A novel multivariate time series combination prediction model
COMMUNICATIONS IN STATISTICS-THEORY AND METHODS(2024)
Abstract
This article presents a novel multivariate time series prediction model based on combination model. The input variables of multivariate time series are coupled and correlated. Firstly, kernel principal component analysis is used to preprocess the original multidimensional input variables to determine the principal components that affect the output. Extracting principal components can reduce the complexity of modeling. Then, an improved grey wolf optimization algorithm optimized echo state network is introduced as the prediction model for principal components. Finally, two typical multivariate time series data, air quality index and stock market price, are taken as research objects to verify the performance of the proposed combination prediction model. Compared with other state-of-the-art prediction models, the results clearly reveal that the proposed prediction model is superior to all the considered models herein in terms of both prediction accuracy and performance indicators. As a result, it is concluded that the proposed prediction model can be an efficient and effective technique for multivariate time series prediction.
MoreTranslated text
Key words
Multivariate time series,combination prediction model,kernel principal component analysis,echo state network,improved grey wolf algorithm
AI Read Science
Must-Reading Tree
Example
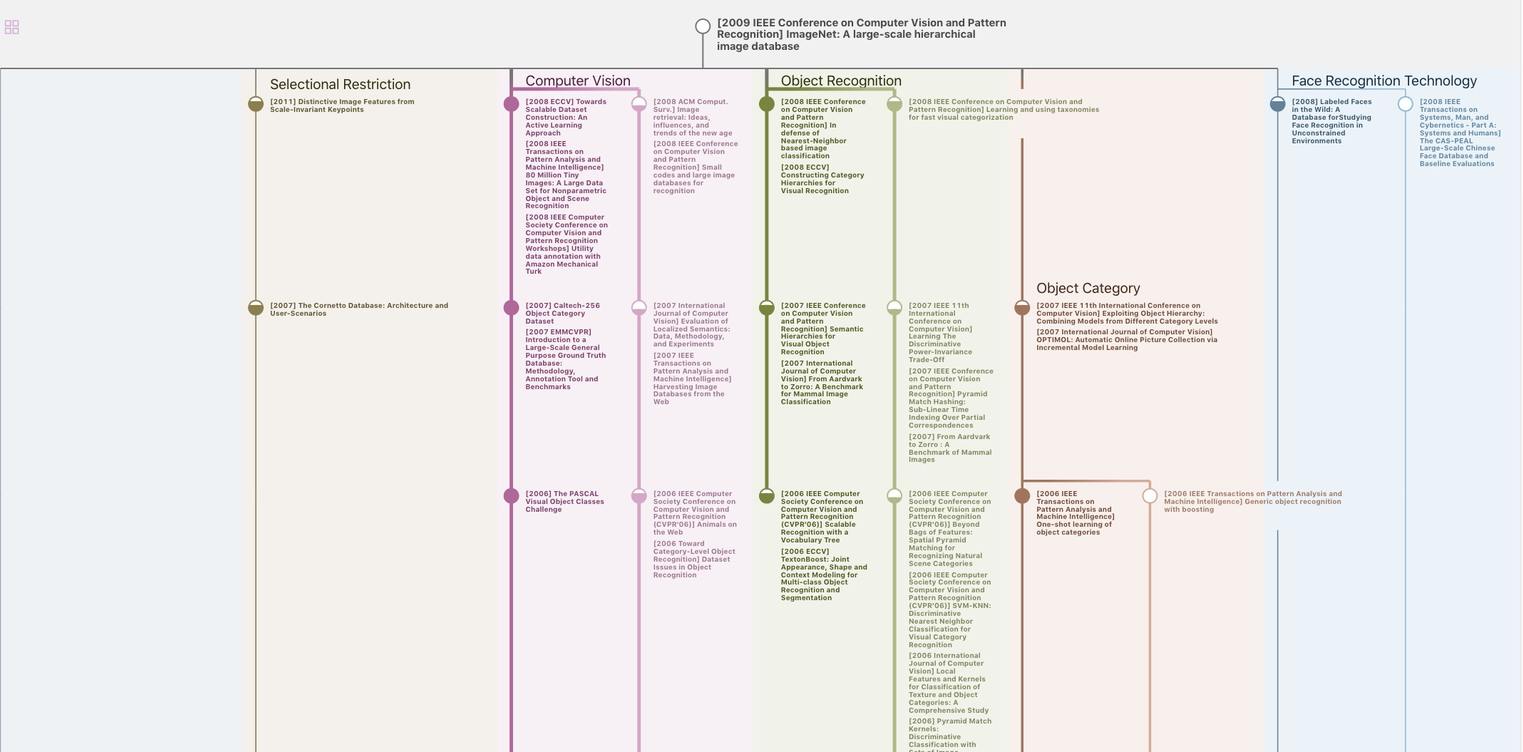
Generate MRT to find the research sequence of this paper
Chat Paper
Summary is being generated by the instructions you defined