Transformer-based Entity Typing in Knowledge Graphs
emnlp 2022(2022)
摘要
We investigate the knowledge graph entity typing task which aims at inferring plausible entity types. In this paper, we propose a novel Transformer-based Entity Typing (TET) approach, effectively encoding the content of neighbors of an entity. More precisely, TET is composed of three different mechanisms: a local transformer allowing to infer missing types of an entity by independently encoding the information provided by each of its neighbors; a global transformer aggregating the information of all neighbors of an entity into a single long sequence to reason about more complex entity types; and a context transformer integrating neighbors content based on their contribution to the type inference through information exchange between neighbor pairs. Furthermore, TET uses information about class membership of types to semantically strengthen the representation of an entity. Experiments on two real-world datasets demonstrate the superior performance of TET compared to the state-of-the-art.
更多查看译文
关键词
entity typing,knowledge,graphs,transformer-based
AI 理解论文
溯源树
样例
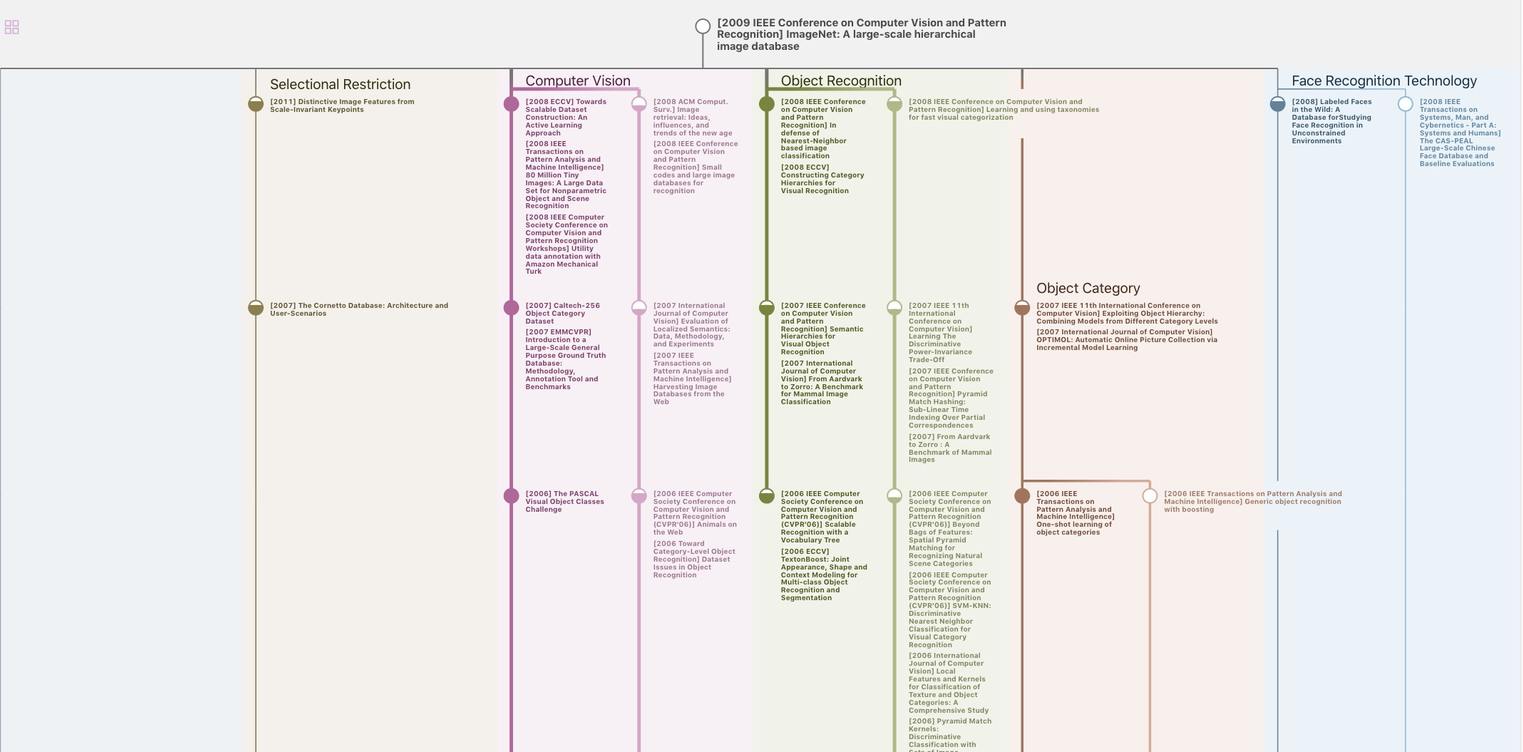
生成溯源树,研究论文发展脉络
Chat Paper
正在生成论文摘要