A triple fusion model for cross-modal deep hashing retrieval
MULTIMEDIA SYSTEMS(2022)
摘要
In the field of resource retrieval, deep cross-modal retrieval is attracting increasing attention. It has a lower storage capacity and faster retrieval speed. However, most of the current methods put their attention on the semantic similarity between hash codes. They ignore the similarity between features extracted by the model from different modalities, which leads them to achieve sub-optimal results. In addition, the correlation between different modalities is difficult to exploit adequately. Therefore, in order to enhance the information correlation between different modalities, a triple fusion model for cross-modal deep hashing retrieval (SSTFH) is proposed in this paper. To weaken the missing feature information when features pass through the fully connected layer, we designed a triple fusion strategy. Specifically, the first fusion and the second fusion are performed for images and text respectively, to obtain pattern-specific features. The third fusion is used to obtain more relevant semantic features. In addition, we attempt to use shared semantic information from semantic features to guide the model in extracting correlations between different modalities. Comprehensive experiments have been conducted on the benchmark IAPR TC-12 and MS COCO datasets. On MS COCO, our approach outperforms all the deep baselines by an average of 7.74 % on the image-to-text task, and by 8.72 % on the text-to-image task. On IAPR TC-12, our approach averagely improves image retrieval by 7.07 % and text retrieval by 4.88 % .
更多查看译文
关键词
Hashing learning, Cross-modal retrieval, Semantic similarity, Shared semantics
AI 理解论文
溯源树
样例
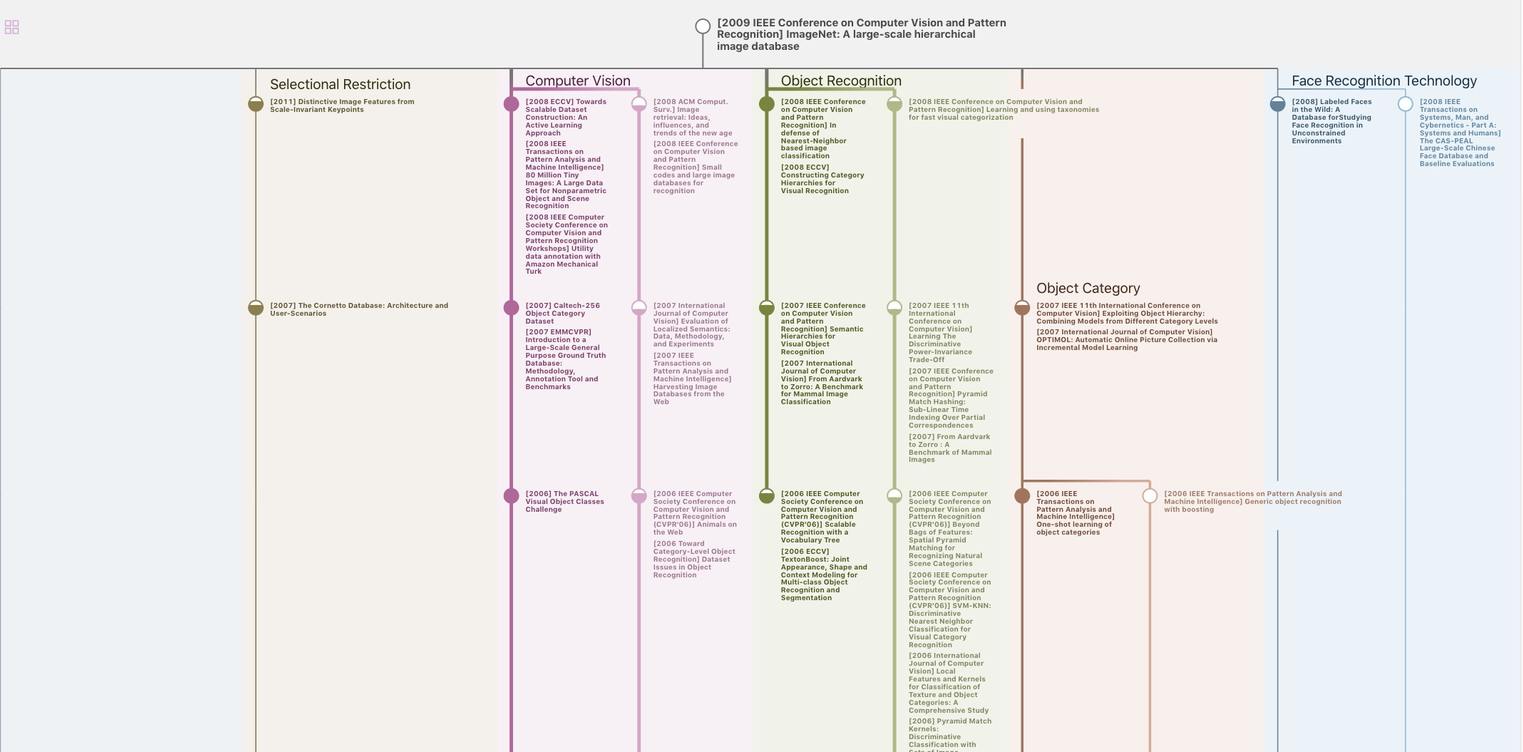
生成溯源树,研究论文发展脉络
Chat Paper
正在生成论文摘要