ECG Signal Features Classification for the Mental Fatigue Recognition
MATHEMATICS(2022)
Abstract
Mental fatigue is a major public health issue worldwide that is common among both healthy and sick people. In the literature, various modern technologies, together with artificial intelligence techniques, have been proposed. Most techniques consider complex biosignals, such as electroencephalogram, electro-oculogram or classification of basic heart rate variability parameters. Additionally, most studies focus on a particular area, such as driving, surgery, etc. In this paper, a novel approach is presented that combines electrocardiogram (ECG) signal feature extraction, principal component analysis (PCA), and classification using machine learning algorithms. With the aim of daily mental fatigue recognition, an experiment was designed wherein ECG signals were recorded twice a day: in the morning, i.e., a state without fatigue, and in the evening, i.e., a fatigued state. PCA analysis results show that ECG signal parameters, such as Q and R wave amplitude values, as well as QT and T intervals, presented with the largest differences between states compared to other ECG signal parameters. Furthermore, the random forest classifier achieved more than 94.5% accuracy. This work demonstrates the feasibility of ECG signal feature extraction for automatic mental fatigue detection.
MoreTranslated text
Key words
machine learning,ECG,mental fatigue,signal analysis,classification
AI Read Science
Must-Reading Tree
Example
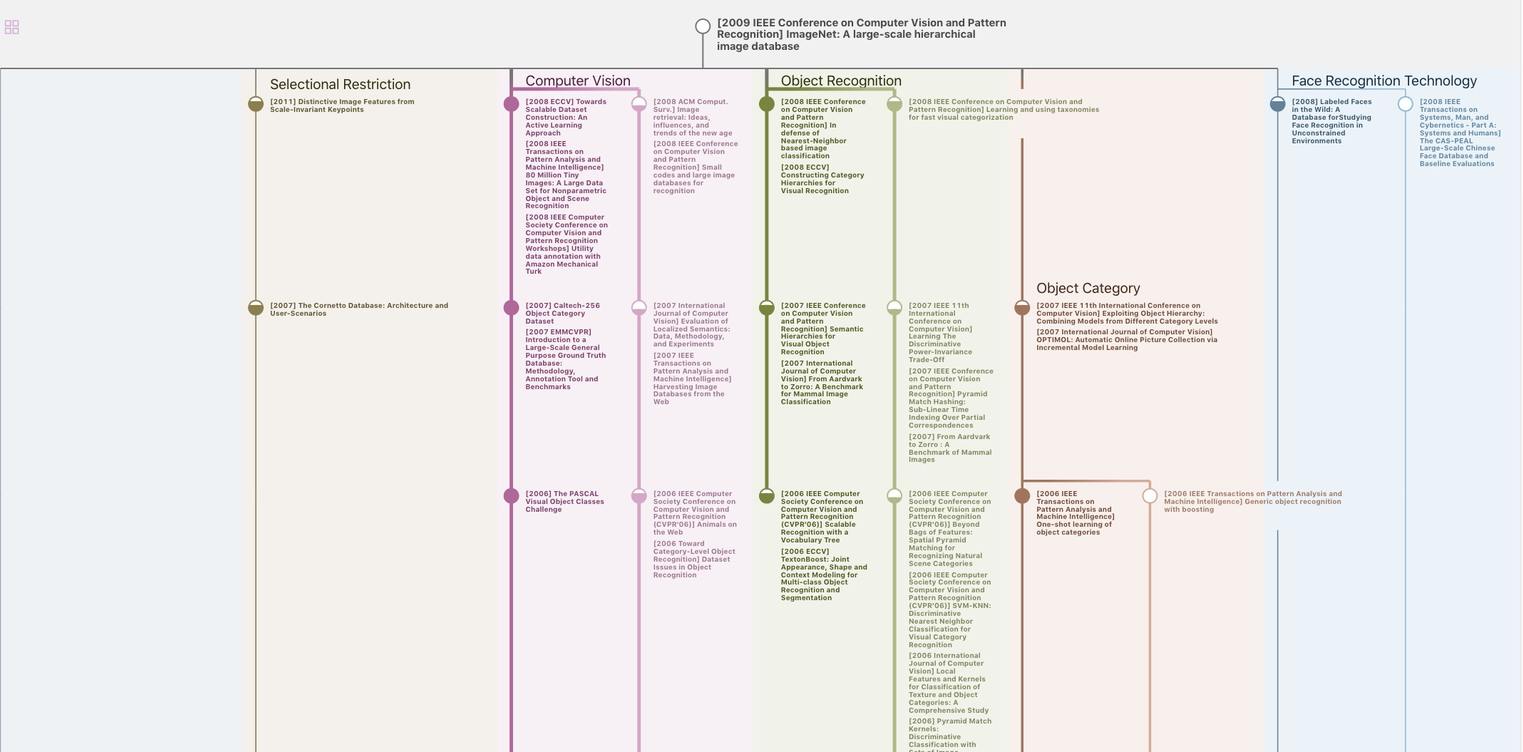
Generate MRT to find the research sequence of this paper
Chat Paper
Summary is being generated by the instructions you defined