Multiview Latent Representation Learning with Feature Diversity for Clustering
MATHEMATICAL PROBLEMS IN ENGINEERING(2022)
摘要
To analyze and manage data, multiple features are extracted for robust and accurate description. Taking account of the complementary nature of multiview data, it is urgent to find a low-dimensional compact representation by leveraging multiple views and generating a better performance for clustering. In this paper, we present multiview latent representation learning with feature diversity for clustering (MvLRFD). To get rid of noise and maintain the information of each view, matrix factorization is adopted to get the latent representation. For fusing the diverse latent representations, we argue that the final representation is constructed by concatenating the latent representation of each view. With the help of the Hilbert-Schmidt Independence Criterion (HSIC), the diversity of features in the final representation is maximized to exploit the complementary information. A new coregularization strategy is introduced to maximize the distance between the similarity between data of the final representation and the latent one. The partition entropy regularization is adopted to control the uniformity level of the value of the similarity matrix and the weight of each view. To verify the availability of MvLRFD compared to state-of-the-art algorithms, several experiments are conducted on four real datasets.
更多查看译文
关键词
feature diversity,clustering,representation,learning
AI 理解论文
溯源树
样例
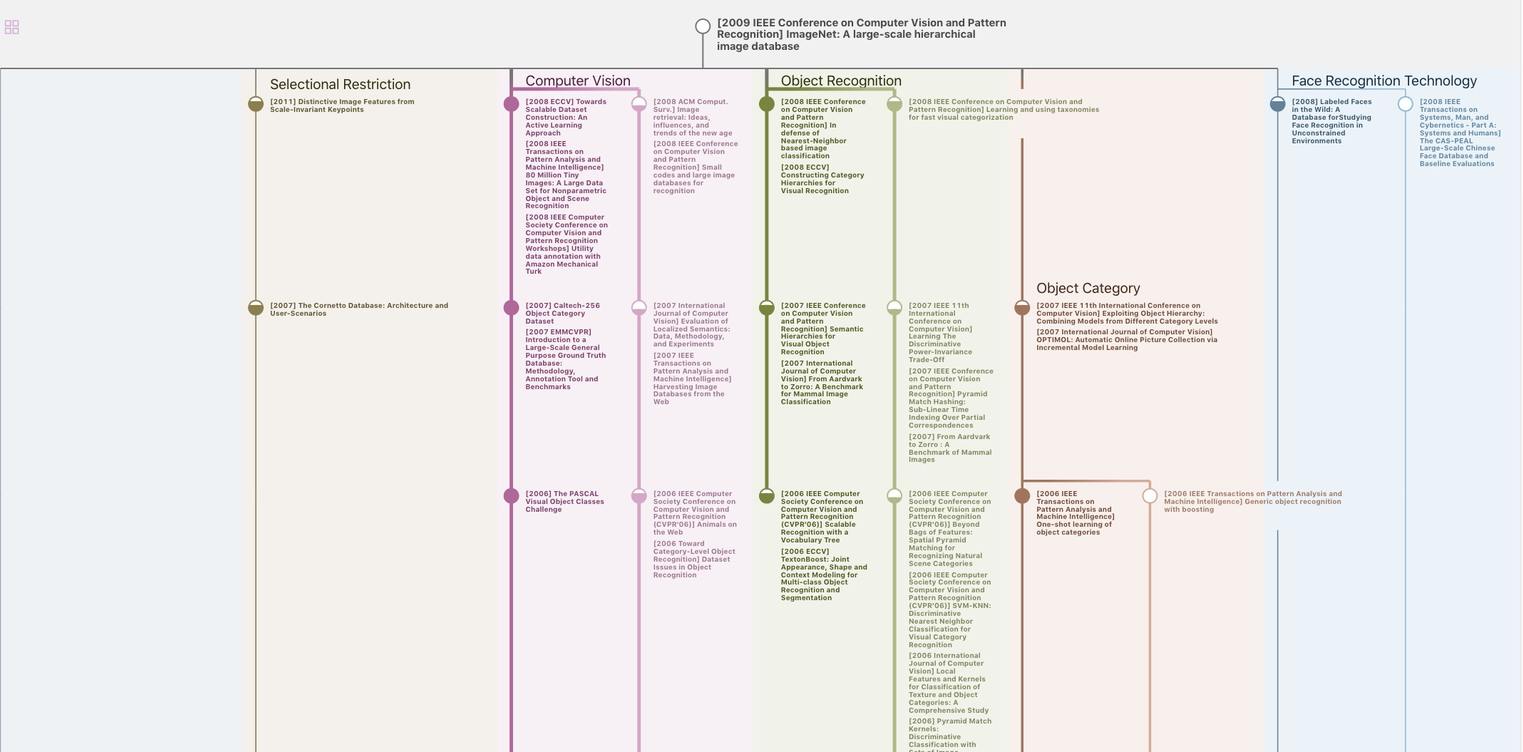
生成溯源树,研究论文发展脉络
Chat Paper
正在生成论文摘要