Comparison between model-based and data-based methods for fault diagnosis of railway turnouts*
2022 8TH INTERNATIONAL CONFERENCE ON CONTROL, DECISION AND INFORMATION TECHNOLOGIES (CODIT'22)(2022)
摘要
Railway switch machines are important elements in a railway network, allowing trains to change directions. Any failure in such safety critical equipment can induce economic losses caused by traffic interruptions and even sometimes catastrophic accidents. Hence, the diagnosis of such failures is recognized to be a primordial task. In the consulted literature, the methods considered are those based on an analytical model or those based on data (artificial intelligence) or hybrid with suitable justifications. The present paper deals with synthesis and discussion of two approaches applied to railway switch machine: Linear Fractional Transformation (LFT) Bond Graph as multiphysic model based robust diagnosis and a data driven method based on k nearest neighbor (kNN) algorithm. A simulation platform is developed to test those two algorithms under various scenarios of faults that may affect the switch machine. Results show that the LFT Bond Graph model based diagnosis whose performances depend mainly on model accuracy is robust with respect to parameters uncertainties and able to detect and isolate the majority of faults, including sensor, plant and actuator faults, while for classification-based diagnosis, even if a good accuracy is achieved with any need of complex equations, a great amount of computational time is devoted to parameters tuning.
更多查看译文
关键词
actuator faults,artificial intelligence,classification-based diagnosis,data-based method,data-driven method,fault diagnosis,k-nearest neighbor algorithm,LFT bond graph model based diagnosis,linear fractional transformation bond graph model based diagnosis,model-based method,railway network,railway switch machine,railway turnouts,safety critical equipment
AI 理解论文
溯源树
样例
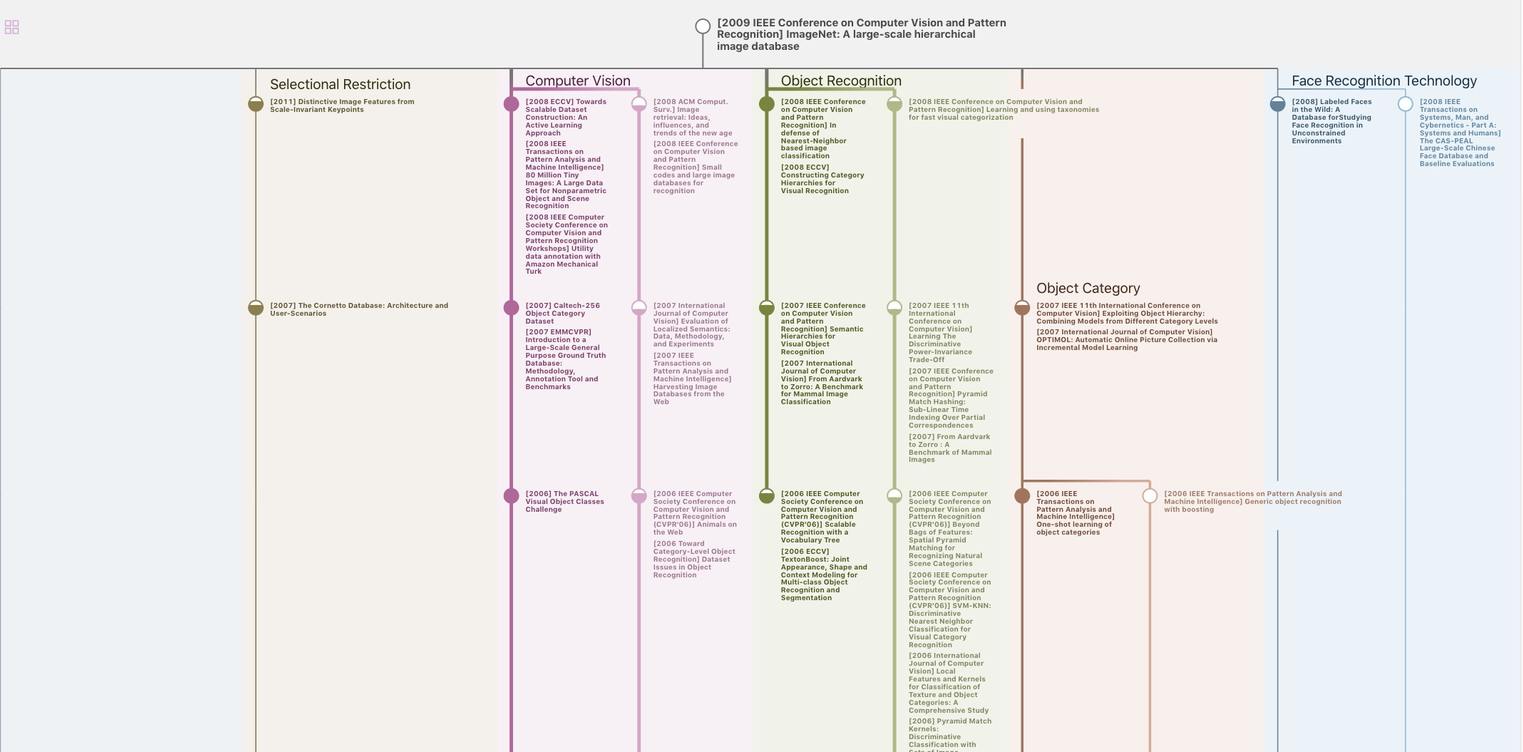
生成溯源树,研究论文发展脉络
Chat Paper
正在生成论文摘要