Hyperspectral image classification with localized spectral filtering-based graph attention network
XXIV ISPRS CONGRESS: IMAGING TODAY, FORESEEING TOMORROW, COMMISSION III(2022)
摘要
Graph-based deep learning has been proved a promising approach that has an apparent superiority for learning graph data and modeling spatial topological relations between features. In particular, graph attention networks (GATs) are good at efficiently processing the graph-structured hyperspectral data by leveraging masked self-attention layers to address the known shortcomings of previous frameworks based on graph convolutions or their approximations. In this study, we proposed a novel approach that combines localized spectral filtering and GAT for the hyperspectral image classification task. First, we conducted unsupervised tSNE (t-distributed stochastic neighbor embedding) manifold learning-based feature dimensionality reduction to create localized hyperspectral data cubes. Then, these feature cubes combined with localized adjacent matrices were fed into a shallow graph attention network in a supervised learning manner. Finally, we obtained credible classification results and promising classification performance in distinguishing diversified land covers through reducing the possible redundancy of spectral information and enhancing the expression of local spatial-spectral information. Experiments on two real hyperspectral data sets (that is, Indian PinesA (IA) and Huanghekou (HH) data sets) demonstrated that the presented approach offers promising classification performance, that is, the GAT using t-SNE acquires superior performance than that of using PCA (principal component analysis), and also proves the great importance of combining spatial- and spectral information for hyperspectral image classification.
更多查看译文
关键词
Hyperspectral image classification,Graph attention network,Self-attention,Localized spectral filtering,Graphbased deep learning
AI 理解论文
溯源树
样例
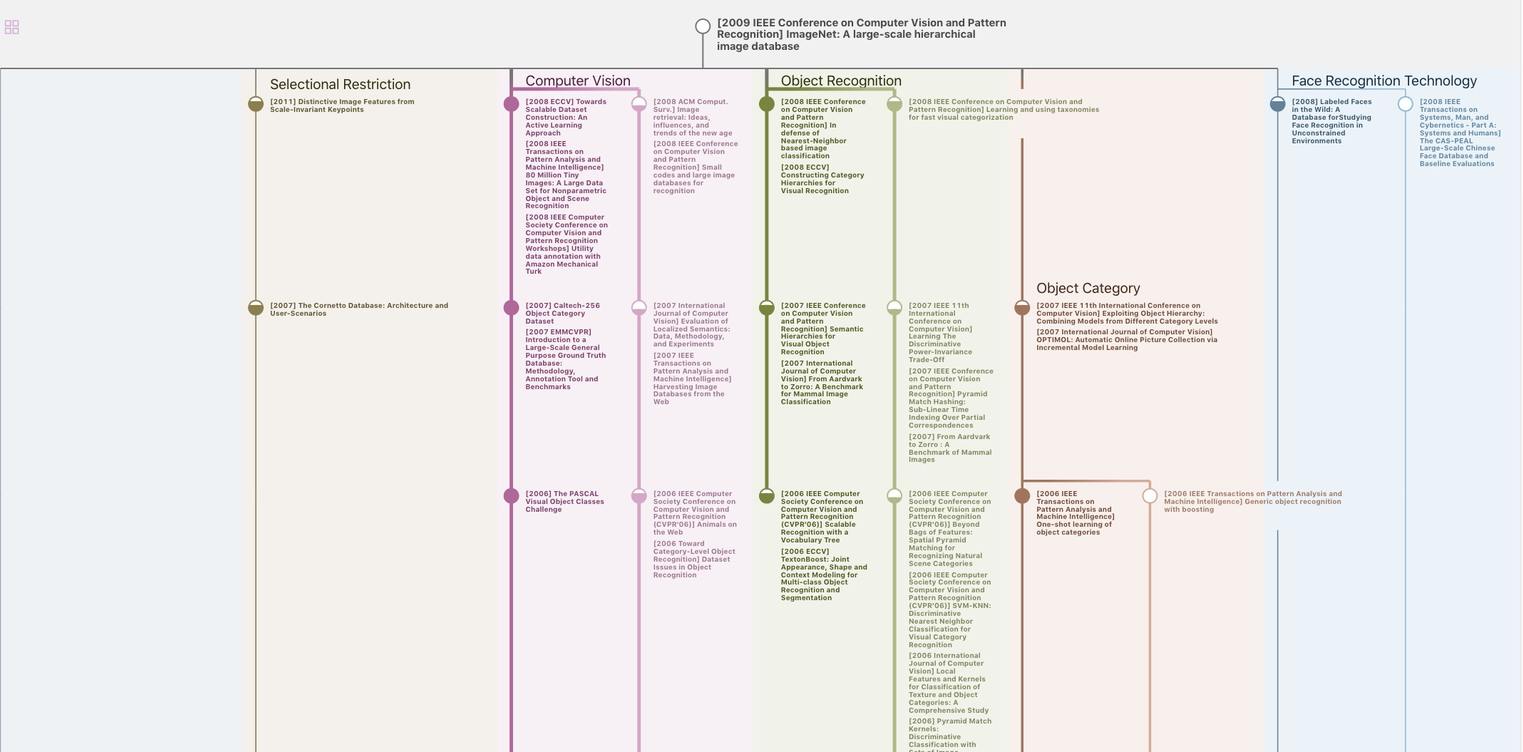
生成溯源树,研究论文发展脉络
Chat Paper
正在生成论文摘要