Predicting classifier performance using distributional separation measures
ARTIFICIAL INTELLIGENCE AND MACHINE LEARNING FOR MULTI-DOMAIN OPERATIONS APPLICATIONS IV(2022)
Abstract
In real world applications, machine learning classifiers are only as good as their performance on real world data. In practice, this data comes without truth labels - that is why you need a classifier. In this paper we consider the scenario where we have a trained classifier, and a set of unlabeled test data that we wish to run through it. If the unlabeled data is not within the distribution of the classifier's training data, we should not expect the classifier to work well on the test set. We explore the use of the Henze-Penrose divergence, a measure of separation between two multivariate distributions, as a way to predict performance of a classifier on a dataset and to detect distributional shift, and we find that by computing Henze-Penrose scores between the training and test sets first in the input space, and then in the feature space of the classifier, we can get an indication that the test data is out of distribution and that classification accuracy will be unreliable.
MoreTranslated text
Key words
classifier, neural network, robustness, performance prediction
AI Read Science
Must-Reading Tree
Example
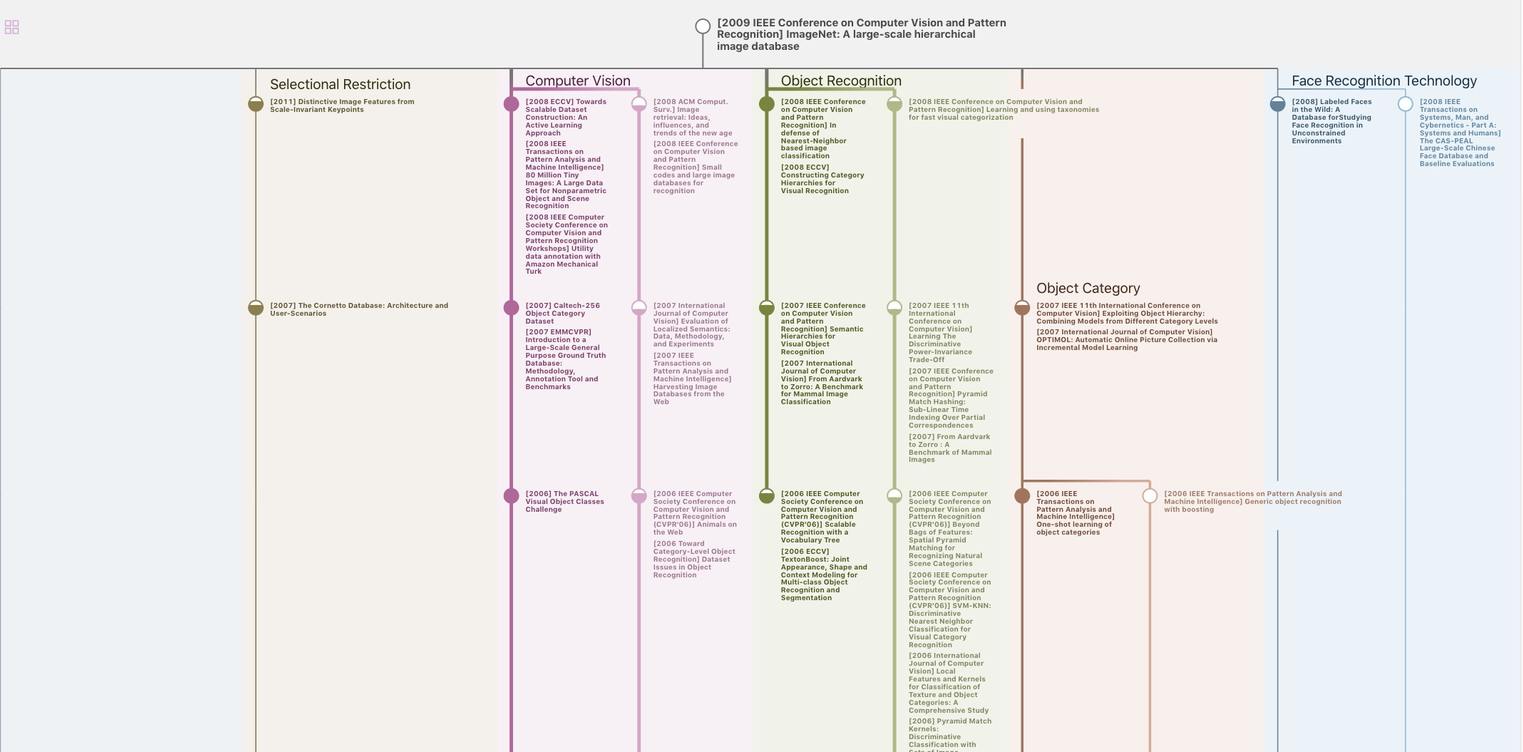
Generate MRT to find the research sequence of this paper
Chat Paper
Summary is being generated by the instructions you defined