Federated Learning via Unmanned Aerial Vehicle
arXiv (Cornell University)(2022)
Abstract
To enable communication-efficient federated learning (FL), this paper studies an unmanned aerial vehicle (UAV)-enabled FL system, where the UAV coordinates distributed ground devices for a shared model training. Specifically, by exploiting the UAV's high altitude and mobility, the UAV can proactively establish short-distance line-of-sight links with devices and prevent any device from being a communication straggler. Thus, the model aggregation process can be accelerated while the cumulative model loss caused by device scheduling can be reduced, resulting in a decreased completion time. We first present the convergence analysis of FL without the assumption of convexity, demonstrating the effect of device scheduling on the global gradients. Based on the derived convergence bound, we further formulate the completion time minimization problem by jointly optimizing device scheduling, UAV trajectory, and time allocation. This problem explicitly incorporates the devices' energy budgets, dynamic channel conditions, and convergence accuracy of FL constraints. Despite the non-convexity of the formulated problem, we exploit its structure to decompose it into two sub-problems and further derive the optimal solutions via the Lagrange dual ascent method. Simulation results show that the proposed design significantly improves the tradeoff between completion time and prediction accuracy in practical FL settings compared to existing benchmarks.
MoreTranslated text
Key words
Federated leaning,UAV communications,completion time minimization,device scheduling,UAV trajectory design
AI Read Science
Must-Reading Tree
Example
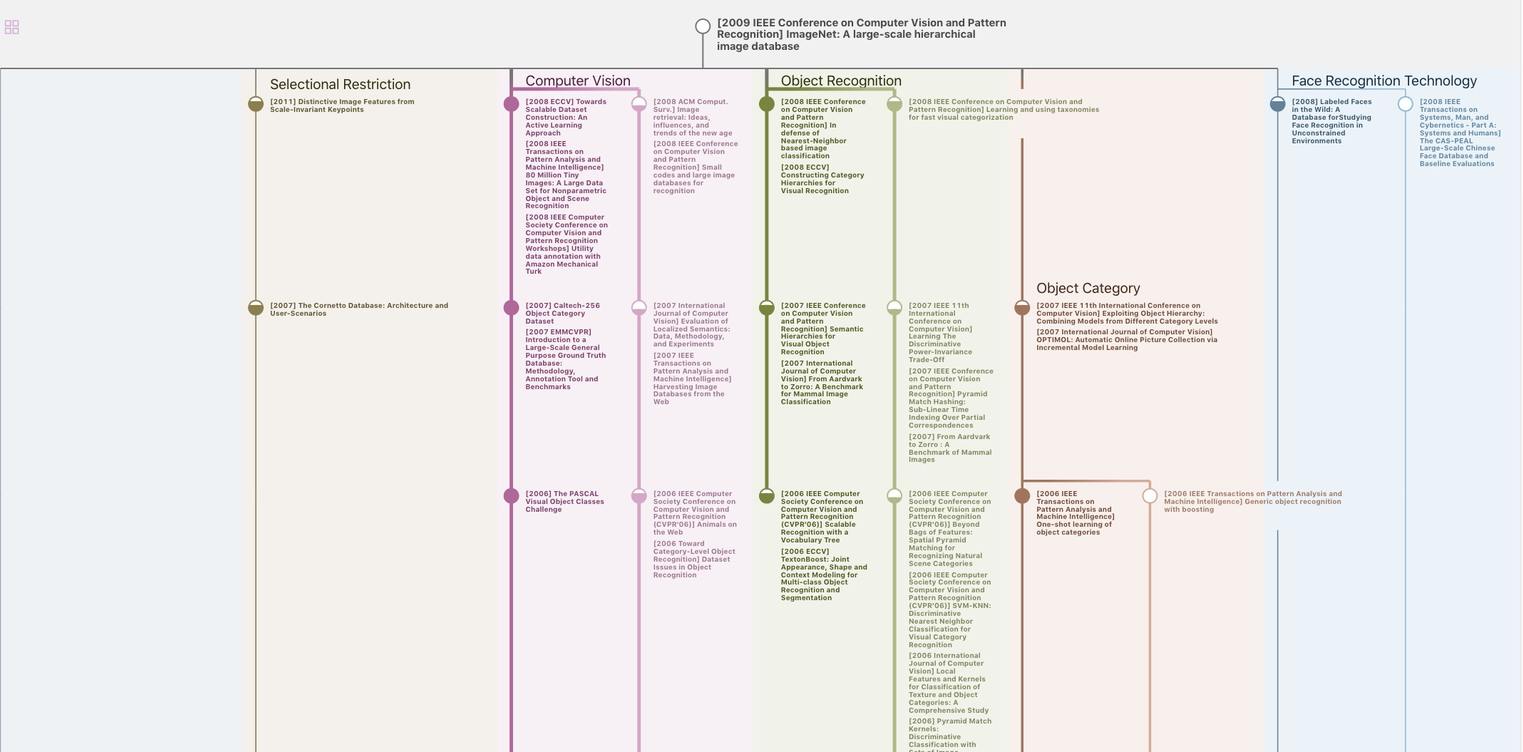
Generate MRT to find the research sequence of this paper
Chat Paper
Summary is being generated by the instructions you defined