3M-CDNet-V2: An Efficient Medium-Weight Neural Network for Remote Sensing Image Change Detection
IEEE ACCESS(2022)
摘要
Remote sensing-based change detection (CD) is a critical technique of detecting land surface changes in earth observation. Inspired by recent success of a lightweight CD network- 3M-CDNet, we implemented 9 meaningful modifications to it, for example, the incorporation of MHSA (Multi-Head Self-Attention). This elaborately designed model is termed as 3M-CDNet-V2. Its effectiveness and advantages were demonstrated on three engineering CD datasets, and experimental results indicated that: (1) relative to other state-of-the-art algorithms, the proposed model obtained very competitive or slight better performance on both visual comparison and quantitative metrics evaluation. (2) By applying a novel transfer learning strategy, 3M-CDNet-V2 could perform well on the small dataset. (3) The incorporation of MHSA brings a substantial accuracy improvement while moderately increasing the computational complexity. (4) The late fusion framework and deep supervision contribute most to the performance gain of 3M-CDNet-V2, while the introduction of low-level features to the classifier by skip connection could guide the model to focus on detailed spatial information such as small changes, narrow shaped objects, or accurate boundaries. We hope that our 3M-CDNet-V2 model helps in improving the understanding of network architecture design for CD.
更多查看译文
关键词
Feature extraction, Semantics, Transformers, Remote sensing, Computer architecture, Computational modeling, Neural networks, Change detection algorithms, Neural network, remote sensing, medium weight, change detection
AI 理解论文
溯源树
样例
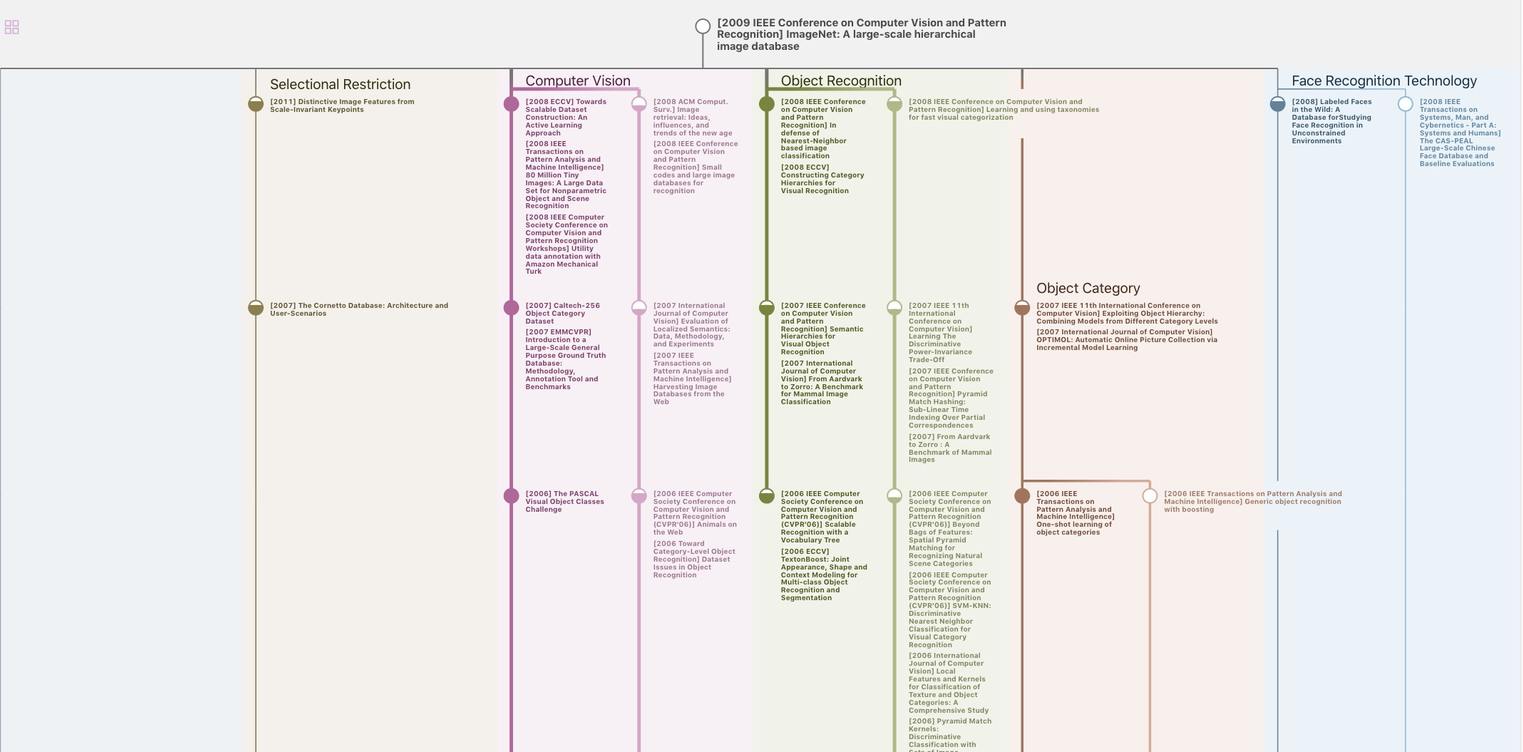
生成溯源树,研究论文发展脉络
Chat Paper
正在生成论文摘要