EXTRACTING RELEVANCE FROM SAR TEMPORAL PROFILES ON A GLACIER AND AN ALPINE WATERSHED BY A DEEP AUTOENCODER
XXIV ISPRS CONGRESS: IMAGING TODAY, FORESEEING TOMORROW, COMMISSION III(2022)
摘要
This paper proposes to use methods for compressing the temporal profiles of Sentinel-1 images, in order to be able to evaluate and analyze the richness of the temporal dynamics, both on a glacier and on a watershed. We propose to use unsupervised deep learning to auto-encode the temporal information in 3 dimensions, allowing to use the three descriptors as three RGB components to produce a colored composition synthesizing the information. We compare this Convolutional AutoEncoder (CAE) approach with a dimensionality reduction based on a Principal Component Analysis (PCA) of the temporal profiles. The two methods, CAE and PCA, are applied to a time series over the Kyagar Glacier before and after a surge event, and on an alpine watershed to compare the differences in dynamic evolution associated with different terrain classes with and without snow. On the one hand, on the glacier, the stacks of 10 images used are too short for CAE to extract more than two really significant axes. On the other hand, with longer profiles available over the alpine watershed, the CAE is interesting to improve the clustering results obtained from the decomposition.
更多查看译文
关键词
deep learning, autoencoder, glacier, visualization, surge, clustering
AI 理解论文
溯源树
样例
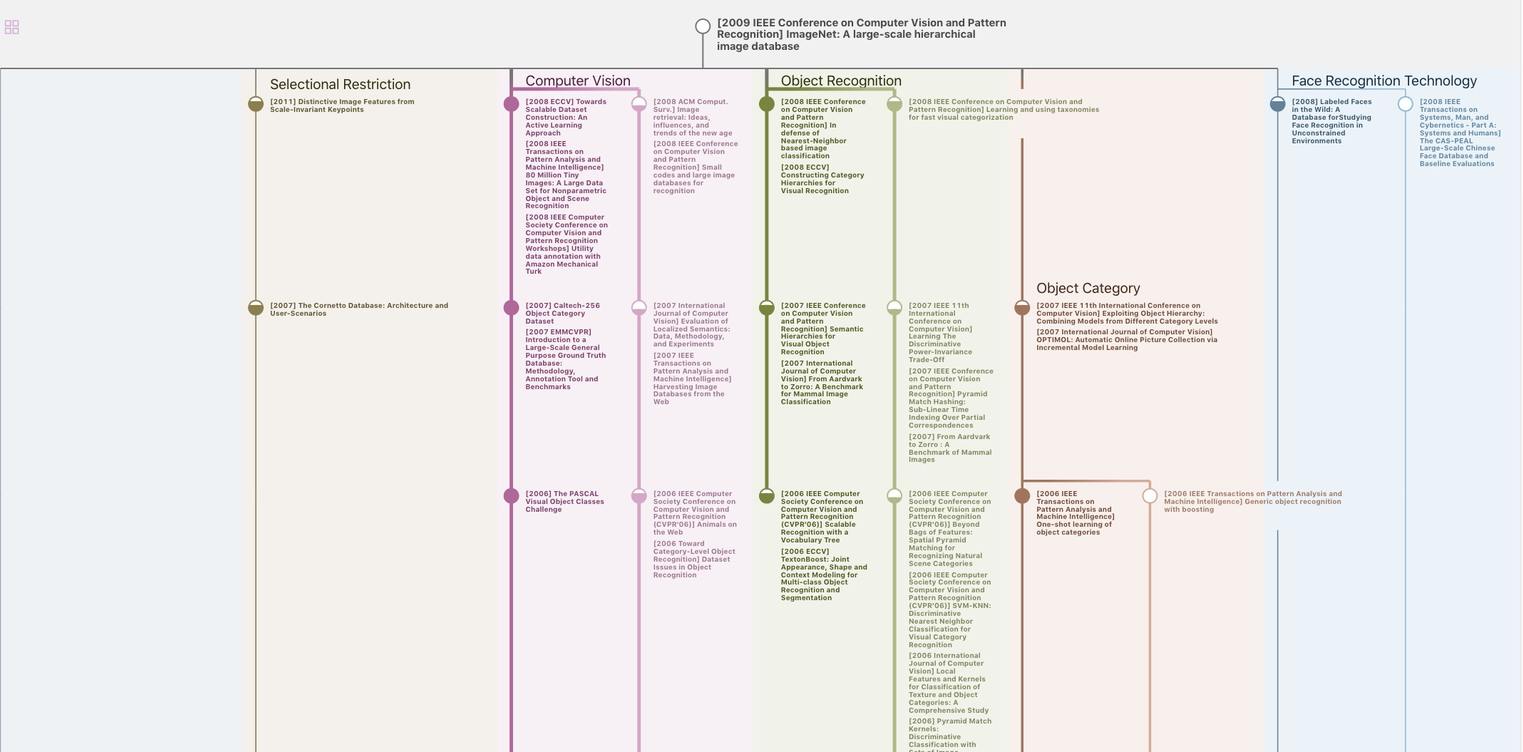
生成溯源树,研究论文发展脉络
Chat Paper
正在生成论文摘要