Saliency Transfer Learning and Central-Cropping Network for Prostate Cancer Classification
NEURAL PROCESSING LETTERS(2022)
摘要
Classifying the malignancy of prostate lesions from MRI images is crucial in diagnosing prostate cancer at the early stage. In clinical examination, radiologists usually focus on the most salient and distinctive regions to diagnose. However, in many state-of-the-art CNN based methods, the conventional convolution operation extracts the features equally importantly, which leads to an excessive feature learning process on the uninterested regions. To address this challenge, we propose a saliency transfer learning network that allows the model to focus on the salient and influential regions automatically. Moreover, a pyramid central-crop pooling scheme is employed to extract the multi-scale, centric-visual, and salient features from different layers. To validate the effectiveness of the proposed model, extensive experiments are conducted on prostate cancer and non-cancer MRI dataset, the experimental results demonstrate that our proposed model could gain competitive performance (Accuracy 94.9%, Sensitivity 96.7%, Specificity 93.5%, AUC 0.989) on this classification task.
更多查看译文
关键词
Prostate cancer,Saliency transfer learning,Feature pyramid,Central-crop pooling
AI 理解论文
溯源树
样例
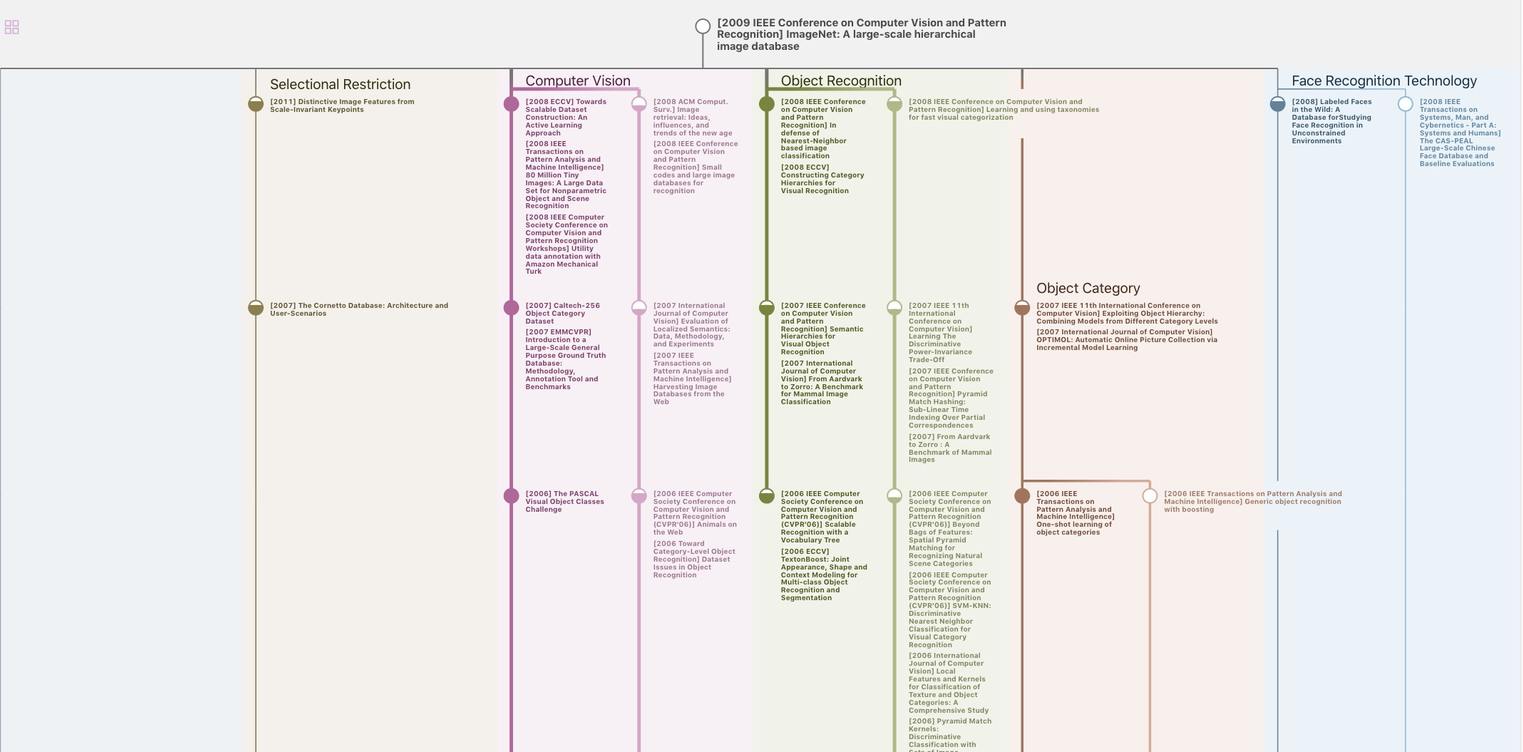
生成溯源树,研究论文发展脉络
Chat Paper
正在生成论文摘要