An improved two-stream compression convolution network for rolling bearing fault diagnosis
MEASUREMENT SCIENCE AND TECHNOLOGY(2022)
摘要
The timely and accurate fault diagnosis of rolling bearings plays a vital role in ensuring the safe and reliable operation of many complex mechanical systems. However, most of the existing rolling bearing fault diagnosis models have complex structures, need a large number of samples, and cannot fully use the information contained in the signal. Based on these problems, an intelligent method for detecting and identifying rolling bearing faults is proposed based on an improved two-stream compression convolution network. The proposed method has a compact structure and powerful feature extraction capability, which consists of two modules. One adopts depthwise separable convolution and squeeze and excitation network, named DSCSE, which can fully extract the spatial features and greatly reduce the number of parameters. The other utilizes a one-dimensional convolutional neural network and spatial dropout mechanism, named 1DCNNSD, which can efficiently extract the temporal features and reduce model complexity. Meanwhile, to reduce the loss of information contained in the signal when the linear rectification unit is under negative input, the improved ReLU is designed. Numerous experiments demonstrate that the novel approach has higher accuracy, better generalization performance, and robustness than other methods under small samples.
更多查看译文
关键词
fault diagnosis,depthwise separable convolutional network,squeeze and excitation network,feature extraction
AI 理解论文
溯源树
样例
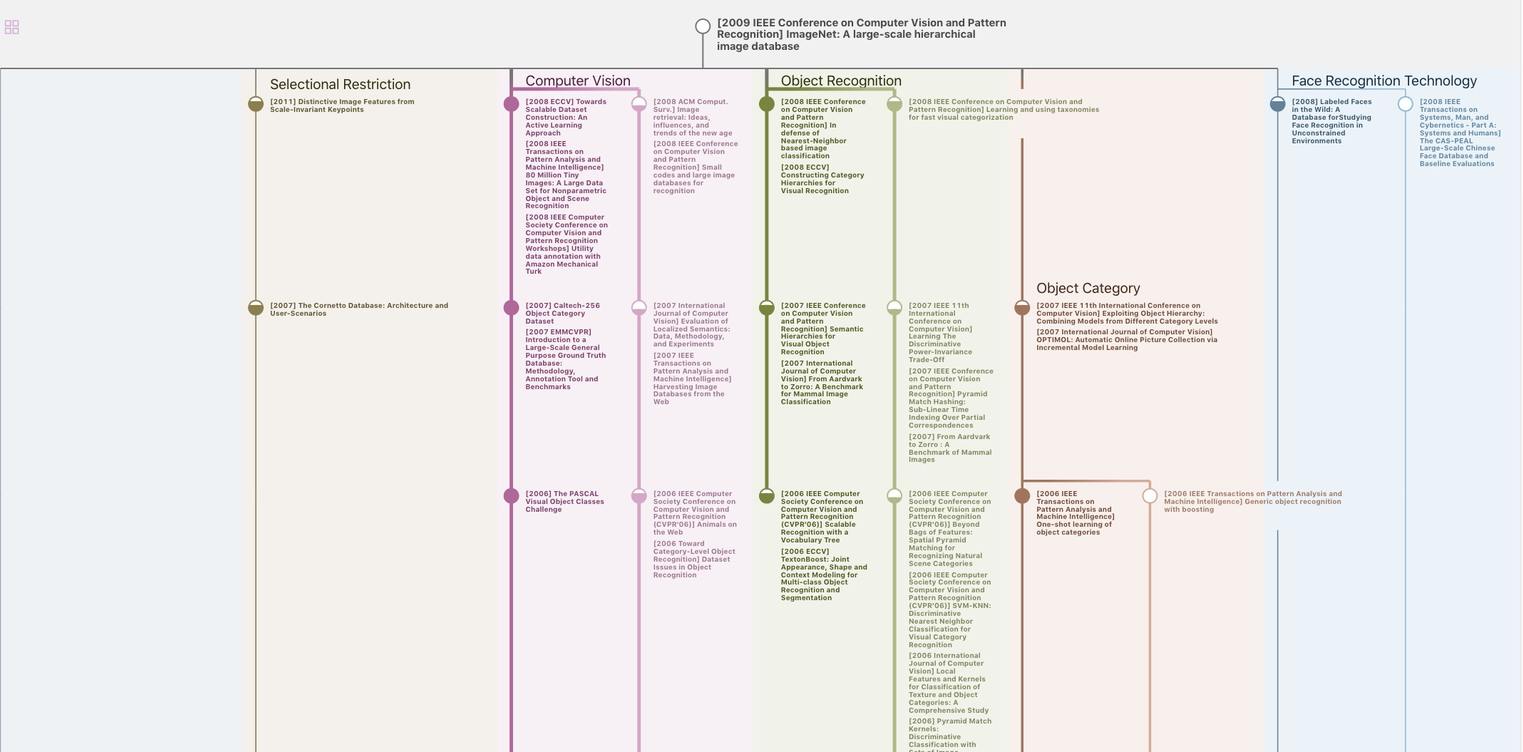
生成溯源树,研究论文发展脉络
Chat Paper
正在生成论文摘要