PAC-Bayesian Learning of Optimization Algorithms
arXiv (Cornell University)(2023)
摘要
We apply the PAC-Bayes theory to the setting of learning-to-optimize. To the best of our knowledge, we present the first framework to learn optimization algorithms with provable generalization guarantees (PAC-bounds) and explicit trade-off between a high probability of convergence and a high convergence speed. Even in the limit case, where convergence is guaranteed, our learned optimization algorithms provably outperform related algorithms based on a (deterministic) worst-case analysis. Our results rely on PAC-Bayes bounds for general, unbounded loss-functions based on exponential families. By generalizing existing ideas, we reformulate the learning procedure into a one-dimensional minimization problem and study the possibility to find a global minimum, which enables the algorithmic realization of the learning procedure. As a proof-of-concept, we learn hyperparameters of standard optimization algorithms to empirically underline our theory.
更多查看译文
关键词
optimization,algorithms,learning,pac-bayesian
AI 理解论文
溯源树
样例
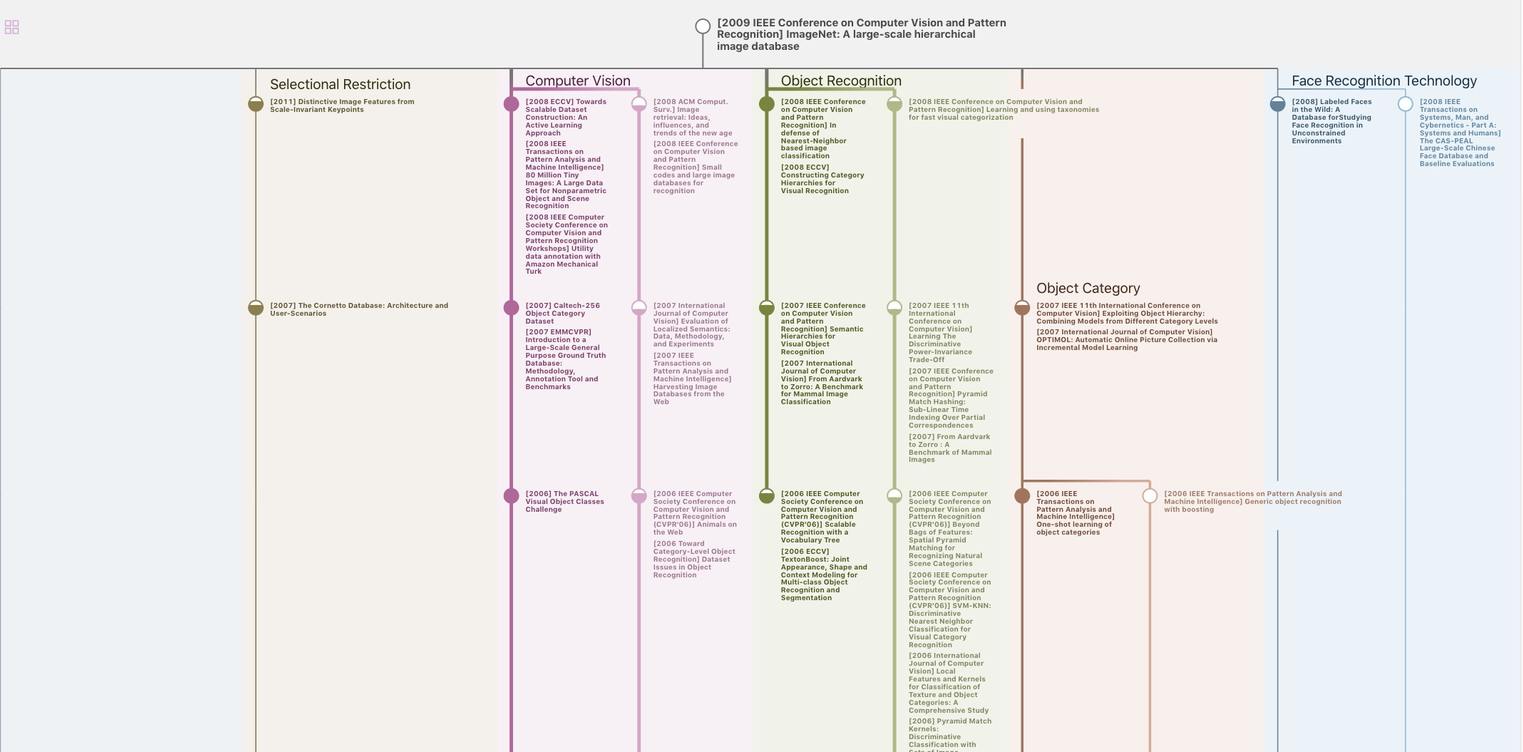
生成溯源树,研究论文发展脉络
Chat Paper
正在生成论文摘要