SRMANet: Toward an Interpretable Neural Network with Multi-Attention Mechanism for Gearbox Fault Diagnosis
APPLIED SCIENCES-BASEL(2022)
摘要
Deep neural network (DNN), with the capacity for feature inference and nonlinear mapping, has demonstrated its effectiveness in end-to-end fault diagnosis. However, the intermediate learning process of the DNN architecture is invisible, making it an uninterpretable black-box model. In this paper, a stacked residual multi-attention network (SRMANet) is proposed as a means of feature extraction of vibration signals, and visualizing the model training process, designing Squeeze-excitation residual (SE-Res) blocks to obtain additive features with minimal redundancy and sparsity. This study recommends the use of the attention fusion unit to ensure the interpretability of the model and ultimately to obtain representative features. By feeding the output gradient of the attention layer back to the original signal, the key feature components in the time domain signal can be effectively captured. Finally, the interpretability, identification accuracy and adaptability of the model under different operating conditions are verified on 12 different fault tasks in the planetary gearbox.
更多查看译文
关键词
machine fault diagnosis,universal feature extractor,convolutional neural network,attention fusion,interpretability,planetary gearbox
AI 理解论文
溯源树
样例
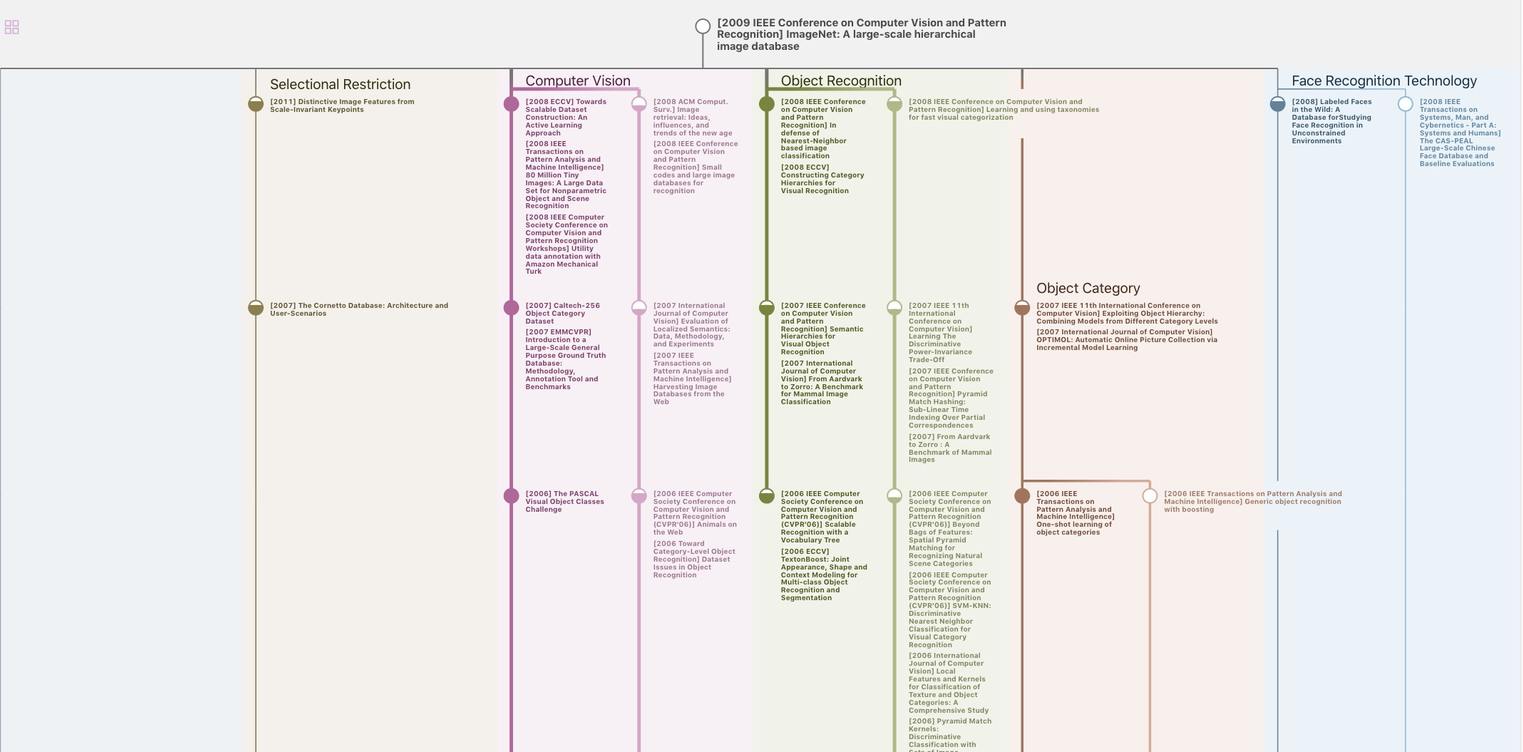
生成溯源树,研究论文发展脉络
Chat Paper
正在生成论文摘要