Hybrid regularization model combining overlapping group sparse second-order total variation and nonconvex total variation
JOURNAL OF ELECTRONIC IMAGING(2022)
摘要
Restoring the original image from an observed noisy or blurred version is a very important issue in image processing. The variational regularization methods obtained by functional minimizing are popular and widely used for image restoration with promising accuracy, but they may suffer from limited performances due to the presence of the conflict between noise-and-blur-removing and detail-and-texture-preserving. To solve this problem, a hybrid variational regularization model is proposed to effectively reconstruct image textures and details while eliminating noise and blur. The proposed model uses an overlapping group of sparse second-order total variation (TV) as the regularizer to restore image textures and details, whereas the nonconvex TV serves as the other regularizer to preserve image edges and contours. An alternating directional multiplier method algorithm is developed to numerically solve the proposed model, where the majorization-minimization and the iterative reweighted l(1) (IRL1) algorithm are used to solve the overlapping group and the nonconvex minimization subproblem, respectively. The numerical experiments validate the proposed model and algorithm. In addition, compared with several state-of-the-art image restoration models, the proposed model shows the competitive performance in terms of PSNR, SSIM, and NIQE values.
更多查看译文
关键词
image restoration, hybrid regularization, overlapping group sparsity, nonconvex
AI 理解论文
溯源树
样例
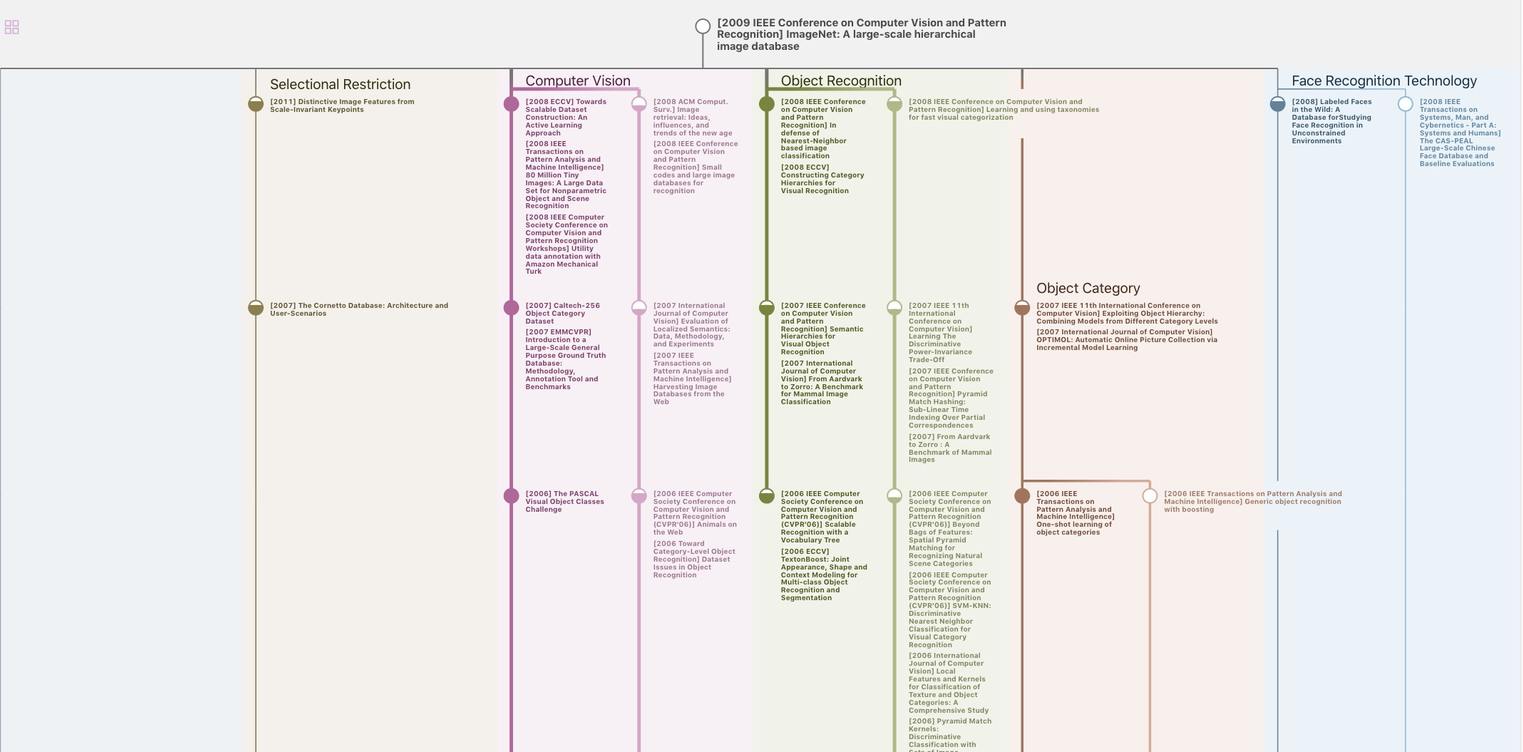
生成溯源树,研究论文发展脉络
Chat Paper
正在生成论文摘要