Vision-Based Sensing for Electrically-Driven Soft Actuators
IEEE ROBOTICS AND AUTOMATION LETTERS(2022)
Abstract
Developing reliable control strategies in soft robotics requires advances in soft robot perception. However, current soft robotic sensors pose many performance limitations, and available materials and manufacturing techniques complicate soft sensorized robot design. To address these long-standing needs, we introduce a method for using vision to sensorize robust, electrically-driven soft robotic actuators constructed from a new class of architected materials. Specifically, we use cameras positioned within the hollow interiors of handed shearing auxetic (HSA) actuators to record deformation during motion. We train a convolutional neural network (CNN) that maps the visual feedback to the actuator's tip pose. Our model provides predictions with sub-millimeter accuracy from only six minutes of training data, while remaining lightweight with an inference time of 18 milliseconds per frame. We also develop a model that additionally predicts the horizontal tip force acting on the actuator and generalizes to previously unseen forces. Finally, we demonstrate the viability of our sensorization strategy for contact-rich applications by training a CNN that predicts the tip pose accurately during tactile interactions. Overall, our methods present a reliable vision-based approach for designing sensorized soft robots built from electrically-actuated, architected materials.
MoreTranslated text
Key words
Deep learning for visual perception,force and tactile sensing,modeling,control,and learning for soft robots,perception for grasping and manipulation,soft sensors and actuators
AI Read Science
Must-Reading Tree
Example
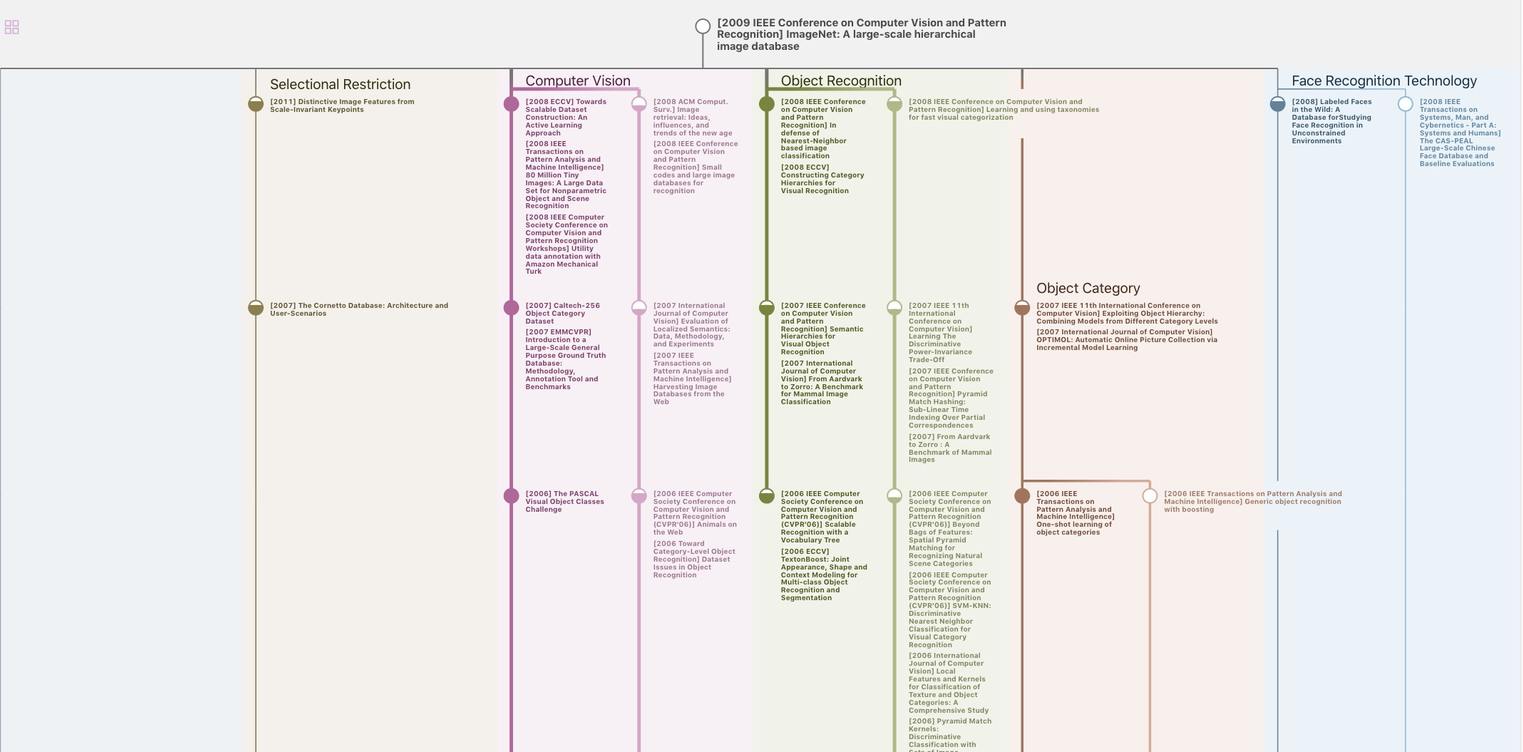
Generate MRT to find the research sequence of this paper
Chat Paper
Summary is being generated by the instructions you defined