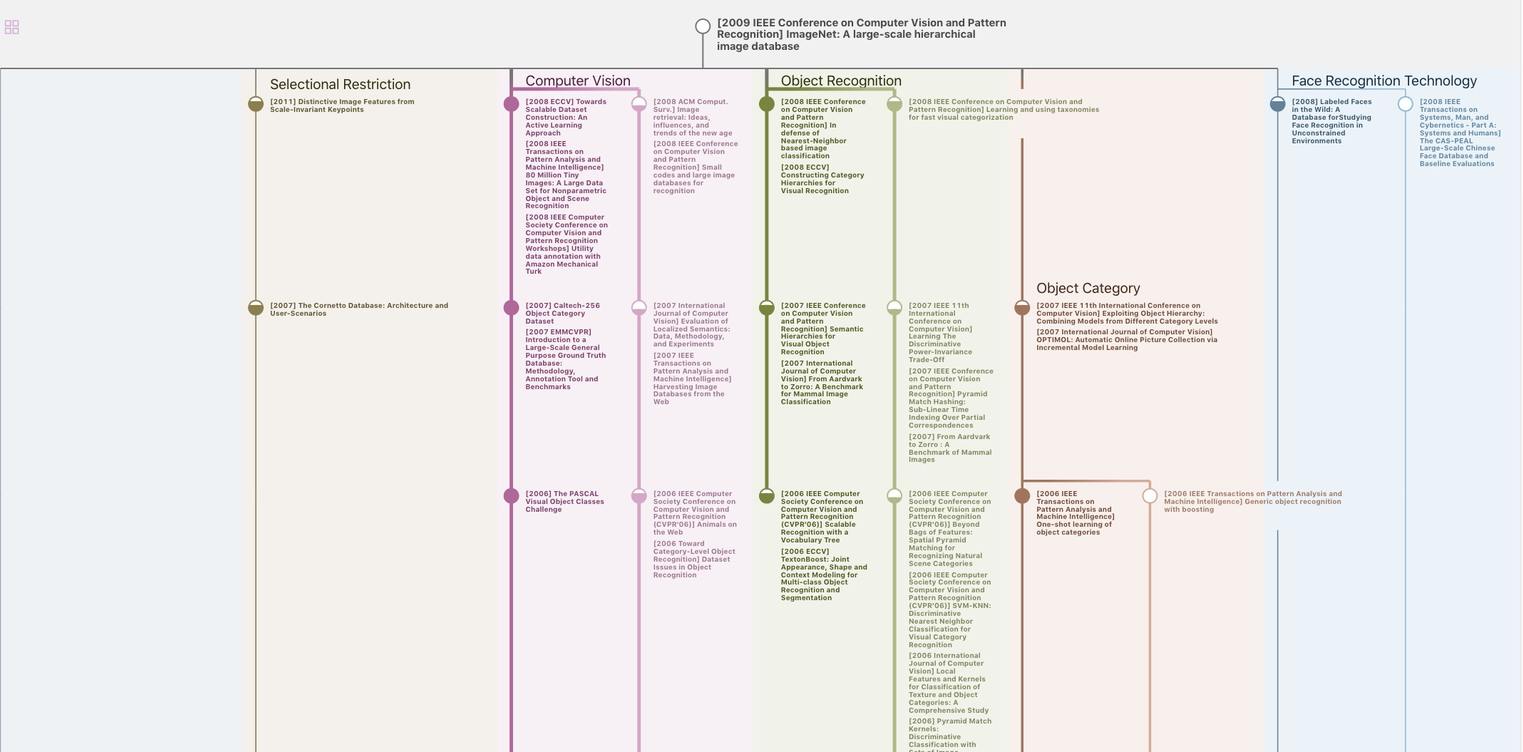
生成溯源树,研究论文发展脉络
Chat Paper
正在生成论文摘要