Predicting soil organic matter and soil moisture content from digital camera images: comparison of regression and machine learning approaches
CANADIAN JOURNAL OF SOIL SCIENCE(2022)
Abstract
Appropriate soil management maintains and improves the health of the entire ecosystem. Soil appropriate administration necessitates proper characterization of its properties including soil organic matter (SOM) and soil moisture content (SMC). Image-based soil characterization has shown strong potential in comparison with traditional methods. This study compared the performance of 22 different supervised regression and machine learning algorithms, including support vector machines (SVMs), Gaussian process regression (GPR) models, ensembles of trees, and artificial neural network (ANN), in predicting SOM and SMC from soil images taken with a digital camera in the laboratory setting. A total of 22 image parameters were extracted and used as predictor variables in the models in two steps. First models were developed using all 22 extracted features and then using a subset of six best features for both SOM and SMC. Saturation index (redness index) was the most important variable for SOM prediction, and contrast (median S) for SMC prediction, respectively. The color and textural parameters demonstrated a high correlation with both SOM and SMC. Results revealed a satisfactory agreement between the image parameters and the laboratory-measured SOM (R-2 and root mean square error (RMSE) of 0.74 and 9.80% using cubist) and SMC (R-2 and RMSE of 0.86 and 8.79% using random forest) for the validation data set using six predictor variables. Overall, GPR models and tree models (cubist, RF, and boosted trees) best captured and explained the nonlinear relationships between SOM, SMC, and image parameters for this study.
MoreTranslated text
Key words
digital camera images, image color and texture features, cubist, random forest, soil characterization, computer vision
AI Read Science
Must-Reading Tree
Example
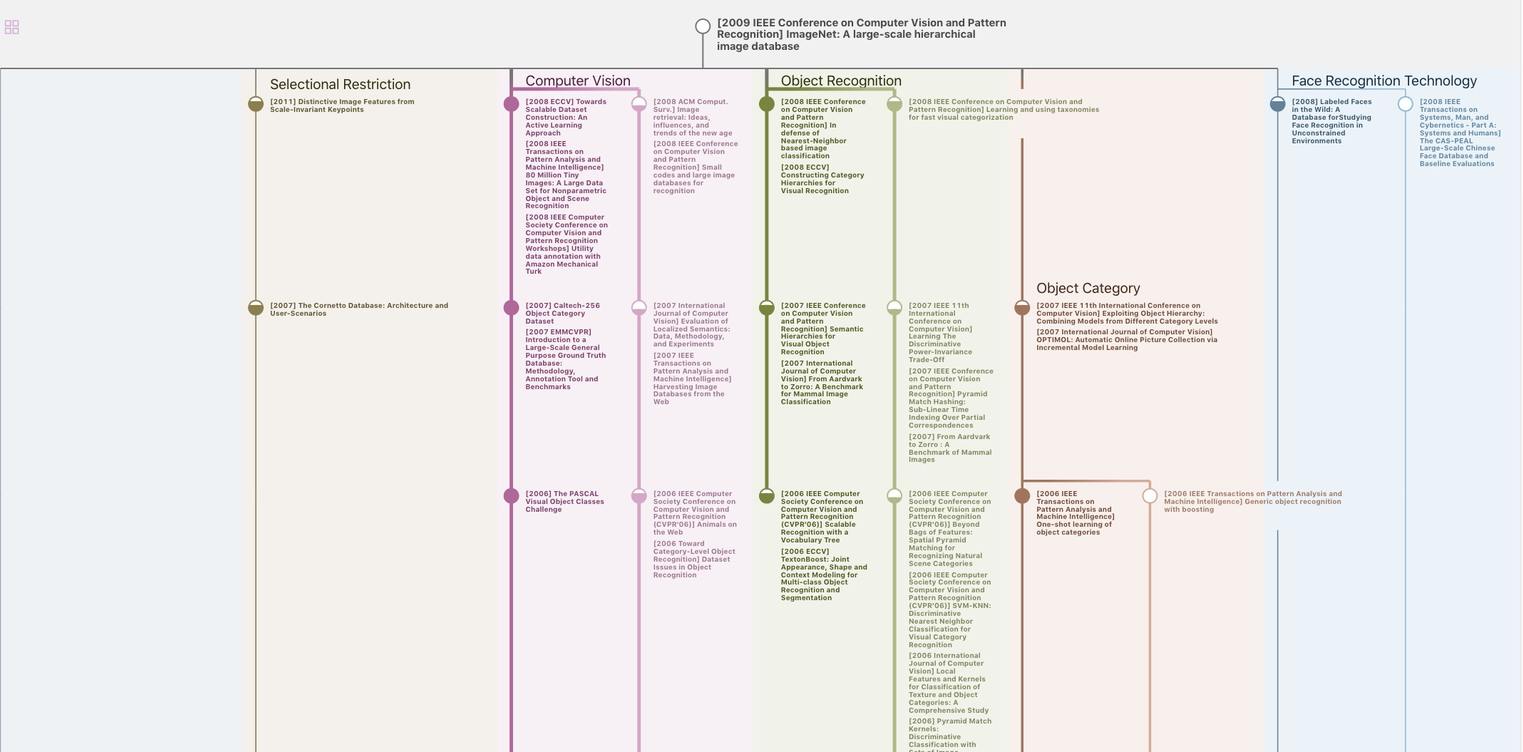
Generate MRT to find the research sequence of this paper
Chat Paper
Summary is being generated by the instructions you defined