Identification of Similar Electrical Loads in Smart Homes with 100% Accuracy Provided by a Convolutional Neural Network with Minimum Parameters
Journal of Control Automation and Electrical Systems(2022)
摘要
The identification of electrical/electronic loads is one of the Smart Grids (SGs) relevant problems. An accuracy of 100 $$\%$$ can be achieved in SGs with non-similar loads. However, to the best of the authors knowledge, this level of accuracy was not described in the literature, considering SGs with similar loads. Therefore, the development of an intelligent classification system of similar electrical/electronic loads is described in this paper, aiming at the achievement of 100% accuracy with minimum parameters of the neural classifiers. An experimental platform containing an arrangement with four high similar loads was implemented. In such arrangement, all possible combinations are realized, that is, from all loads turned on until all turned off, resulting in non-similar and very similar loads, as found in real scenarios. The electrical signals demanded by the connected load arrangements are acquired and submitted to two different neural networks. The first neural network is an Extreme Learning Machine (ELM) classifier, where a Particle Swarm Optimization (PSO) technique is applied to select the best ELM model, whereas the second network is a Convolutional Neural Network (CNN) classifier. The ELM-PSO system is based on pre-processed data because it uses information based on the spectrum amplitude of the demand signals (features), whereas the CNN system is based on the use of the raw data, thus requiring a greater processing capacity when compared to the ELM-PSO. The simulation results show that the proposed CNN based system provides success rates considerably higher than the proposed ELM-PSO system, i.e., $$100\%$$ of accuracy with 381, 512 network parameters against $$85\%$$ with 14, 989 parameters of the ELM-PSO-based system.
更多查看译文
关键词
Smart homes,Similar load,Convolutional Neural Networks,Extreme Learning Machines,Particle Swarm Optimization
AI 理解论文
溯源树
样例
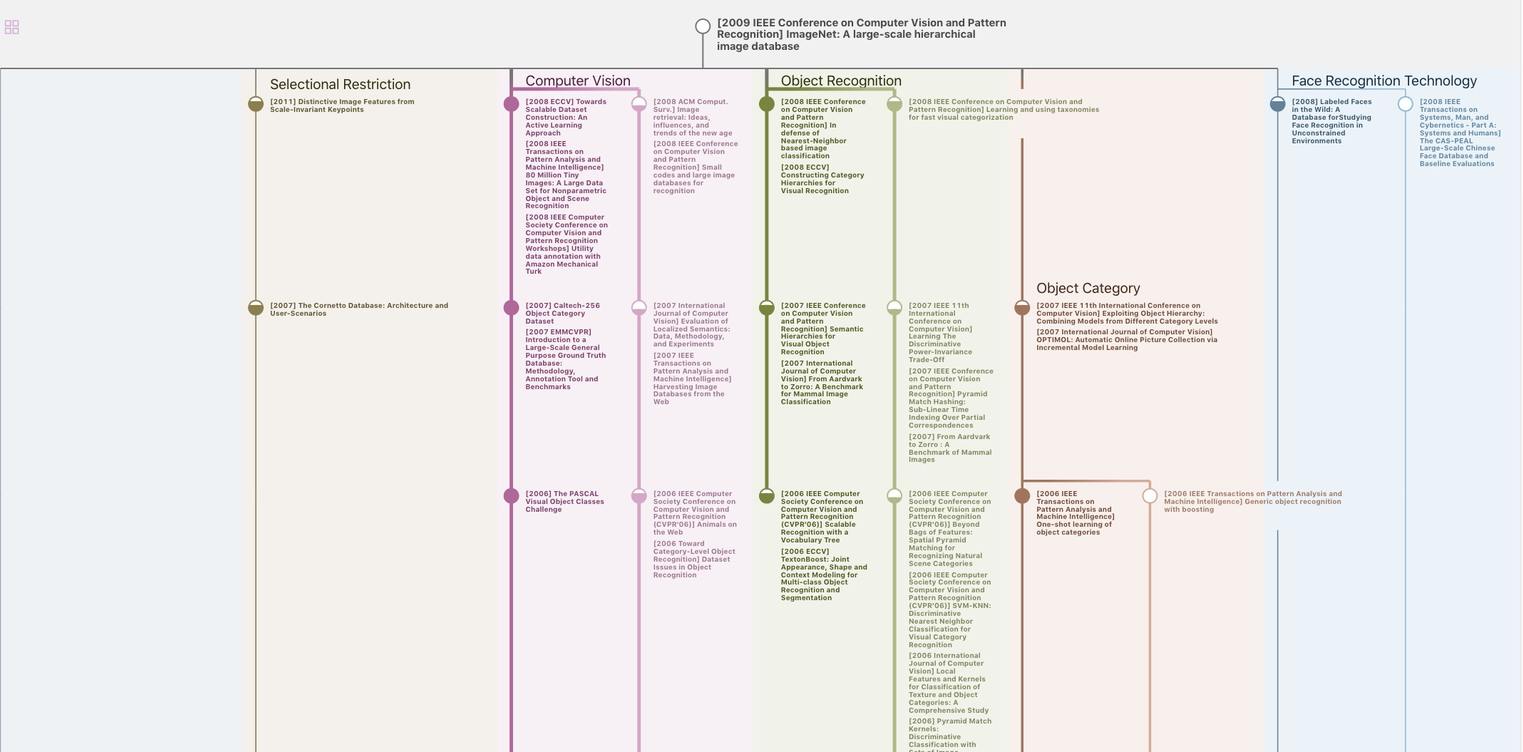
生成溯源树,研究论文发展脉络
Chat Paper
正在生成论文摘要