Learning-based compression of visual objects for smart surveillance
2022 Eleventh International Conference on Image Processing Theory, Tools and Applications (IPTA)(2022)
摘要
Advanced video applications in smart environments (e.g., smart cities) bring different challenges associated with increasingly intelligent systems and demanding requirements in emerging fields such as urban surveillance, computer vision in industry, medicine and others. As a consequence, a huge amount of visual data is captured to be analyzed by task-algorithm driven machines. In this context, this paper proposes an efficient learning-based approach to compress relevant visual objects, captured in surveillance contexts and delivered for machine vision processing. An object-based compression scheme is devised, comprising multiple autoencoders, each one optimised to produce an efficient latent representation of a corresponding object class. The performance of the proposed approach is evaluated with two types of visual objects: persons and faces and two task-algorithms: class identification and object recognition, besides traditional image quality metrics like PSNR and VMAF. In comparison with the Versatile Video Coding (VVC) standard, the proposed approach achieves significantly better coding efficiency than the VVC, e.g., up to 46.7% BD-rate reduction. The accuracy of the machine vision tasks is also significantly higher when performed over visual objects compressed with the proposed scheme in comparison with the same tasks performed over the same visual objects compressed with the VVC. These results demonstrate that the learning-based approach proposed in this paper is a more efficient solution for compression of visual objects than standard encoding.
更多查看译文
关键词
Learning-based compression,autoencoders,visual objects,video surveillance
AI 理解论文
溯源树
样例
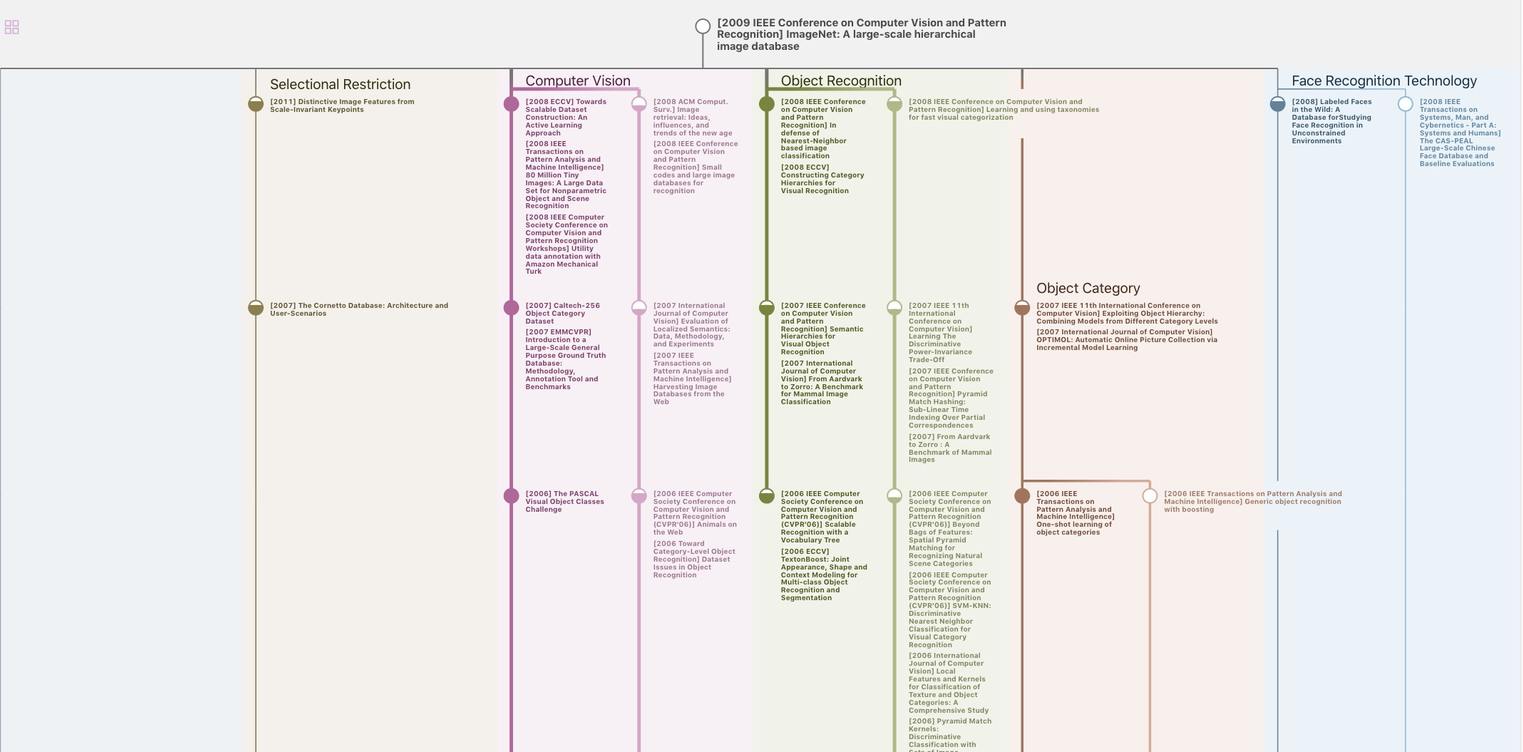
生成溯源树,研究论文发展脉络
Chat Paper
正在生成论文摘要