Response best-subset selector for multivariate regression with high-dimensional response variables
BIOMETRIKA(2023)
摘要
This article investigates the statistical problem of response-variable selection with high-dimensional response variables and a diverging number of predictor variables with respect to the sample size in the framework of multivariate linear regression. A response best-subset selection model is proposed by introducing a 0-1 selection indicator for each response variable, and then a response best-subset selector is developed by introducing a separation parameter and a novel penalized least-squares function. The proposed procedure can perform response-variable selection and regression-coefficient estimation simultaneously, and the response best-subset selector has the property of model consistency under mild conditions for both fixed and diverging numbers of predictor variables. Also, consistency and asymptotic normality of regression-coefficient estimators are established for cases with a fixed dimension, and it is found that the Bonferroni test is a special response best-subset selector. Finite-sample simulations show that the response best-subset selector has strong advantages over existing competitors in terms of the Matthews correlation coefficient, a criterion that aims to balance accuracies for both true and false response variables. An analysis of real data demonstrates the effectiveness of the response best-subset selector in an application involving the identification of dosage-sensitive genes.
更多查看译文
关键词
High dimensionality, Mixed 0-1 integer optimization, Multivariate linear regression, Penalized least-squares function, Response best-subset selector, Response-variable selection
AI 理解论文
溯源树
样例
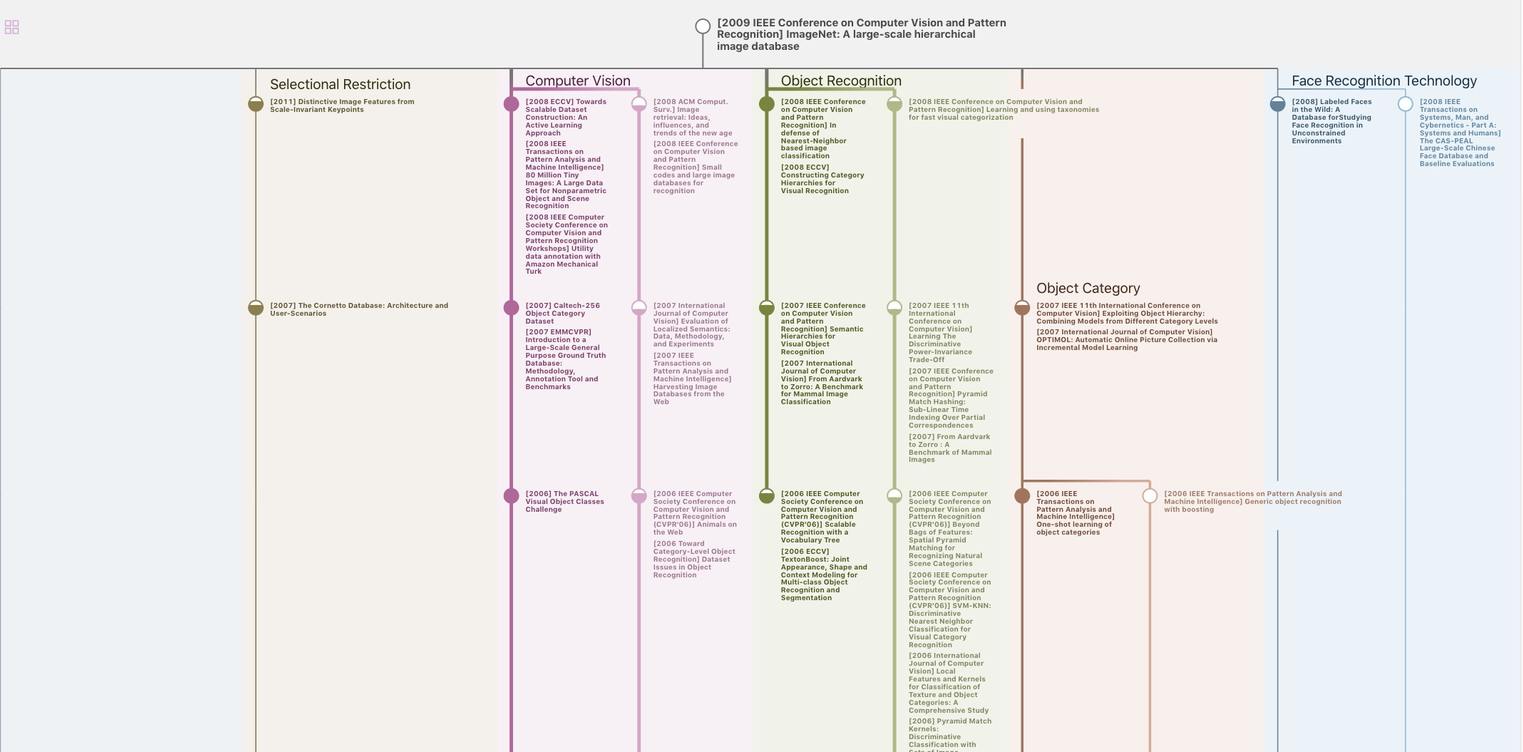
生成溯源树,研究论文发展脉络
Chat Paper
正在生成论文摘要